Data-Efficiency with a Single GPU: An Exploration of Transfer Methods for Small Language Models
CoRR(2022)
Abstract
Multi-task learning (MTL), instruction tuning, and prompting have recently been shown to improve the generalizability of large language models to new tasks. However, the benefits of such methods are less well-documented in smaller language models, with some studies finding contradictory results. In this work, we explore and isolate the effects of (i) model size, (ii) general purpose MTL, (iii) in-domain MTL, (iv) instruction tuning, and (v) few-shot fine-tuning for models with fewer than 500 million parameters. Our experiments in the zero-shot setting demonstrate that models gain 31% relative improvement, on average, from general purpose MTL, with an additional 37.6% relative gain from in-domain MTL. Contradictory to prior works on large models, we find that instruction tuning provides a modest 2% performance improvement for small models.
MoreTranslated text
Key words
single gpu,transfer methods
AI Read Science
Must-Reading Tree
Example
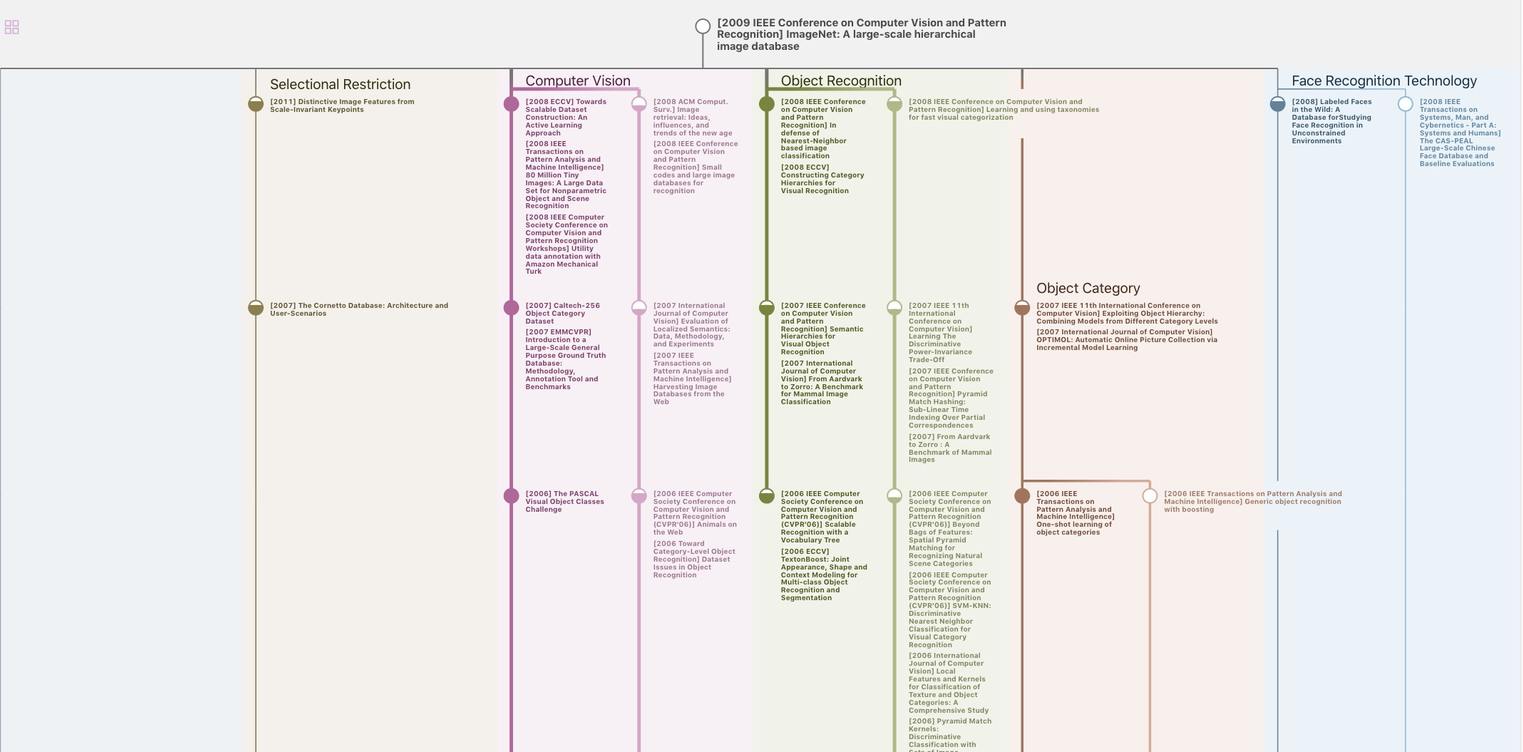
Generate MRT to find the research sequence of this paper
Chat Paper
Summary is being generated by the instructions you defined