Temporal Language Modeling for Short Text Document Classification with Transformers
2022 17th Conference on Computer Science and Intelligence Systems (FedCSIS)(2022)
摘要
Language models are typically trained on solely text data, not utilizing document timestamps, which are available in most internet corpora. In this paper, we examine the impact of incorporating timestamp into transformer language model in terms of downstream classification task and masked language modeling on 2 short texts corpora. We examine different times-tamp components: day of the month, month, year, weekday. We test different methods of incorporating date into the model: prefixing date components into text input and adding trained date embeddings. Our study shows, that such a temporal language model performs better than a regular language model for both documents from training data time span and unseen time span. That holds true for classification and language modeling. Prefixing date components into text performs no worse than training special date components embeddings.
更多查看译文
关键词
short text document classification,language
AI 理解论文
溯源树
样例
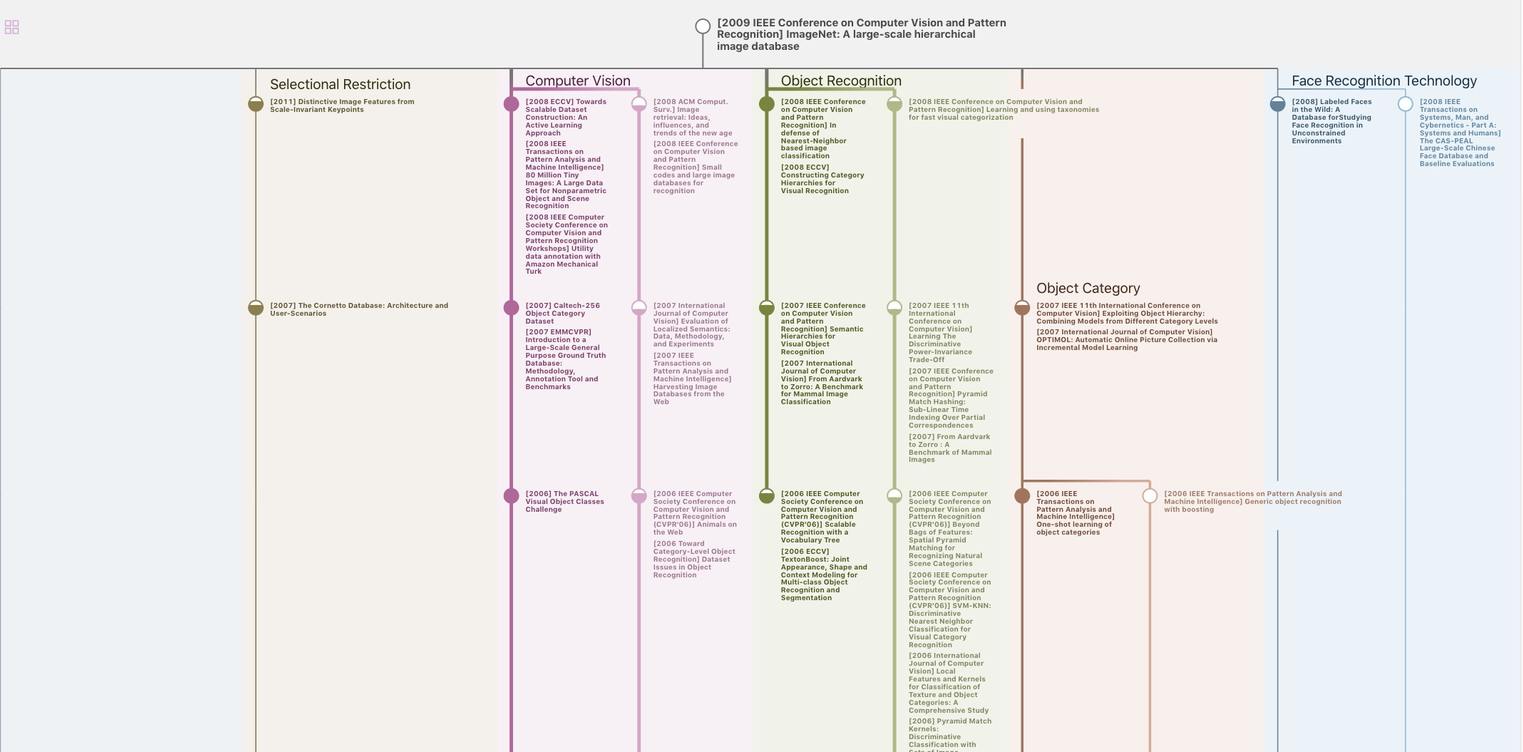
生成溯源树,研究论文发展脉络
Chat Paper
正在生成论文摘要