RMBench: Benchmarking Deep Reinforcement Learning for Robotic Manipulator Control.
2023 IEEE/RSJ INTERNATIONAL CONFERENCE ON INTELLIGENT ROBOTS AND SYSTEMS, IROS(2023)
摘要
Reinforcement learning is used to tackle complex tasks with high-dimensional sensory inputs. Over the past decade, a wide range of reinforcement learning algorithms have been developed, with recent progress benefiting from deep learning for raw sensory signal representation. This raises a natural question: how well do these algorithms perform across different robotic manipulation tasks? To objectively compare algorithms, benchmarks use performance metrics. Benchmarks use objective performance metrics to offer a scientific way to compare algorithms. In this paper, we introduce RMBench, the first benchmark for robotic manipulations with high-dimensional continuous action and state spaces. We implement and evaluate reinforcement learning algorithms that take observed pixels as inputs and report their average performance and learning curves to demonstrate their performance and training stability. Our study concludes that none of the evaluated algorithms can handle all tasks well, with soft Actor-Critic outperforming most algorithms in terms of average reward and stability, and an algorithm combined with data augmentation potentially facilitating learning policies. Our code is publicly available at https://github.com/xiangyanfei212/RMBench-2022.git, including all benchmark tasks and studied algorithms.
更多查看译文
AI 理解论文
溯源树
样例
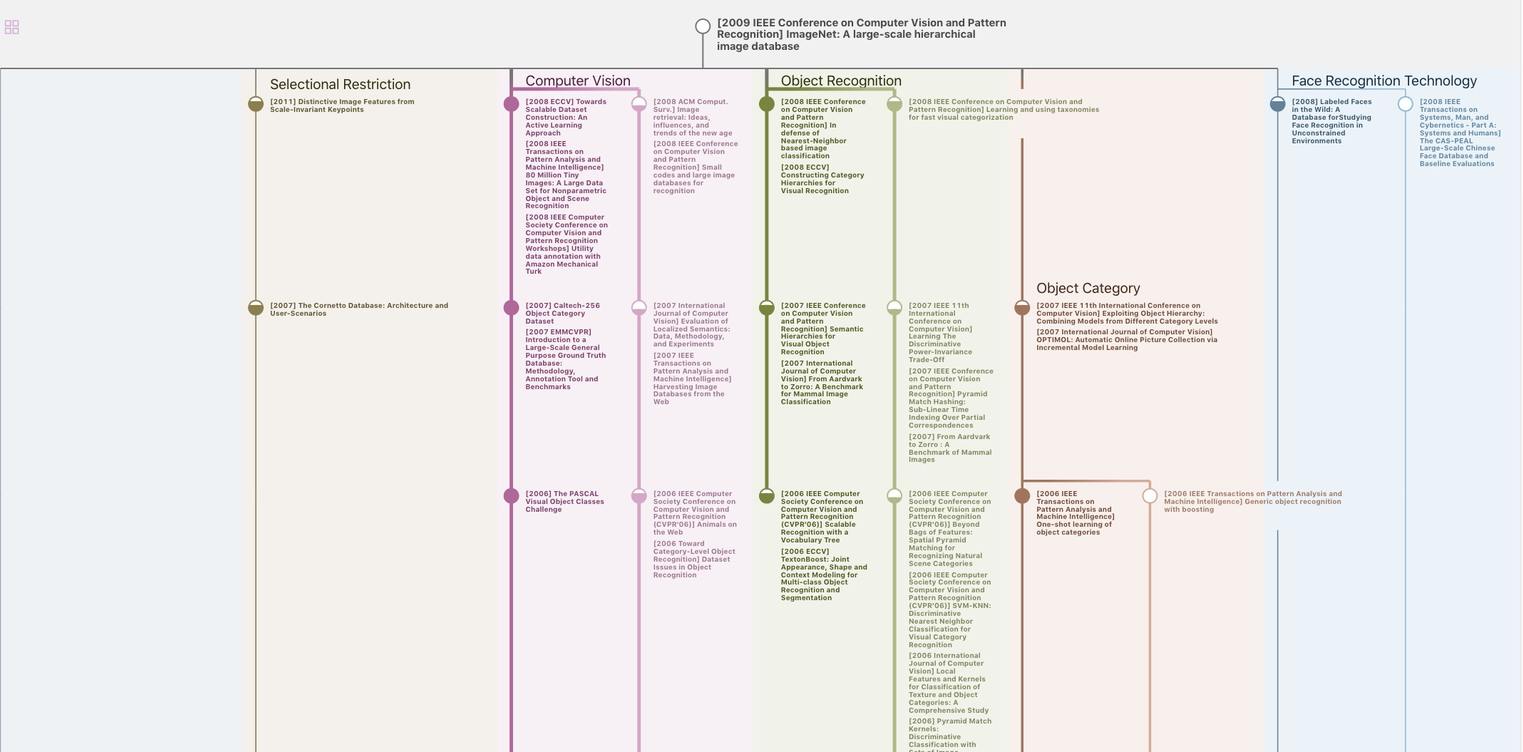
生成溯源树,研究论文发展脉络
Chat Paper
正在生成论文摘要