A Classification Performance Evaluation Measure Considering Data Separability
ARTIFICIAL NEURAL NETWORKS AND MACHINE LEARNING, ICANN 2023, PT I(2023)
摘要
Machine learning and deep learning classification models are data-driven, and the model and the data jointly determine their classification performance. It is biased to evaluate the model's performance only based on the classifier accuracy while ignoring the data separability. Sometimes, the model exhibits excellent accuracy, which might be attributed to its testing on highly separable data. Most of the current studies on data separability measures are defined based on the distance between sample points, but this has been demonstrated to fail in several circumstances. In this paper, we propose a new separability measure-the rate of separability (RS), which is based on the data coding rate. We validate its effectiveness as a supplement to the separability measure by comparing it to four other distance-based measures on synthetic dataset. Then, we discover the positive correlation between the proposed measure and recognition accuracy in a multi-task scenario constructed from a real dataset. Finally, we discuss the methods for evaluating the classification performance of machine learning and deep learning models considering data separability.
更多查看译文
关键词
Machine learning,Classification accuracy,Data separability,Classification difficulty,Performance evaluation
AI 理解论文
溯源树
样例
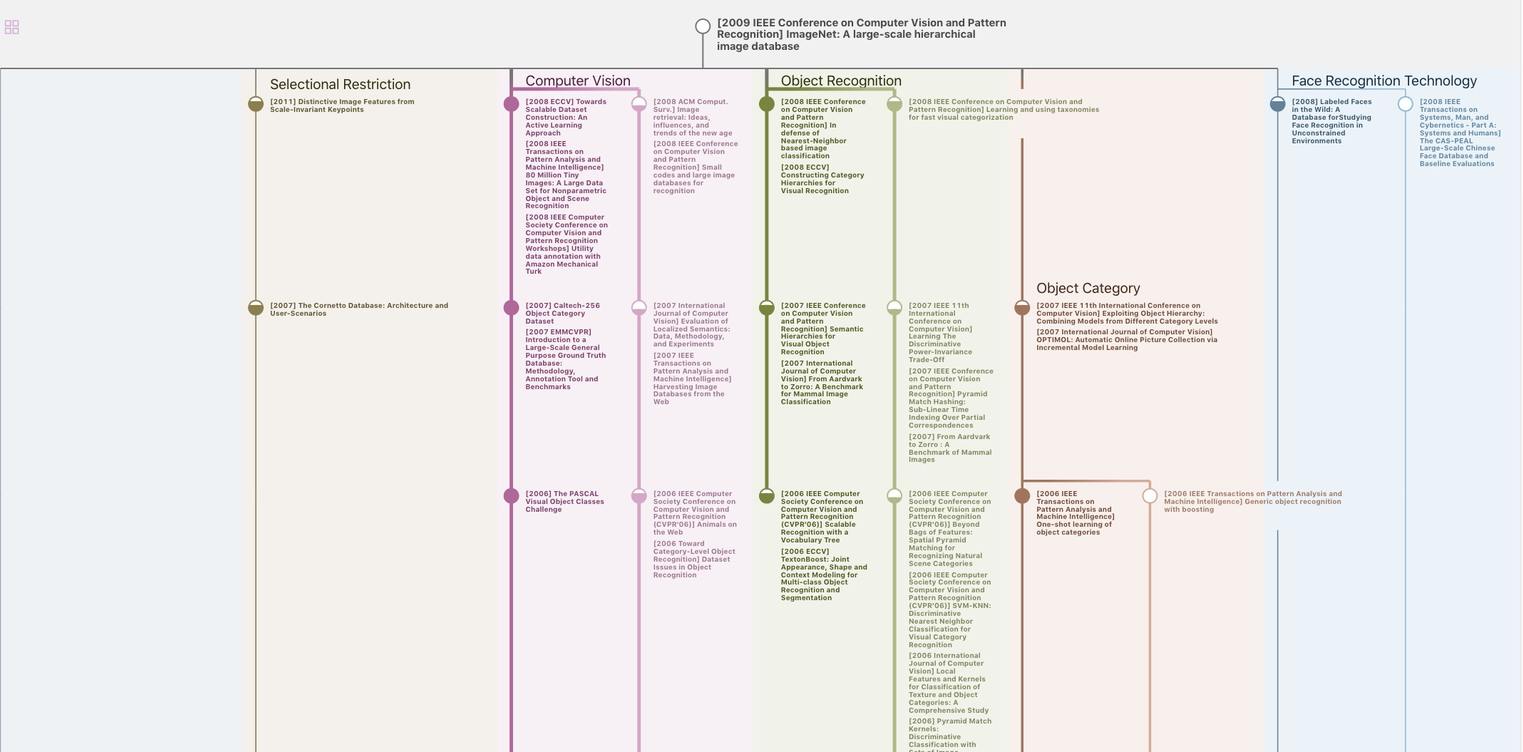
生成溯源树,研究论文发展脉络
Chat Paper
正在生成论文摘要