Masked Imitation Learning: Discovering Environment-Invariant Modalities in Multimodal Demonstrations
2023 IEEE/RSJ INTERNATIONAL CONFERENCE ON INTELLIGENT ROBOTS AND SYSTEMS, IROS(2023)
Abstract
Multimodal demonstrations provide robots with an abundance of information to make sense of the world. However, such abundance may not always lead to good performance when it comes to learning sensorimotor control policies from human demonstrations. Extraneous data modalities can lead to state over-specification, where the state contains modalities that are not only useless for decision-making but also can change data distribution across environments. State over-specification leads to issues such as the learned policy not generalizing outside of the training data distribution. In this work, we propose Masked Imitation Learning (MIL) to address state over-specification by selectively using informative modalities. Specifically, we design a masked policy network with a binary mask to block certain modalities. We develop a bi-level optimization algorithm that learns this mask to accurately filter over-specified modalities. We demonstrate empirically that MIL outperforms baseline algorithms in simulated domains including MuJoCo and a robot arm environment using the Robomimic dataset, and effectively recovers the environment-invariant modalities on a multimodal dataset collected on a real robot. Our project website presents supplemental details and videos of our results at: https://tinyurl.com/masked-il
MoreTranslated text
Key words
Robot Learning,Reinforcement Learning,Multimodal Fusion
AI Read Science
Must-Reading Tree
Example
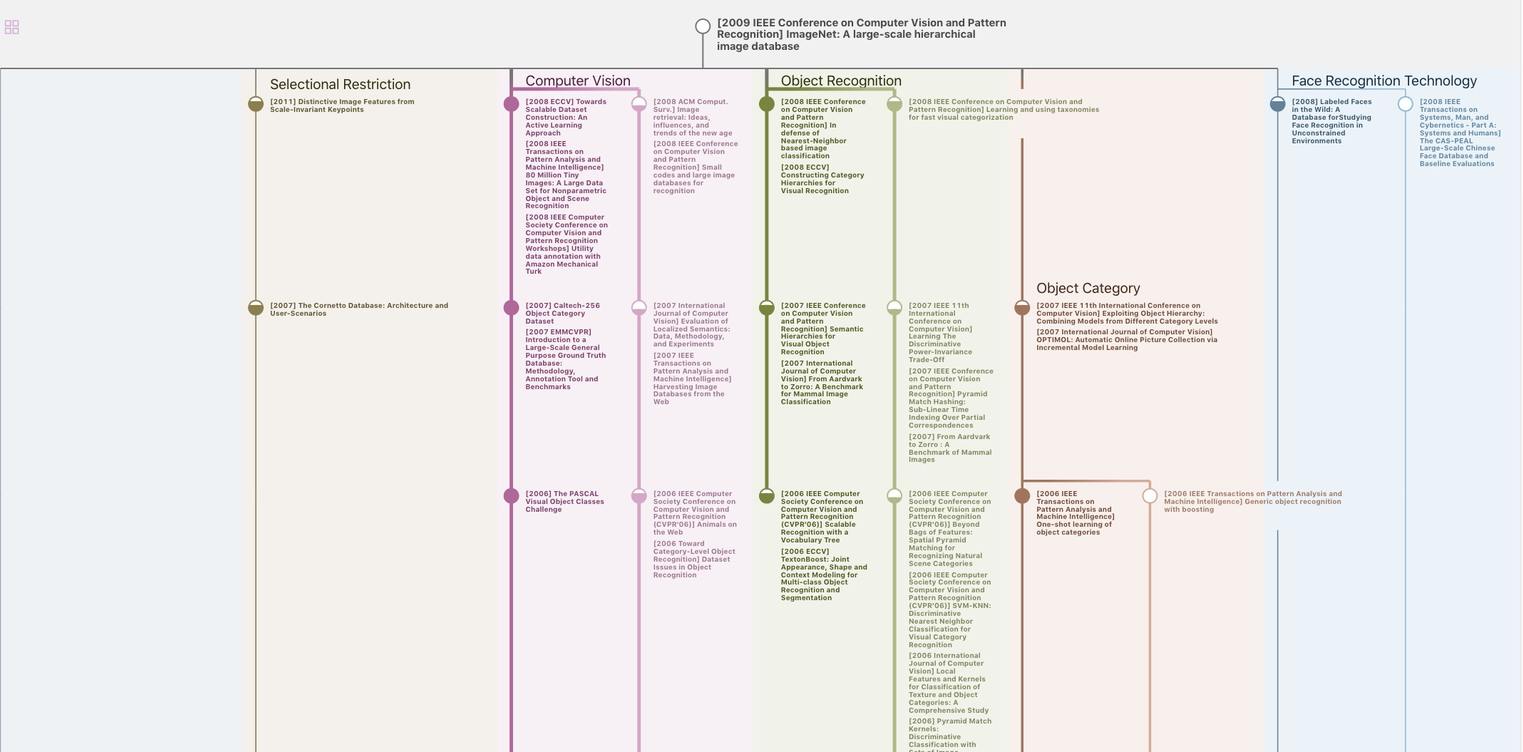
Generate MRT to find the research sequence of this paper
Chat Paper
Summary is being generated by the instructions you defined