Geodesic Learning With Uniform Interpolation on Data Manifold
IEEE ACCESS(2022)
摘要
Recently with the development of deep learning on data representation and generation, how to sampling on a data manifold becomes a crucial problem for research. In this paper, we propose a method to learn a minimizing geodesic within a data manifold. Along the learned geodesic, our method is able to generate high-quality uniform interpolations with the shortest path between two given data samples. Specifically, we use an autoencoder network to map data samples into the latent space and perform interpolation in the latent space via an interpolation network. We add prior geometric information to regularize our autoencoder for a flat latent embedding. The Riemannian metric on the data manifold is induced by the canonical metric in the Euclidean space in which the data manifold is isometrically immersed. Based on this defined Riemannian metric, we introduce a constant-speed loss and a minimizing geodesic loss to regularize the interpolation network to generate uniform interpolations along the learned geodesic on the manifold. We provide a theoretical analysis of our model and use image interpolation as an example to demonstrate the effectiveness of our method.
更多查看译文
关键词
Interpolation,Manifolds,Measurement,Decoding,Manifold learning,Geometry,Trajectory,Learning systems,Geodesic learning,uniform interpolation,flat embedding,autoencoder,constant-speed
AI 理解论文
溯源树
样例
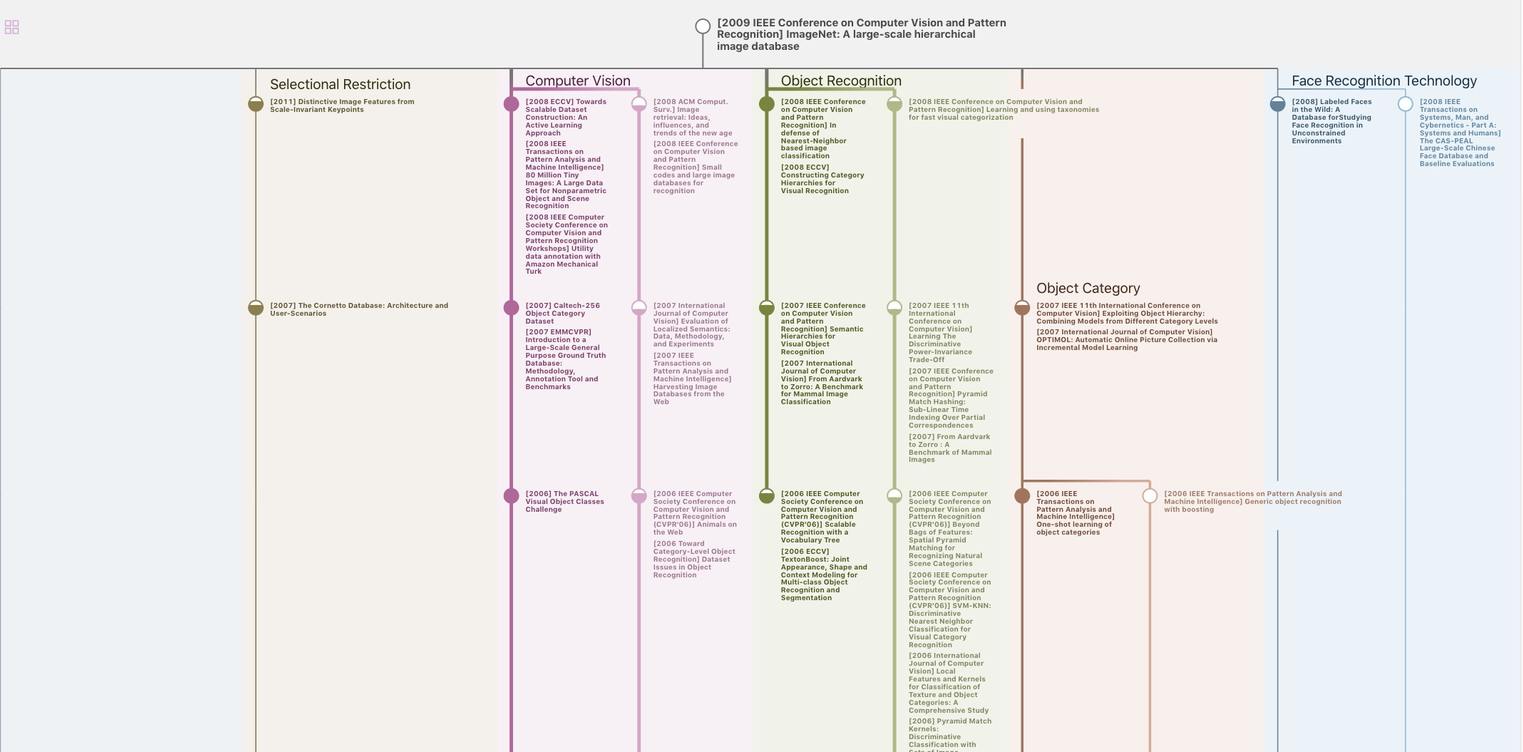
生成溯源树,研究论文发展脉络
Chat Paper
正在生成论文摘要