Graph Node Based Interpretability Guided Sample Selection for Active Learning
IEEE Transactions on Medical Imaging(2023)
摘要
While supervised learning techniques have demonstrated state-of-the-art performance in many medical image analysis tasks, the role of sample selection is important. Selecting the most informative samples contributes to the system attaining optimum performance with minimum labeled samples, which translates to fewer expert interventions and cost. Active Learning (AL) methods for informative sample selection are effective in boosting performance of computer aided diagnosis systems when limited labels are available. Conventional approaches to AL have mostly focused on the single label setting where a sample has only one disease label from the set of possible labels. These approaches do not perform optimally in the multi-label setting where a sample can have multiple disease labels (e.g. in chest X-ray images). In this paper we propose a novel sample selection approach based on graph analysis to identify informative samples in a multi-label setting. For every analyzed sample, each class label is denoted as a separate node of a graph. Building on findings from interpretability of deep learning models, edge interactions in this graph characterize similarity between corresponding interpretability saliency map model encodings. We explore different types of graph aggregation to identify informative samples for active learning. We apply our method to public chest X-ray and medical image datasets, and report improved results over state-of-the-art AL techniques in terms of model performance, learning rates, and robustness.
更多查看译文
关键词
Uncertainty,Measurement,Computational modeling,X-ray imaging,Entropy,Predictive models,Estimation,Interpretability,graphs multi-label,sample selection lung disease classification
AI 理解论文
溯源树
样例
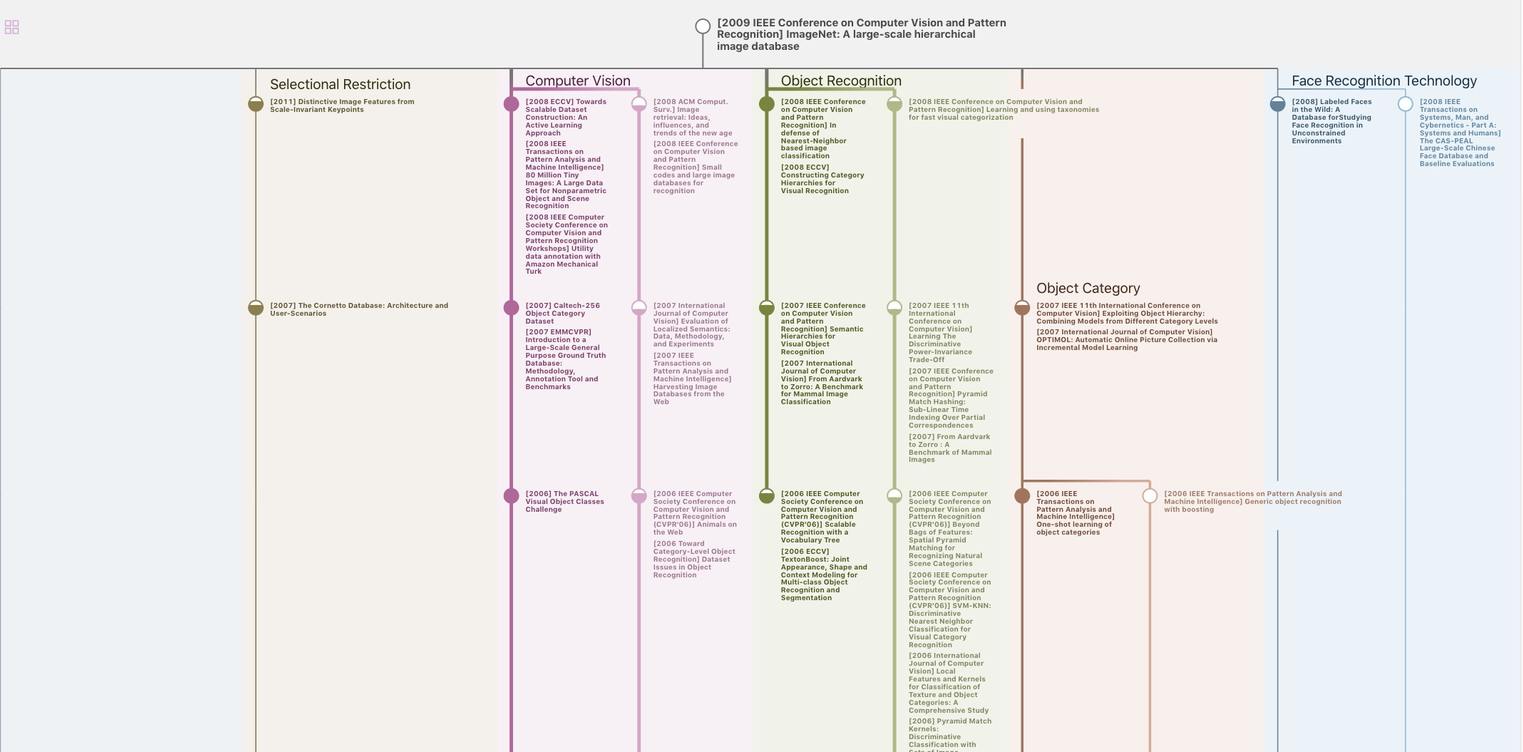
生成溯源树,研究论文发展脉络
Chat Paper
正在生成论文摘要