Arithmetic Sampling: Parallel Diverse Decoding for Large Language Models
arxiv(2022)
摘要
Decoding methods for large language models often trade-off between diversity of outputs and parallelism of computation. Methods such as beam search and Gumbel top-k sampling can guarantee a different output for each element of the beam, but are not easy to parallelize. Alternatively, methods such as temperature sampling and its modifications (top-k sampling, nucleus sampling, typical decoding, and others), are embarrassingly parallel, but have no guarantees about duplicate samples. We present a framework for sampling according to an arithmetic code book implicitly defined by a large language model, compatible with common sampling variations, with provable beam diversity under certain conditions, as well as being embarrassingly parallel and providing unbiased and consistent expectations from the original model. We demonstrate the effectiveness of our approach on WMT machine translation, showing substantially reduced variance when estimating expected BLEU score and up to 1 point increased BLEU in oracle experiments.
更多查看译文
关键词
parallel diverse decoding,sampling,language,models
AI 理解论文
溯源树
样例
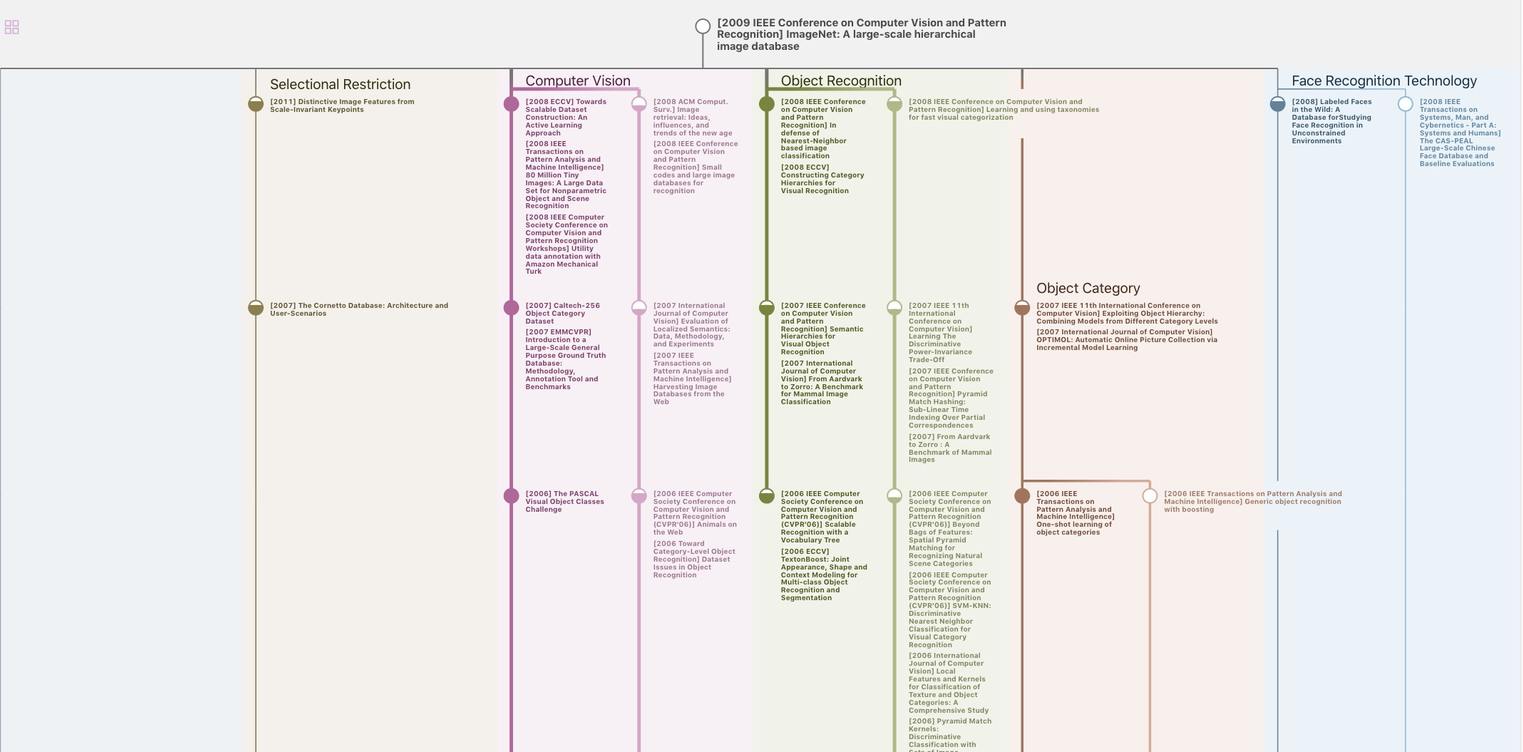
生成溯源树,研究论文发展脉络
Chat Paper
正在生成论文摘要