LEAD: Liberal Feature-based Distillation for Dense Retrieval
PROCEEDINGS OF THE 17TH ACM INTERNATIONAL CONFERENCE ON WEB SEARCH AND DATA MINING, WSDM 2024(2024)
摘要
Knowledge distillation is often used to transfer knowledge from a strong teacher model to a relatively weak student model. Traditional methods include response-based methods and featurebased methods. Response-based methods are widely used but suffer from lower upper limits of performance due to their ignorance of intermediate signals, while feature-based methods have constraints on vocabularies, tokenizers and model architectures. In this paper, we propose a liberal feature-based distillation method (LEAD). LEAD aligns the distribution between the intermediate layers of teacher model and student model, which is effective, extendable, portable and has no requirements on vocabularies, tokenizers, or model architectures. Extensive experiments show the effectiveness of LEAD on widely-used benchmarks, including MS MARCO Passage Ranking, TREC 2019 DL Track, MS MARCO Document Ranking and TREC 2020 DL Track. Our code is available in https://github.com/microsoft/SimXNS/tree/main/LEAD.
更多查看译文
关键词
Dense Retrieval,Knowledge Distillation
AI 理解论文
溯源树
样例
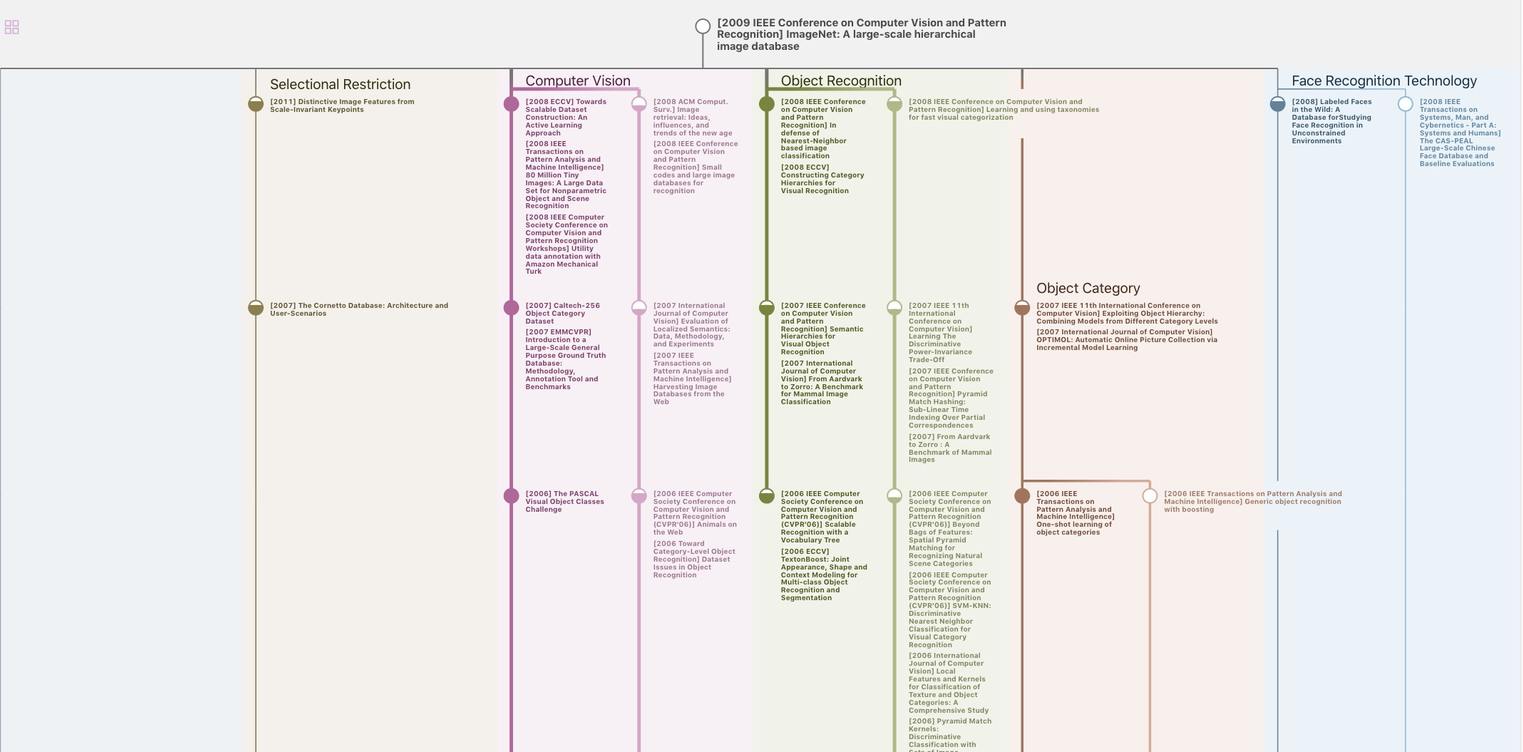
生成溯源树,研究论文发展脉络
Chat Paper
正在生成论文摘要