Improved Infilling of Missing Metadata from Expendable Bathythermographs (XBTs) Using Multiple Machine Learning Methods
JOURNAL OF ATMOSPHERIC AND OCEANIC TECHNOLOGY(2022)
摘要
Historical in situ ocean temperature profile measurements are important for a wide range of ocean and climate research activities. A large proportion of the profile observations have been recorded using expendable bathythermographs (XBTs), and required bias corrections for use in climate change studies. It is generally accepted that the bias, and therefore bias correction, depends on the type of XBT used. However, poor historical metadata collection practices mean the XBT probe type information is often missing, for 59% of profiles between 1967 and 2000, limiting the development of reliable bias corrections. We develop a process of estimating missing instrument type metadata (the combination of both model and manufacturer) systematically, constructing a machine learning pipeline based on thorough data exploration to inform these choices. The predicted instrument type, where missing, will facilitate improved XBT bias corrections. The new approach improves the accuracy of the XBT type classification compared to previous approaches from a recall value of 0.75-0.94. We also develop an approach to account for the uncertainty associated with metadata assignments using ensembles of decision trees, which could feed into an ensemble approach to creating ocean temperature datasets. We describe the challenges arising from the nature of the dataset in applying standard machine learning techniques to the problem. We have implemented this in a portable, reproducible way using standard data science tools, with a view to these techniques being applied to other similar problems in climate science.
更多查看译文
关键词
Ocean, Data quality control, Profilers, oceanic, Software, Classification, Data science, Decision trees, Machine learning
AI 理解论文
溯源树
样例
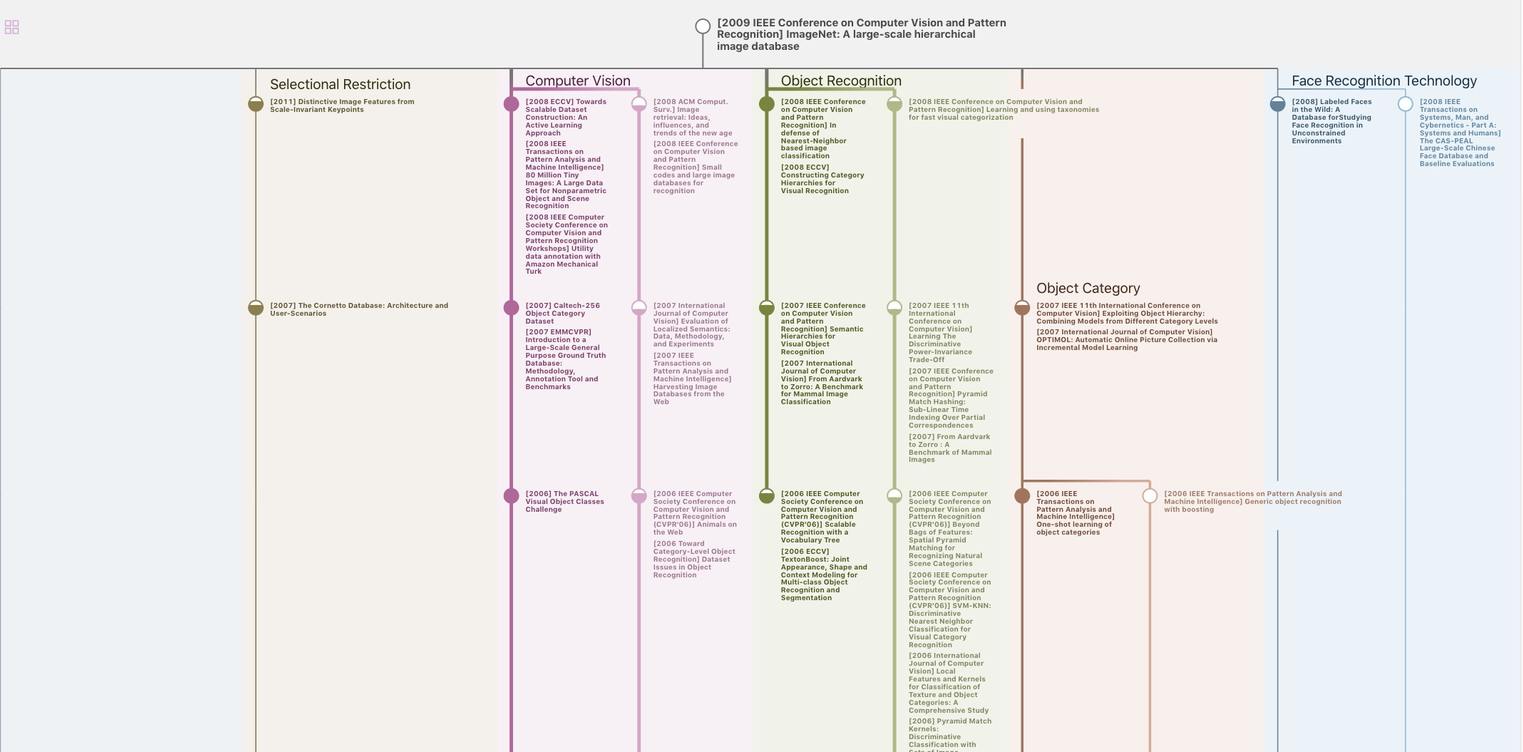
生成溯源树,研究论文发展脉络
Chat Paper
正在生成论文摘要