Visual Query Tuning: Towards Effective Usage of Intermediate Representations for Parameter and Memory Efficient Transfer Learning
CVPR 2023(2023)
摘要
Intermediate features of a pre-trained model have been shown informative for making accurate predictions on downstream tasks, even if the model backbone is frozen. The key challenge is how to utilize them, given the gigantic amount. We propose visual query tuning (VQT), a simple yet effective approach to aggregate intermediate features of Vision Transformers. Through introducing a handful of learnable "query" tokens to each layer, VQT leverages the inner workings of Transformers to "summarize" rich intermediate features of each layer, which can then be used to train the prediction heads of downstream tasks. As VQT keeps the intermediate features intact and only learns to combine them, it enjoys memory efficiency in training, compared to many other parameter-efficient fine-tuning approaches that learn to adapt features and need back-propagation through the entire backbone. This also suggests the complementary role between VQT and those approaches in transfer learning. Empirically, VQT consistently surpasses the state-of-the-art approach that utilizes intermediate features for transfer learning and outperforms full fine-tuning in many cases. Compared to parameter-efficient approaches that adapt features, VQT achieves much higher accuracy under memory constraints. Most importantly, VQT is compatible with these approaches to attain higher accuracy, making it a simple add-on to further boost transfer learning.
更多查看译文
关键词
transfer learning,representations,parameter
AI 理解论文
溯源树
样例
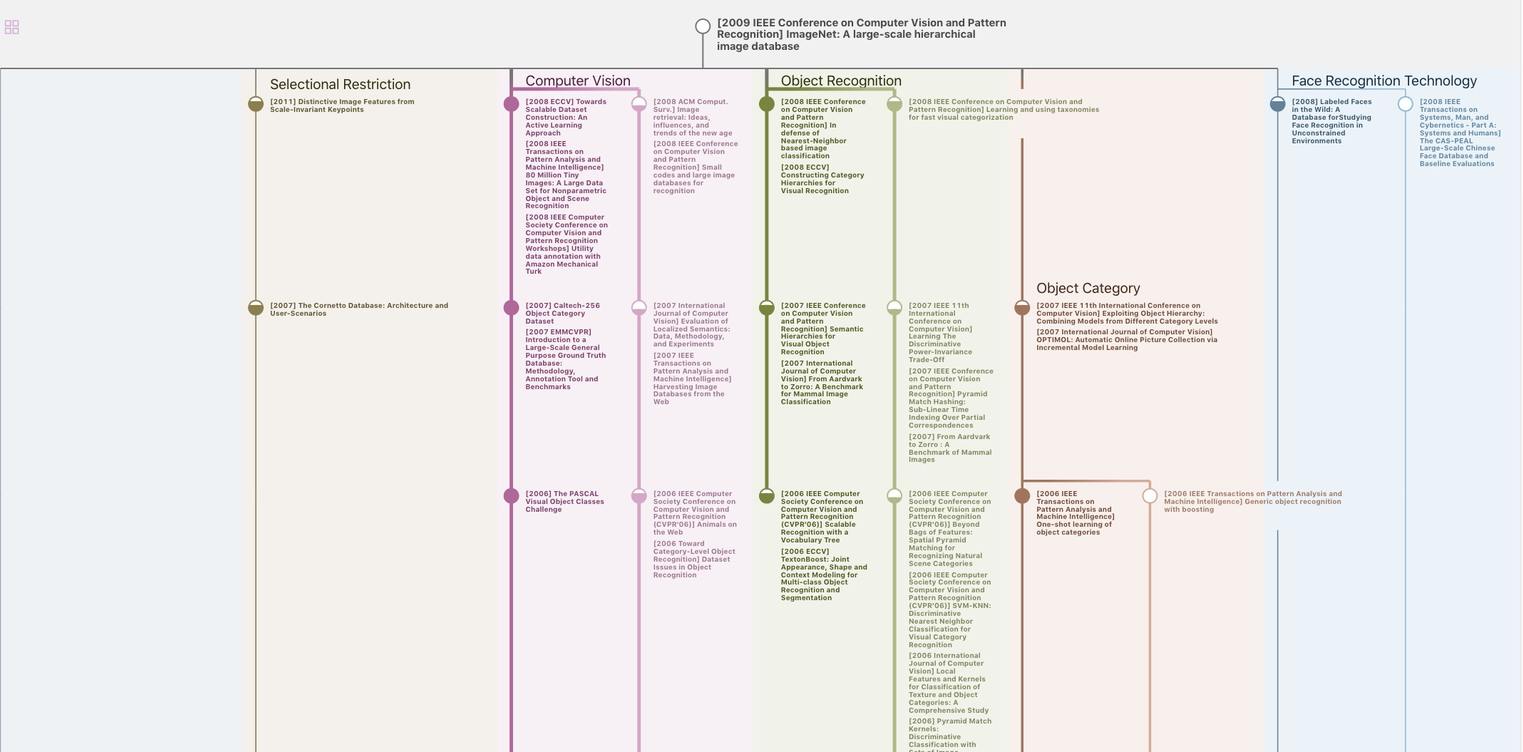
生成溯源树,研究论文发展脉络
Chat Paper
正在生成论文摘要