AnyGrasp: Robust and Efficient Grasp Perception in Spatial and Temporal Domains
ICRA 2024(2024)
摘要
As the basis for prehensile manipulation, it is vital to enable robots to grasp as robustly as humans. Our innate grasping system is prompt, accurate, flexible, and continuous across spatial and temporal domains. Few existing methods cover all these properties for robot grasping. In this paper, we propose AnyGrasp for grasp perception to enable robots these abilities using a parallel gripper. Specifically, we develop a dense supervision strategy with real perception and analytic labels in the spatial-temporal domain. Additional awareness of bjects' center-of-mass is incorporated into the learning process to help improve grasping stability. Utilization of grasp correspondence across observations enables dynamic grasp tracking. Our model can efficiently generate accurate, 7-DoF, dense, and temporally-smooth grasp poses and works robustly against large depth-sensing noise. Using AnyGrasp, we achieve a 93.3% success rate when clearing bins with over 300 unseen objects, which is on par with human subjects under controlled conditions. Over 900 mean-picks-per-hour is reported on a single-arm system. For dynamic grasping, we demonstrate catching swimming robot fish in the water.
更多查看译文
关键词
Perception for Grasping and Manipulation,Grasping,Computer Vision for Automation,Grasp Tracking
AI 理解论文
溯源树
样例
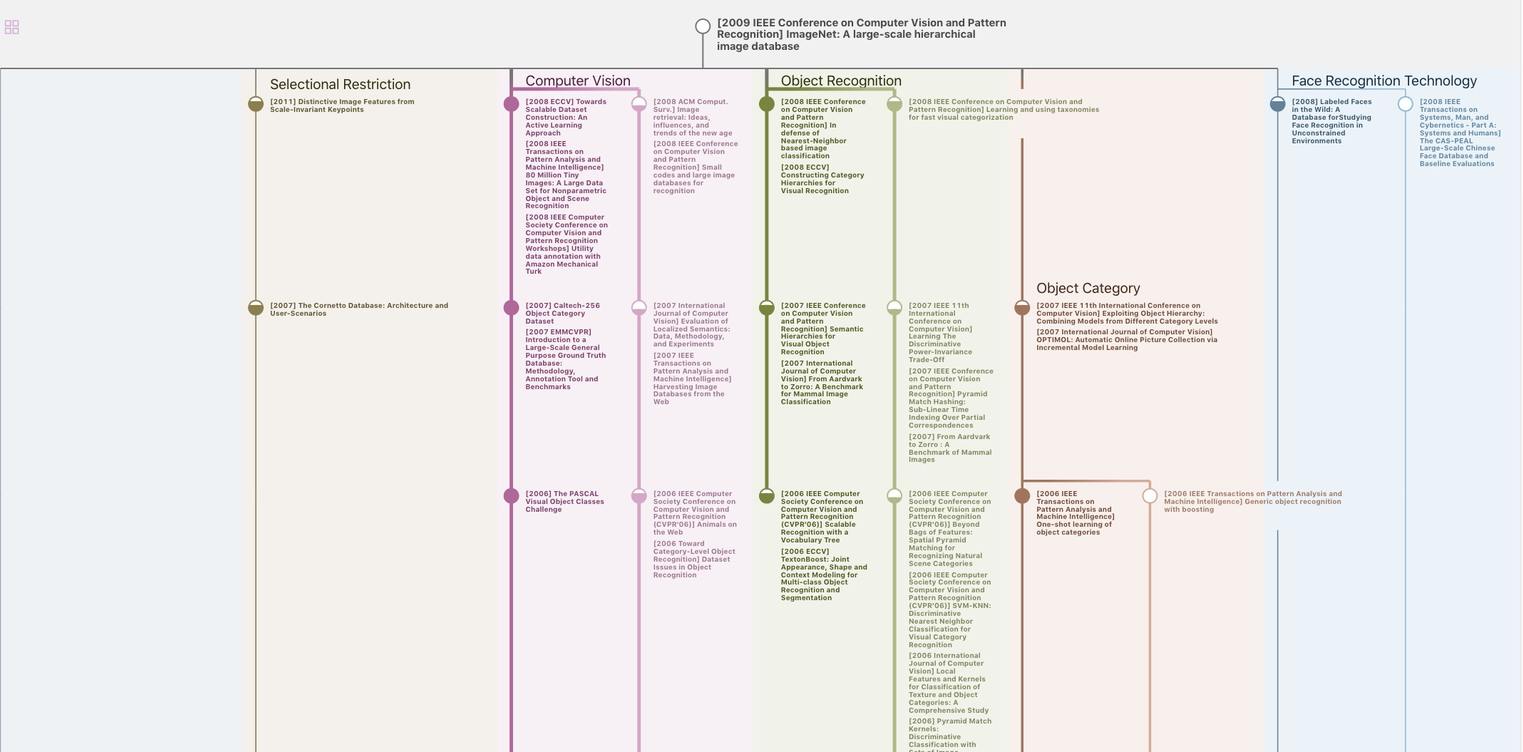
生成溯源树,研究论文发展脉络
Chat Paper
正在生成论文摘要