SoundDoA: Learn Sound Source Direction of Arrival and Semantics from Sound Raw Waveforms
Conference of the International Speech Communication Association (INTERSPEECH)(2022)
摘要
A fundamental task for an agent to understand an environment acoustically is to detect sound source location (like direction of arrival (DoA)) and semantic label. It is a challenging task: firstly, sound sources overlap in time, frequency and space; secondly, while semantics are largely conveyed through time-frequency energy (amplitude) contours, DoA is encoded in inter-channel phase difference; lastly, although the number of microphone sensors are sparse, recorded sound waveform is temporally dense due to the high sampling rates. Existing methods for predicting DoA mostly depend on pre-extracted 2D acoustic feature such as GCC-PHAT and Mel-spectrograms so as to benefit from the success of mature 2D image based deep neural networks. We instead propose a novel end-to-end trainable framework, named SoundDoA, that is capable of learning sound source DoA and semantics directly from sound raw waveforms. We first use a learnable front-end filter bank to dynamically encode sound source semantics and DoA relevant features into a compact representation. A backbone network consisting of two identical sub-networks with layerwise communication strategy is then proposed to further learn semantic label and DoA both separately and jointly. Finally, a permutation invariant multi-track head is added to regress DoA and classify semantic label. Extensive experimental results on DCASE 2020 sound event detection and localization dataset (SELD) demonstrate the superiority of SoundDoA, when comparing with other existing methods.
更多查看译文
关键词
DoA estimation, Learning from Raw Waveform
AI 理解论文
溯源树
样例
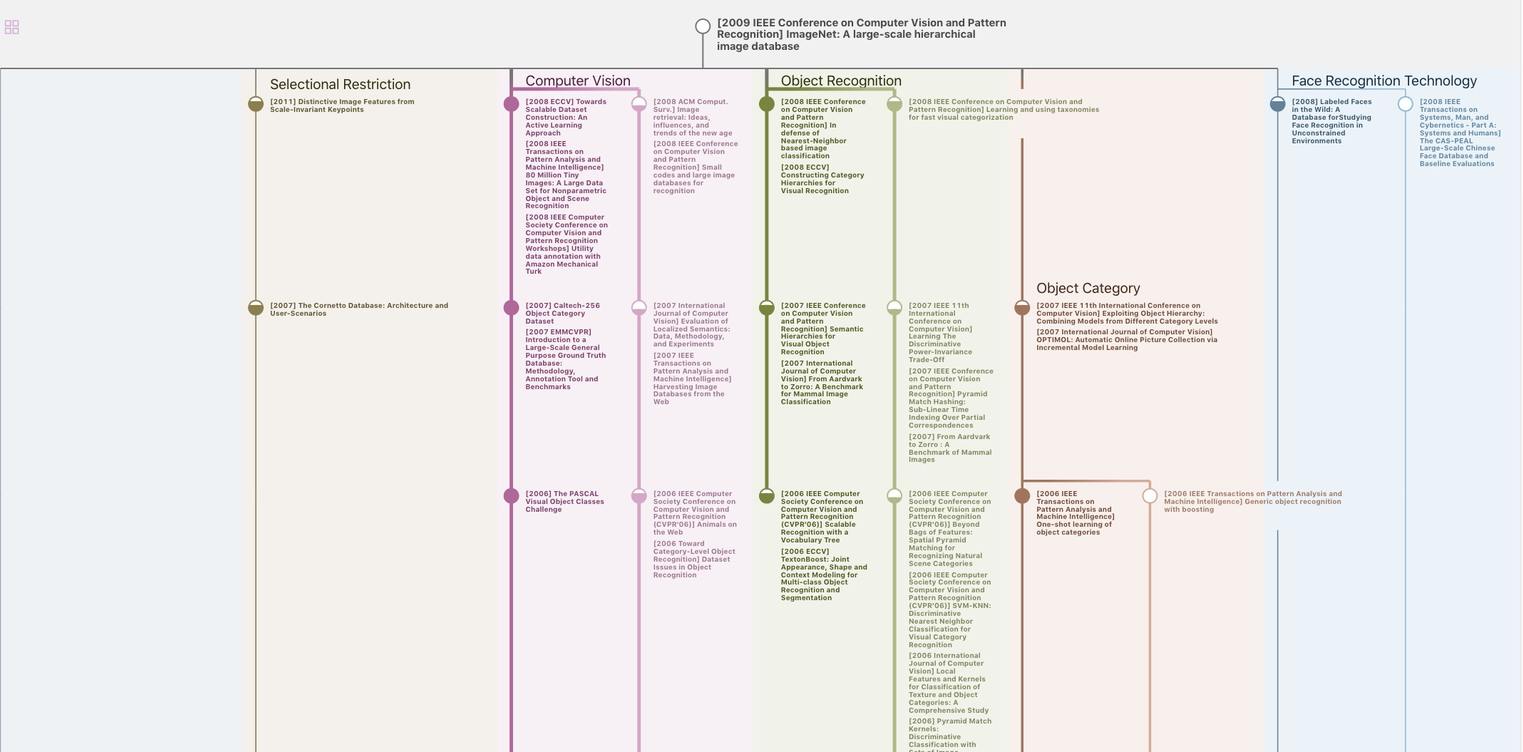
生成溯源树,研究论文发展脉络
Chat Paper
正在生成论文摘要