Relation-dependent contrastive learning with cluster sampling for inductive relation prediction
Neurocomputing(2024)
摘要
Relation prediction is a task designed for knowledge graph completion which aims to predict missing relationships between entities. Recent subgraph-based models for inductive relation prediction have received increasing attention, which can predict relation for unseen entities based on the extracted subgraph surrounding the candidate triplet. However, they are not completely inductive because of their disability of predicting unseen relations. Moreover, they only rely on the graph neural network (GNN) to learn the embedding of extracted subgraph relations and independently assign learnable parameters to each relation, which ignores the role of relations and leads to inaccurate prediction on long-tail relations. In this paper, we introduce Relation-dependent Contrastive Learning (ReCoLe) for inductive relation prediction, which adapts contrastive learning with a novel sampling method based on clustering algorithm to enhance the role of relation and improve the generalization ability to unseen relations. Instead of directly learning embedding for relations, ReCoLe allocates a pre-trained GNN-based encoder for each relation to strengthen the influence of relations. The GNN-based encoder is optimized by contrastive learning, which improves the expressiveness of the embedding of long-tail relations and ensures satisfactory performance. In addition, the cluster sampling method equips ReCoLe with the ability to handle both unseen relations and entities. Experimental results suggest that ReCoLe outperforms state-of-the-art methods on commonly used inductive datasets.
更多查看译文
关键词
Contrastive learning,Inductive relation prediction,Knowledge graph completion,Capsule network,Long-tail situation
AI 理解论文
溯源树
样例
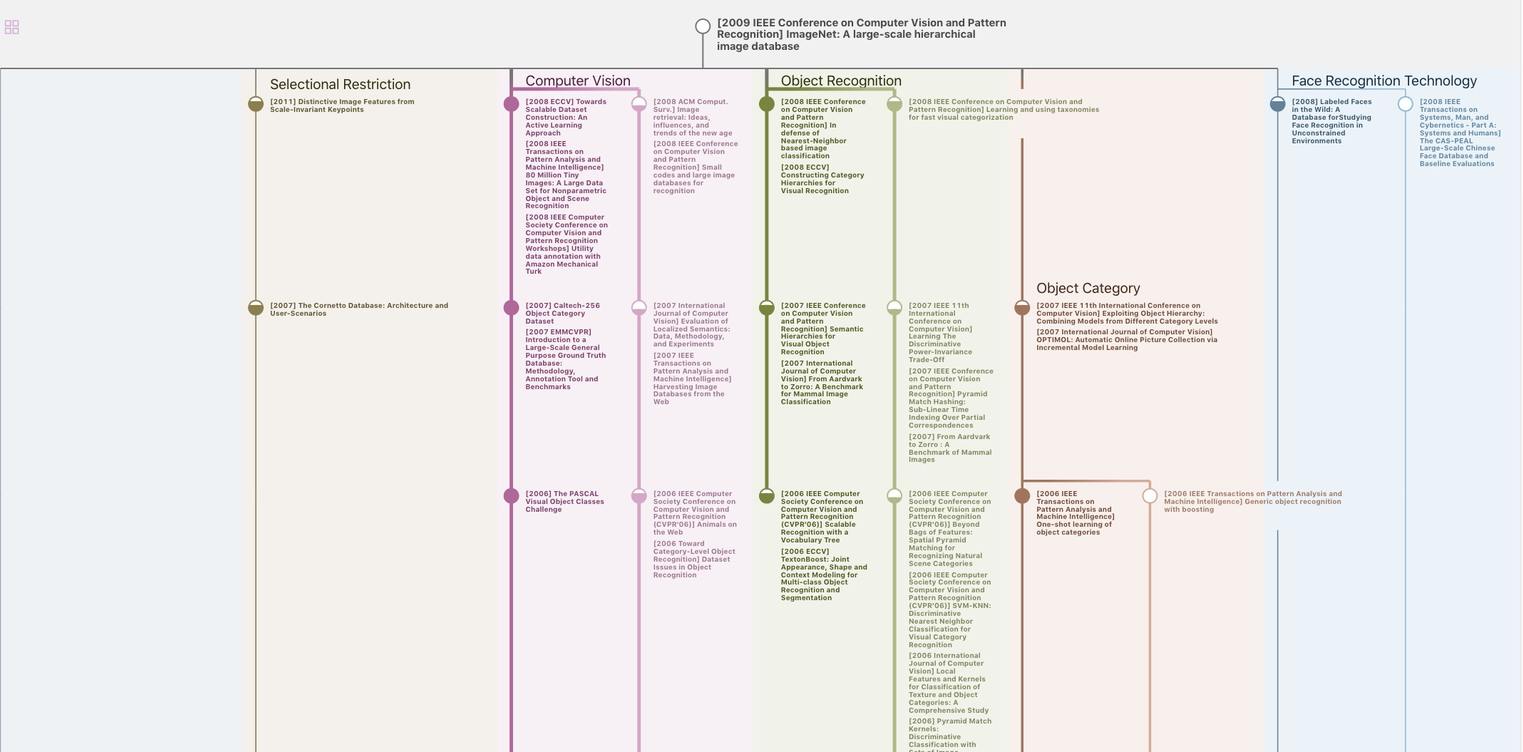
生成溯源树,研究论文发展脉络
Chat Paper
正在生成论文摘要