Tiered Pruning for Efficient Differentialble Inference-Aware Neural Architecture Search
arxiv(2022)
摘要
We propose three novel pruning techniques to improve the cost and results of inference-aware Differentiable Neural Architecture Search (DNAS). First, we introduce , a stochastic bi-path building block for DNAS, which can search over inner hidden dimensions with memory and compute complexity. Second, we present an algorithm for pruning blocks within a stochastic layer of the SuperNet during the search. Third, we describe a novel technique for pruning unnecessary stochastic layers during the search. The optimized models resulting from the search are called PruNet and establishes a new state-of-the-art Pareto frontier for NVIDIA V100 in terms of inference latency for ImageNet Top-1 image classification accuracy. PruNet as a backbone also outperforms GPUNet and EfficientNet on the COCO object detection task on inference latency relative to mean Average Precision (mAP).
更多查看译文
关键词
nas,dnas,neural architecture search,differentiable neural architecture search,state-of-the-art,imagenet,classification,gpunet,efficientnet,pruning,inference-aware,computer vision,object detection
AI 理解论文
溯源树
样例
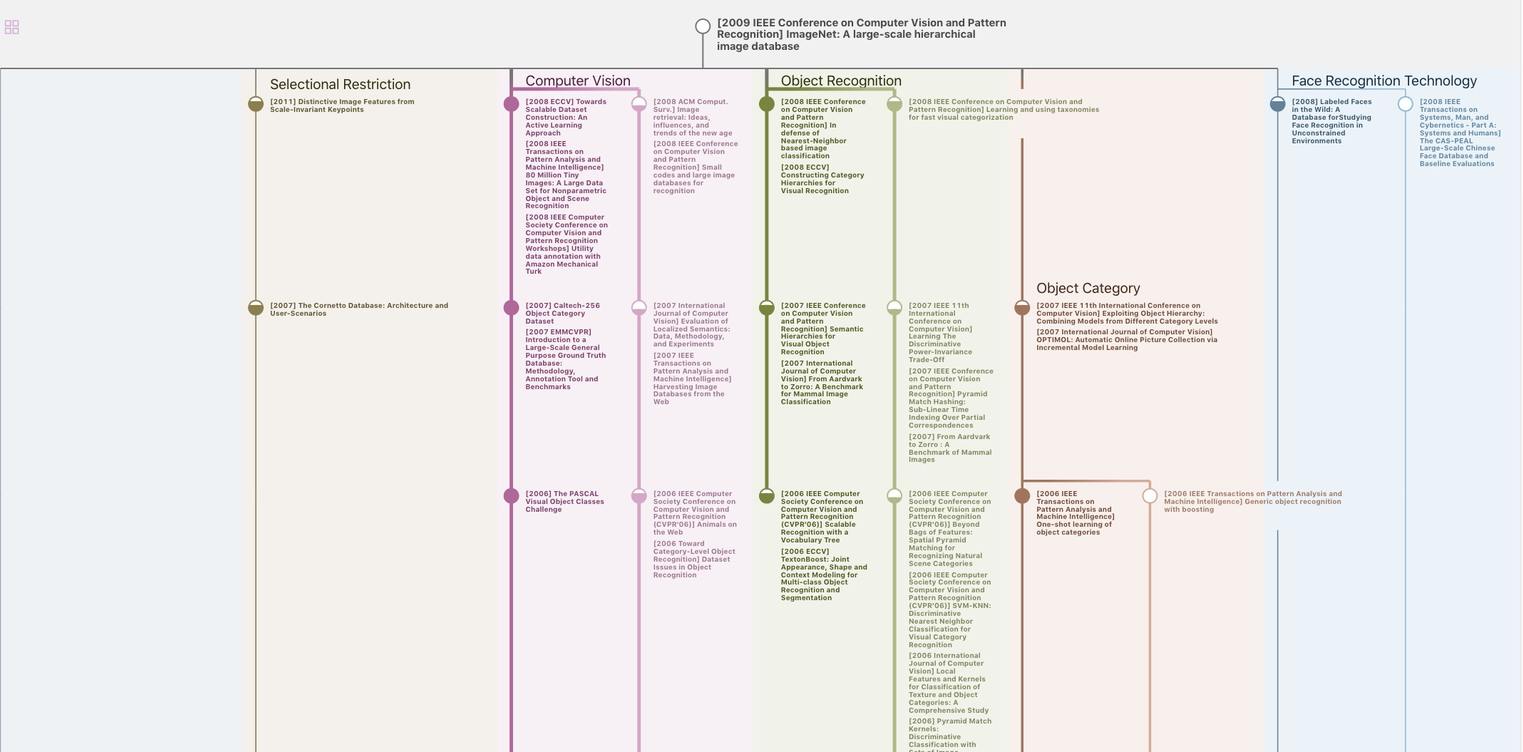
生成溯源树,研究论文发展脉络
Chat Paper
正在生成论文摘要