Machine-learning enabled prediction of successful sperm retrieval for microdissection testicular sperm extraction
Fertility and Sterility(2022)
摘要
Successful sperm retrieval (SSR) for microdissection testicular sperm extraction (mTESE) is reported in only 50% of patients. Therefore, identifying demographic and clinical factors associated with SSR is essential to improve preoperative decision-making. We utilized machine learning (ML) to develop predictive models of SSR for mTESE. We retrospectively reviewed patients who underwent mTESE between 2002-2021. Demographic and clinical information including age, BMI, race/ethnicity, urologic history, semen volume, testis size, and preoperative testosterone, follicle stimulating hormone (FSH), and luteinizing hormone (LH) were collected. Univariate logistic regression was used to identify factors associated with SSR. Covariates with p<0.30 were included as inputs to each of five supervised ML models: elastic net-regularized logistic regression, random forest, support vector machine, neural network, and eXtreme gradient boosting (XGBoost). The dataset was partitioned into training (80%) and testing (20%) sets, and hyperparameter optimization was conducted in a 5-fold cross-validated fashion with training data. Lastly, models were assessed for accuracy, discrimination via area under the receiver operating characteristic curve (AUROC), calibration, and Brier score on the hold-out test set. 217 patients undergoing mTESE were included, of whom 123 (56.7%) had SSR. The random forest algorithm achieved the best performance in the hold-out test set (86% accuracy, 0.94 [95% CI 0.81-0.98] AUROC, calibration slope 1.33, calibration intercept 0.02, Brier score 0.10). The most important predictive factors associated with SSR in the ML model were preoperative FSH, preoperative LH, age at the time of mTESE, and preoperative semen volume. Per univariate logistic regression, preoperative FSH (OR 0.95, 95% CI 0.93-0.97, p<0.001), LH (OR 0.91, 95% CI 0.86-0.96, p=0.001), and semen volume (OR 0.67, 95% CI 0.55-0.80, p<0.001) were significantly inversely associated with SSR. Preoperative demographic and clinical factors, namely FSH, LH, preoperative semen volume, and patient age at time of mTESE, were utilized in ML models to accurately predict SSR for mTESE. This approach may improve prognostication for patients with male factor infertility.
更多查看译文
关键词
microdissection testicular sperm extraction,successful sperm retrieval,machine-learning machine-learning
AI 理解论文
溯源树
样例
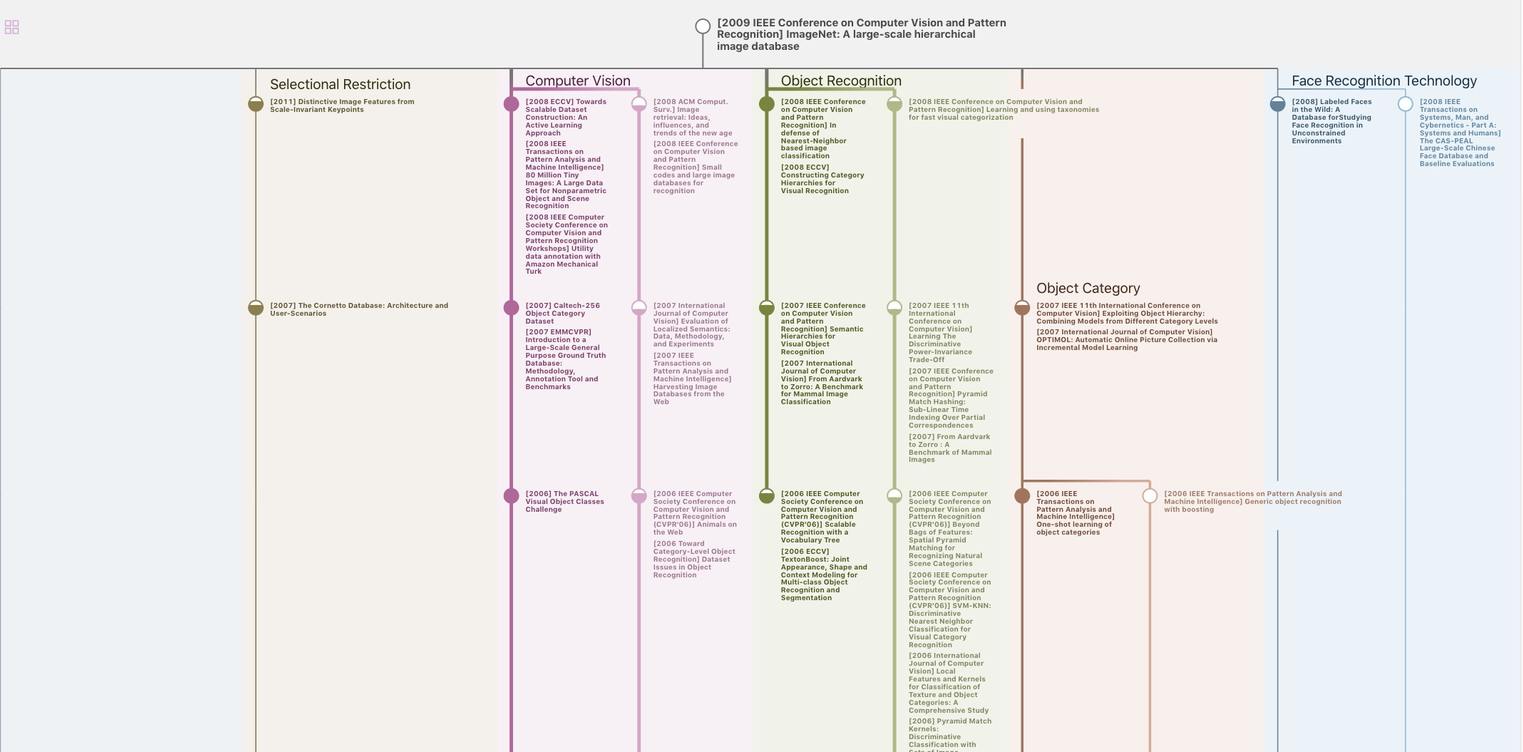
生成溯源树,研究论文发展脉络
Chat Paper
正在生成论文摘要