Retrieval as Attention: End-to-end Learning of Retrieval and Reading within a Single Transformer
CoRR(2022)
摘要
Systems for knowledge-intensive tasks such as open-domain question answering (QA) usually consist of two stages: efficient retrieval of relevant documents from a large corpus and detailed reading of the selected documents to generate answers. Retrievers and readers are usually modeled separately, which necessitates a cumbersome implementation and is hard to train and adapt in an end-to-end fashion. In this paper, we revisit this design and eschew the separate architecture and training in favor of a single Transformer that performs Retrieval as Attention (ReAtt), and end-to-end training solely based on supervision from the end QA task. We demonstrate for the first time that a single model trained end-to-end can achieve both competitive retrieval and QA performance, matching or slightly outperforming state-of-the-art separately trained retrievers and readers. Moreover, end-to-end adaptation significantly boosts its performance on out-of-domain datasets in both supervised and unsupervised settings, making our model a simple and adaptable solution for knowledge-intensive tasks. Code and models are available at https://github.com/jzbjyb/ReAtt.
更多查看译文
关键词
retrieval,attention,learning,reading
AI 理解论文
溯源树
样例
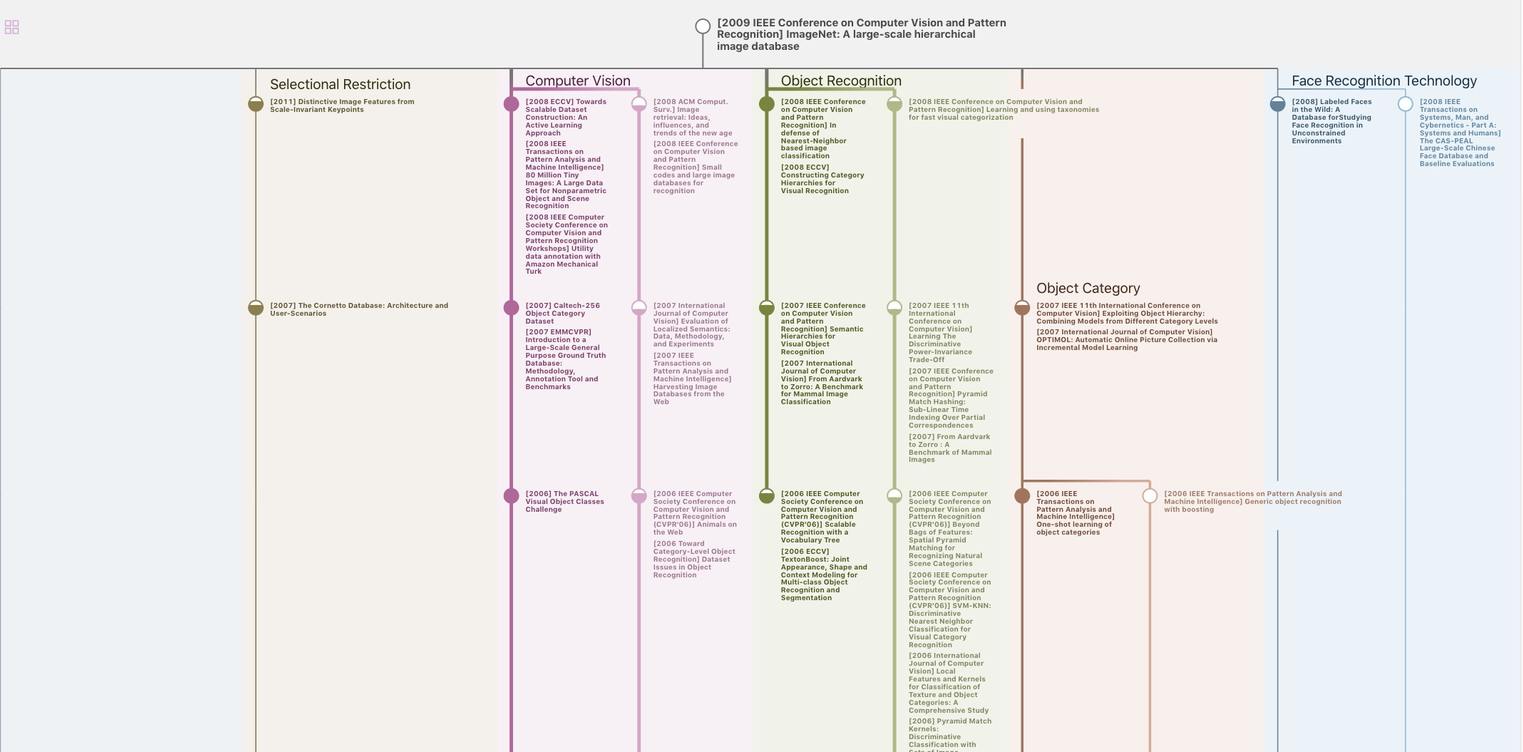
生成溯源树,研究论文发展脉络
Chat Paper
正在生成论文摘要