Prostate Cancer Risk Stratification in NRG Oncology Phase III Randomized Trials Using Multi-Modal Deep Learning with Digital Histopathology
International Journal of Radiation Oncology*Biology*Physics(2022)
摘要
Purpose/Objective(s)
Patients with intermediate- (int) and high-risk localized prostate cancer (PCa) are known to exhibit highly variable prognoses. There is a need for improved risk stratification tools for more accurate prognostication. We previously used clinical and digital histopathology data from five phase III PCa trials (NRG/RTOG 9202, 9408, 9413, 9910, and 0126) to develop and validate multi-modal artificial intelligence models (MMAI) that outperform NCCN in prediction of distant metastasis (DM) and other outcomes. In the five randomized trials, we demonstrated that the MMAI model could stratify patients into risk groups that more accurately reflect their prognosis compared to NCCN risk groups.Materials/Methods
The previously locked MMAI 5-yr metastasis model was applied across the 5,569 men, and deciles with similar DM rates were binned into 3 tiers: favorable (1–6th decile), moderate (7–9th) and unfavorable (10th) risk. Patients unable to be classified into NCCN risk groups were excluded. The Fine-Gray competing risk method was used to estimate the cumulative incidence of metastasis. The three-tier MMAI model was compared to standard prognostic factors such as PSA, Grade Group (GG), and three-tier NCCN.Results
The median follow-up for censored patients was 11.4 yrs. The median (interquartile range) PSA was 8.4 (5.8–12), 14 (8.8–25), and 32 (16–63) ng/mL for MMAI favorable, moderate, and unfavorable risks. Of the 3,829 patients with GG1/2, 71%, 25%, and 4% were stratified as MMAI favorable, moderate, and unfavorable risk respectively. Similarly, 63%, 30% and 7% for the 867 GG3 patients; and 7%, 50%, and 43% for the 716 GG4/5 patients. NCCN classified 584 (10%) patients as low-, 3,060 (55%) as int-, and 1,925 (35%) as high-risk, with estimated 5-yr DM rates (DM5, 95% confidence intervals [CI]) of 1% (0%–2%), 3% (3%–4%) and 10% (9%–12%) respectively. In contrast, the MMAI model grouped 3,342 (60%) patients as favorable, 1,671 (30%) as moderate, and 556 (10%) as unfavorable risk, with estimated DM5 rates of 1% (1–2%), 6% (5–7%), and 28% (25–32%). The estimated 10-yr DM rates (DM10, 95%CI) were 3% (1%–4%), 6% (5%–7%) and 17% (15%–18%) for NCCN low-, int-, and high-, respectively; and were 3% (3%–4%), 12% (10%–14%), and 37% (33%–41%) for MMAI risk groups. Within NCCN int-risk, MMAI identified 83% favorable risk patients with 4% DM10; and within NCCN high-risk, MMAI identified 13% favorable risk patients with only 4% DM10.Conclusion
The three-tier MMAI models integrating clinical and digital histopathology data demonstrated improved discrimination of DM risk compared to NCCN. The MMAI model identified 6-fold more patients with a lower risk of DM compared to NCCN. Conversely, patients in the MMAI unfavorable risk group had substantially greater DM risk than NCCN high-risk. Use of these MMAI models may improve prognostication, enabling more informed decision-making for patient care.查看译文
AI 理解论文
溯源树
样例
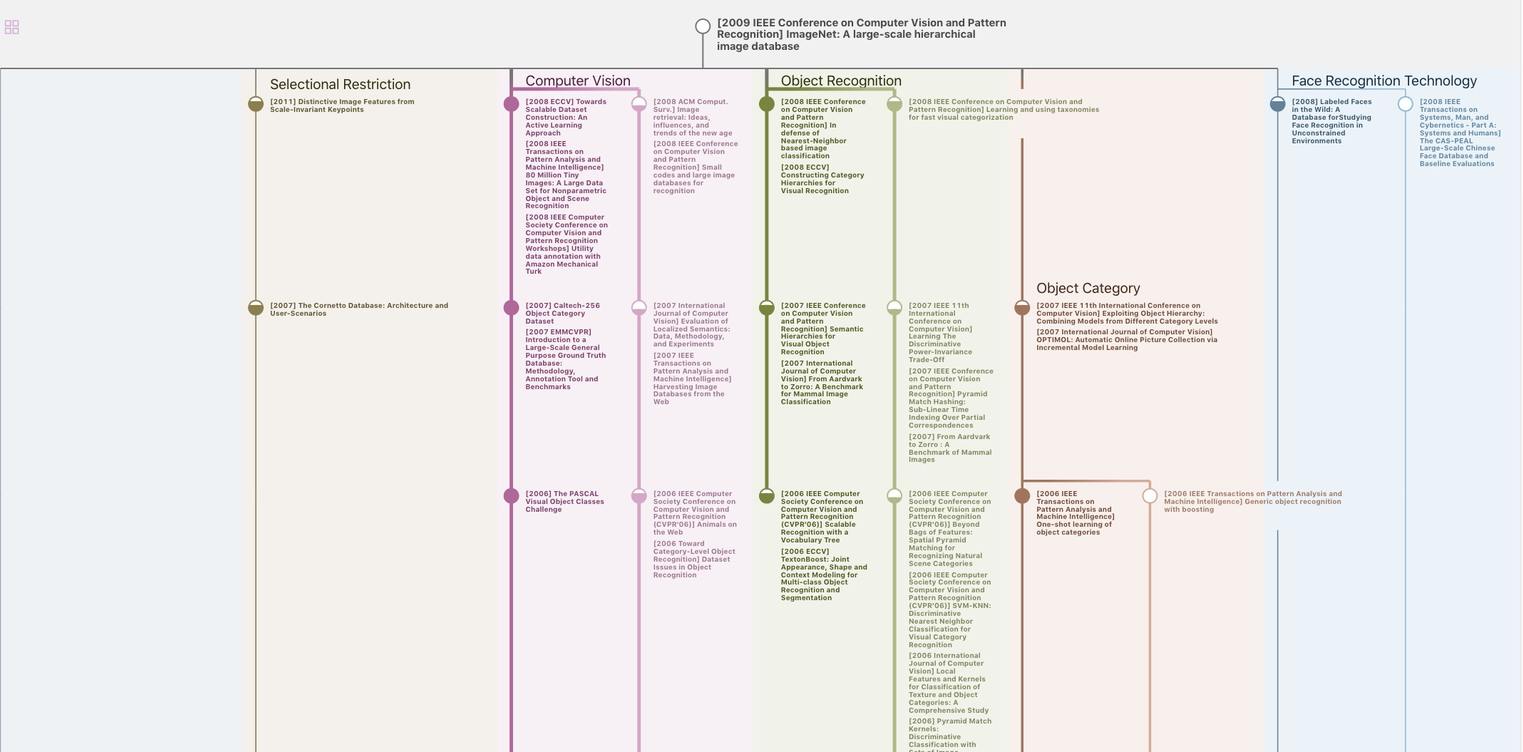
生成溯源树,研究论文发展脉络
Chat Paper
正在生成论文摘要