ReCoRo: Region-Controllable Robust Light Enhancement with User-Specified Imprecise Masks
International Multimedia Conference(2022)
摘要
ABSTRACTLow-light enhancement is an increasingly important function in image editing and visual creation. Most existing enhancing algorithms are trained to enlighten a given image in a globally homogeneous way, and (implicitly) to some predefined extent of brightness. They are neither capable of enhancing only local regions of interest ("where") while keeping the overall visual appearance plausible, nor producing outputs at a range of different illumination levels ("how much"). Those hurdles significantly limit the prospect of flexible, customizable, or even user-interactive low-light enhancement. To address these gaps, we propose Region-Controllable Robust Light Enhancement (ReCoRo), a novel framework that allows users to directly specify "where" and "how much" they want to enhance from an input low-light image; meanwhile, the model will learn to intelligently maintain the overall consistent visual appearance and plausible composition via a discriminator. Moreover, since in practical mobile APPs, such user specifications often come in imprecise forms (e.g., finger-drawn masks), we propose to bake in domain-specific data augmentations into training ReCoRo, so that the learned model can gain resilience to various roughly-supplied user masks. Up to our best knowledge, ReCoRo is the first of its kind that allows the user to localize the enlightenment region as well as to control the light intensity. Extensive experiments clearly demonstrate that ReCoRo outperforms state-of-the-art methods in terms of qualitative results, quantitative metrics, and versatile controllability. Project repository: https://bit.ly/ReCoRo-lowlight.
更多查看译文
AI 理解论文
溯源树
样例
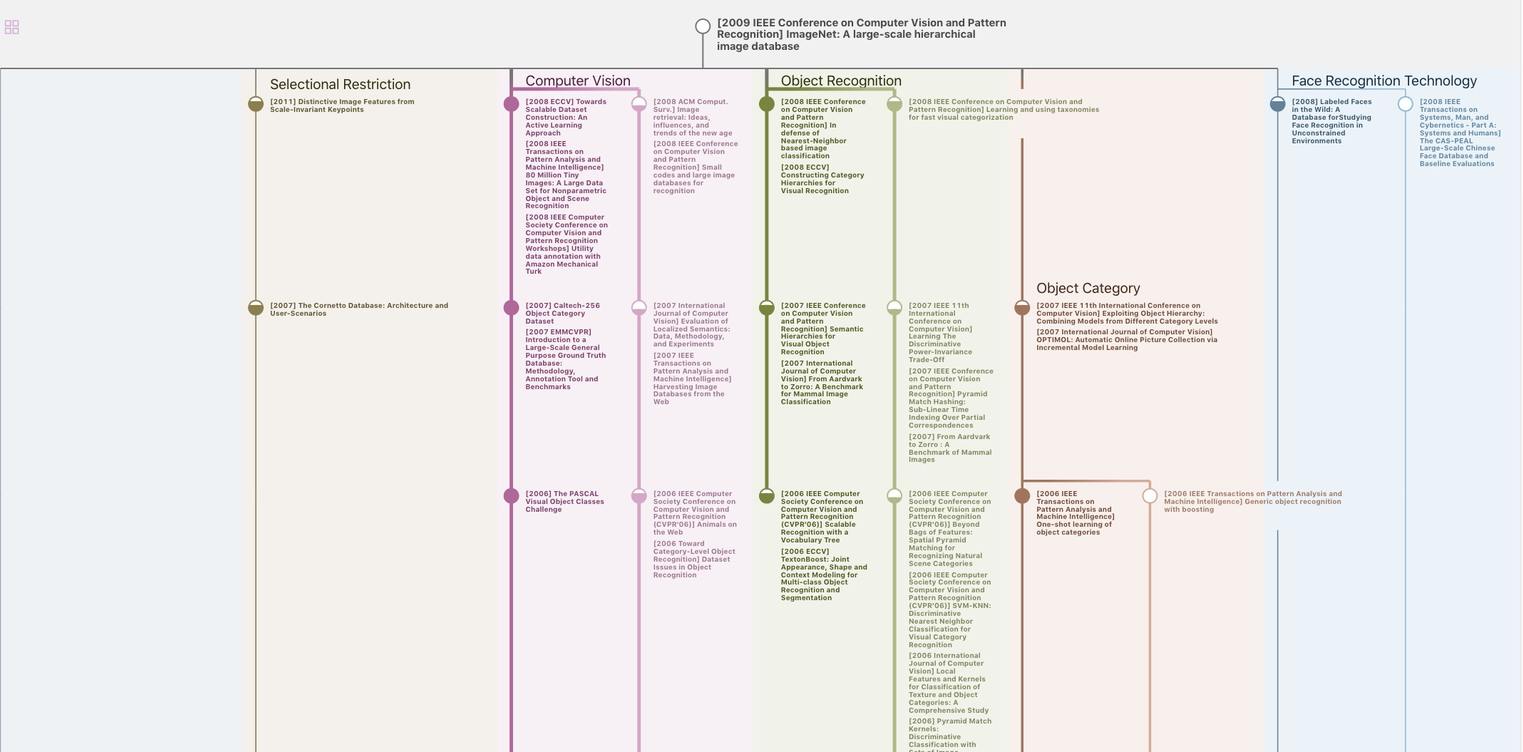
生成溯源树,研究论文发展脉络
Chat Paper
正在生成论文摘要