NARF22: Neural Articulated Radiance Fields for Configuration-Aware Rendering
2022 IEEE/RSJ International Conference on Intelligent Robots and Systems (IROS)(2022)
Abstract
Articulated objects pose a unique challenge for robotic perception and manipulation. Their increased number of degrees-of-freedom makes tasks such as localization computationally difficult, while also making the process of realworld dataset collection unscalable. With the aim of addressing these scalability issues, we propose Neural Articulated Radiance Fields (NARF22), a pipeline which uses a fully-differentiable, configuration-parameterized Neural Radiance Field (NeRF) as a means of providing high quality renderings of articulated objects. NARF22 requires no explicit knowledge of the object structure at inference time. We propose a two-stage partsbased training mechanism which allows the object rendering models to generalize well across the configuration space even if the underlying training data has as few as one configuration represented. We demonstrate the efficacy of NARF22 by training configurable renderers on a real-world articulated tool dataset collected via a Fetch mobile manipulation robot. We show the applicability of the model to gradient-based inference methods through a configuration estimation and 6 degree-of-freedom pose refinement task.
MoreTranslated text
Key words
Neural Radiance Fields,Shape Representation,Robot Learning,3D Object Recognition,Surface Parameterization
AI Read Science
Must-Reading Tree
Example
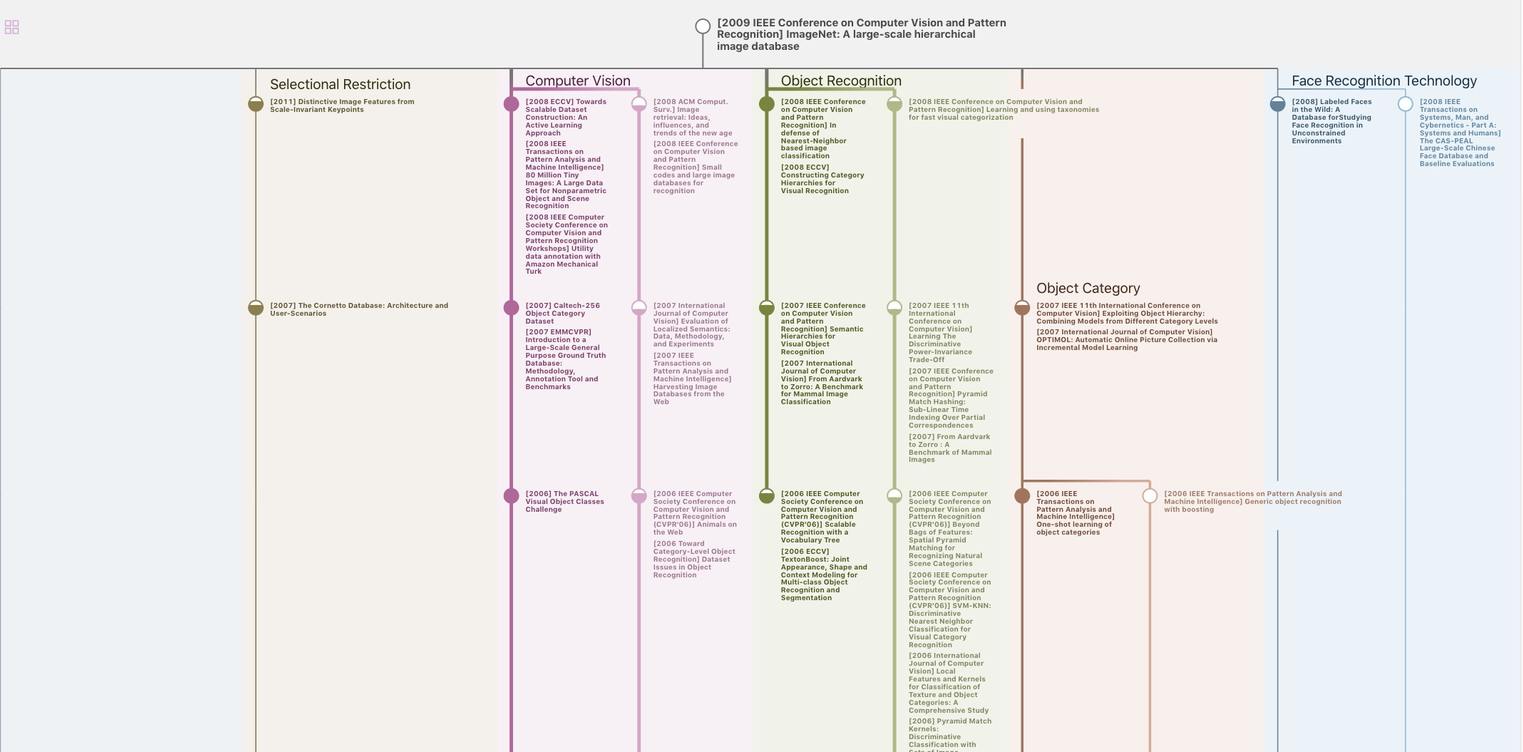
Generate MRT to find the research sequence of this paper
Chat Paper
Summary is being generated by the instructions you defined