Multiple Temporal Pooling Mechanisms for Weakly Supervised Temporal Action Localization
ACM Transactions on Multimedia Computing, Communications, and Applications(2022)
摘要
Recent action localization works learn in a weakly supervised manner to avoid the expensive cost of human labeling. Those works are mostly based on the Multiple Instance Learning framework, where temporal pooling is an indispensable part that usually relies on the guidance of snippet-level Class Activation Sequences (CAS). However, we observe that previous works only leverage a simple convolutional neural network for the generation of CAS, which ignores the weak discriminative foreground action segments and the background ones, and meanwhile, the relationship between different actions has not been considered. To solve this problem, we propose multiple temporal pooling mechanisms (MTP) for a more sufficient information utilization. Specifically, with the design of the Foreground Variance Branch, Dual Foreground Attention Branch and Hybrid Attention Fine-tuning Branch, MTP can leverage more effective information from different aspects and generate different CASs to guide the learning of temporal pooling. Moreover, different loss functions are designed for a better optimization of individual branches, aiming to effectively distinguish the action from the background. Our method shows excellent results on the THUMOS14 and ActivityNet1.2 datasets.
更多查看译文
关键词
Weakly supervised temporal action localization,multiple instance learning,temporal pooling
AI 理解论文
溯源树
样例
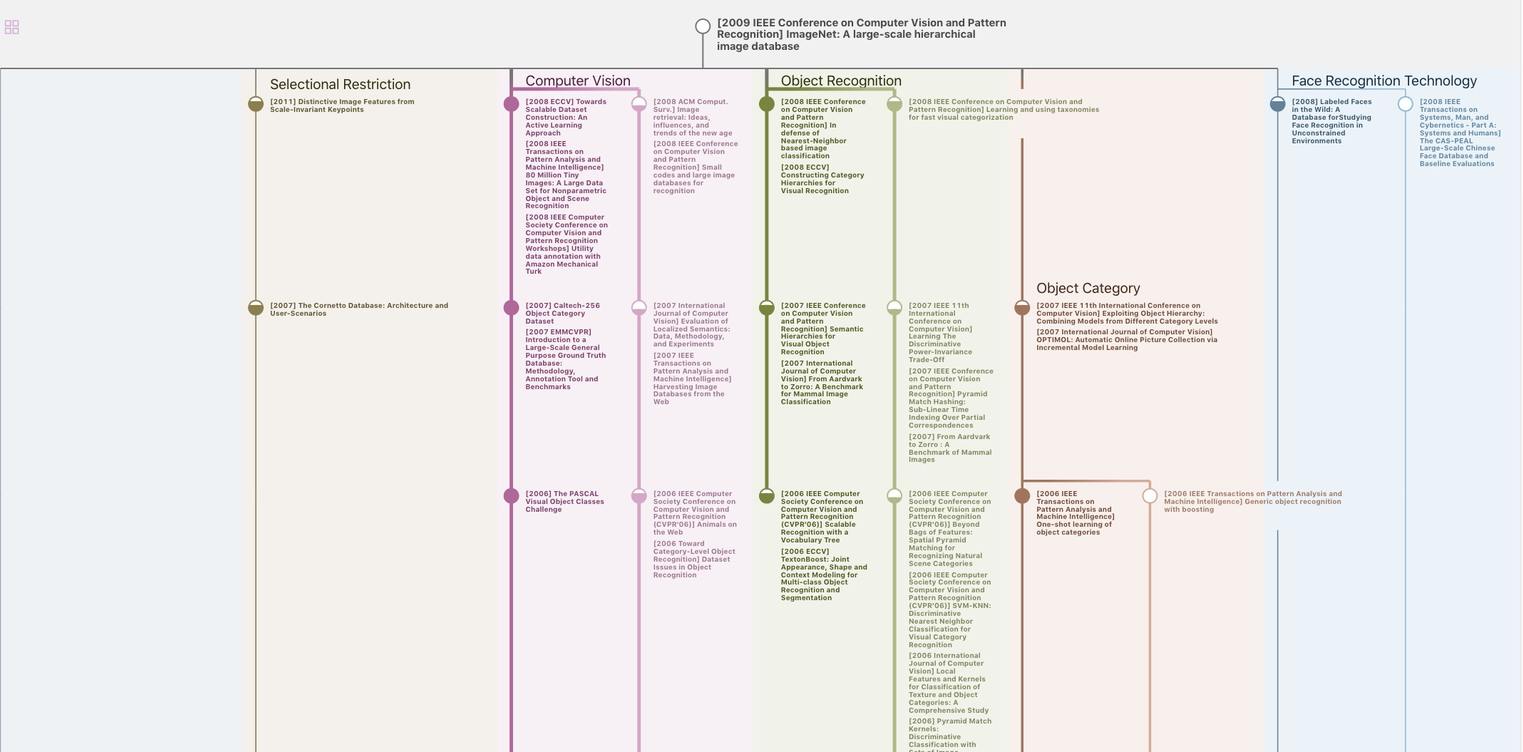
生成溯源树,研究论文发展脉络
Chat Paper
正在生成论文摘要