Improving Energy Efficiency of Convolutional Neural Networks on Multi-core Architectures Through Run-time Reconfiguration
2022 IEEE International Symposium on Circuits and Systems (ISCAS)(2022)
摘要
Convolutional neural networks (CNNs) are built with convolution layers which account for most of their computation time. The differences in the convolution kernel types (2D, point-wise, depth-wise), and input sizes lead to significant differences in their computation and memory demands. In this work, we exploit run-time reconfiguration to adapt to the differences in the characteristics of different convolution kernels on a low-power reconfigurable architecture, Transmuter. The architecture consists of light-weight cores interconnected by caches and crossbars that support run-time reconfiguration between different cache modes - shared or private, different dataflow modes - systolic or parallel, and different computation mapping schemes. To achieve run-time reconfiguration, we propose a decision-tree-based engine that selects the optimal Transmuter configuration at a low cost. The proposed method is evaluated on commonly-used CNN models such as ResNetl8, VGGII, AlexNet and MobileNetV3. Simulation results show that run-time reconfiguration helps improve the energy efficiency of Transmuter in the range of 3.1$\times-13.7\times$ across all networks.
更多查看译文
关键词
Energy-efficiency,CNN,runtime reconfiguration,multicore architecture
AI 理解论文
溯源树
样例
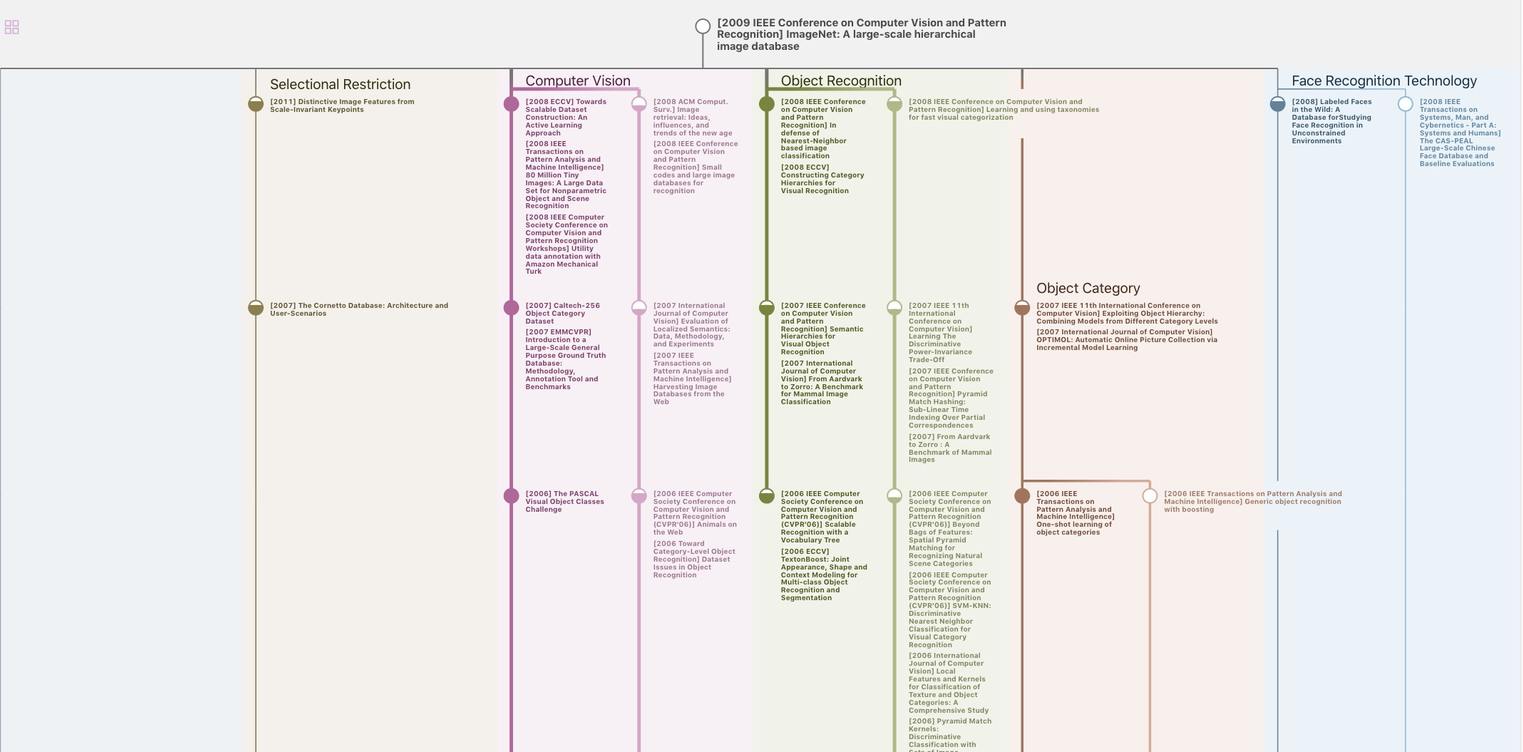
生成溯源树,研究论文发展脉络
Chat Paper
正在生成论文摘要