AugTriever: Unsupervised Dense Retrieval by Scalable Data Augmentation
arxiv(2023)
摘要
Dense retrievers have made significant strides in text retrieval and open-domain question answering, even though most achievements were made possible only with large amounts of human supervision. In this work, we aim to develop unsupervised methods by proposing two methods that create pseudo query-document pairs and train dense retrieval models in an annotation-free and scalable manner: query extraction and transferred query generation. The former method produces pseudo queries by selecting salient spans from the original document. The latter utilizes generation models trained for other NLP tasks (e.g., summarization) to produce pseudo queries. Extensive experiments show that models trained with the proposed augmentation methods can perform comparably well (or better) to multiple strong baselines. Combining those strategies leads to further improvements, achieving the state-of-the-art performance of unsupervised dense retrieval on both BEIR and ODQA datasets.
更多查看译文
关键词
unsupervised dense retrieval,augtriever,augmentation,data
AI 理解论文
溯源树
样例
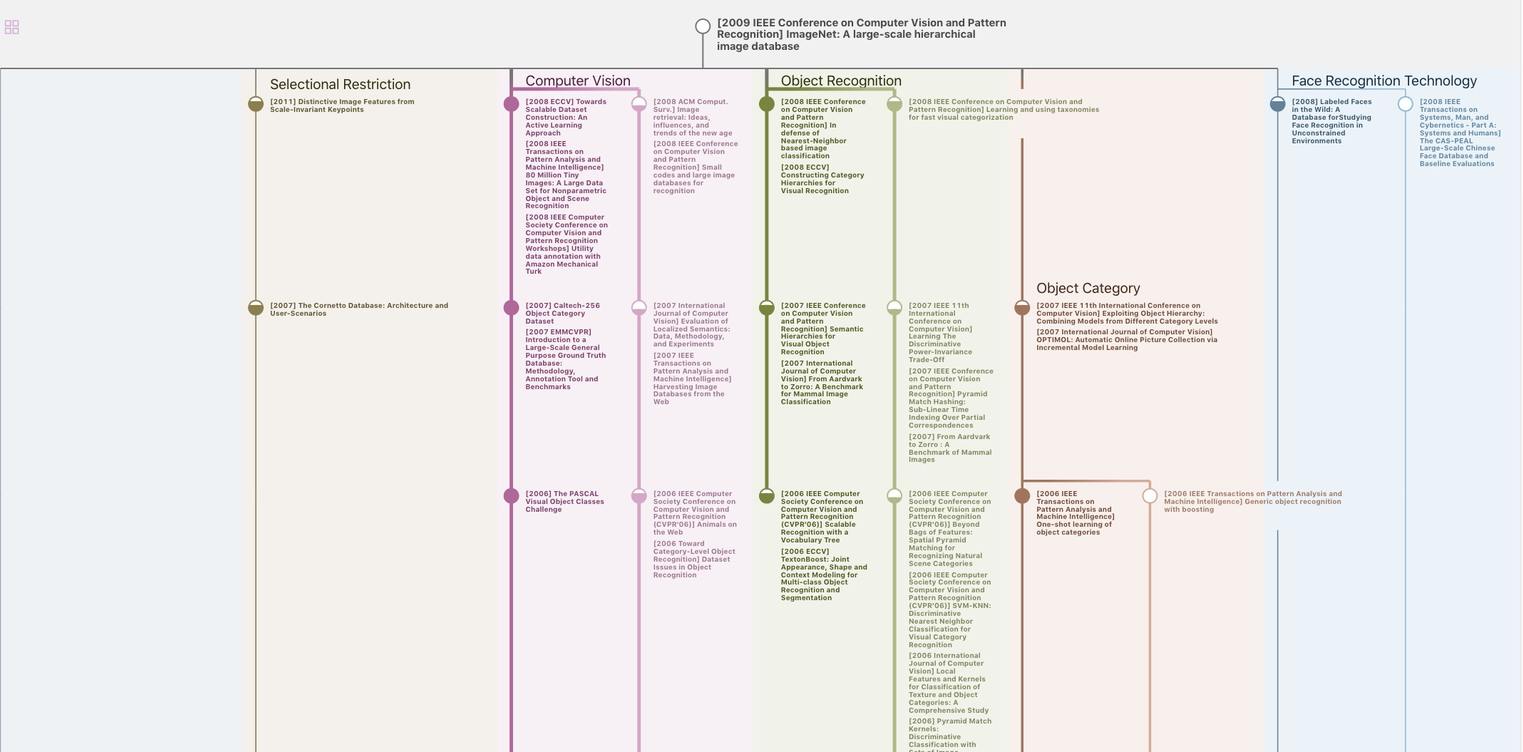
生成溯源树,研究论文发展脉络
Chat Paper
正在生成论文摘要