Calibrate Automated Graph Neural Network via Hyperparameter Uncertainty
Conference on Information and Knowledge Management(2022)
摘要
ABSTRACTAutomated graph learning has drawn widespread research attention due to its great potential to reduce human efforts when dealing with graph data, among which hyperparameter optimization (HPO) is one of the mainstream directions and has made promising progress. However, how to obtain reliable and trustworthy prediction results with automated graph neural networks (GNN) is still quite underexplored. To this end, we investigate automated GNN calibration by marrying uncertainty estimation to the HPO problem. Specifically, we propose a hyperparameter uncertainty-induced graph convolutional network (HyperU-GCN) with a bilevel formulation, where the upper-level problem explicitly reasons uncertainties by developing a probabilistic hypernetworks through a variational Bayesian lens, while the lower-level problem learns how the GCN weights respond to a hyperparameter distribution. By squeezing model uncertainty into the hyperparameter space, the proposed HyperU-GCN could achieve calibrated predictions in a similar way to Bayesian model averaging over hyperparameters. Extensive experimental results on six public datasets were provided in terms of node classification accuracy and expected calibration error (ECE), demonstrating the effectiveness of our approach compared with several state-of-the-art uncertainty-aware and calibrated GCN methods.
更多查看译文
关键词
Graph Neural Networks, Uncertainty Estimation, Hypernetworks
AI 理解论文
溯源树
样例
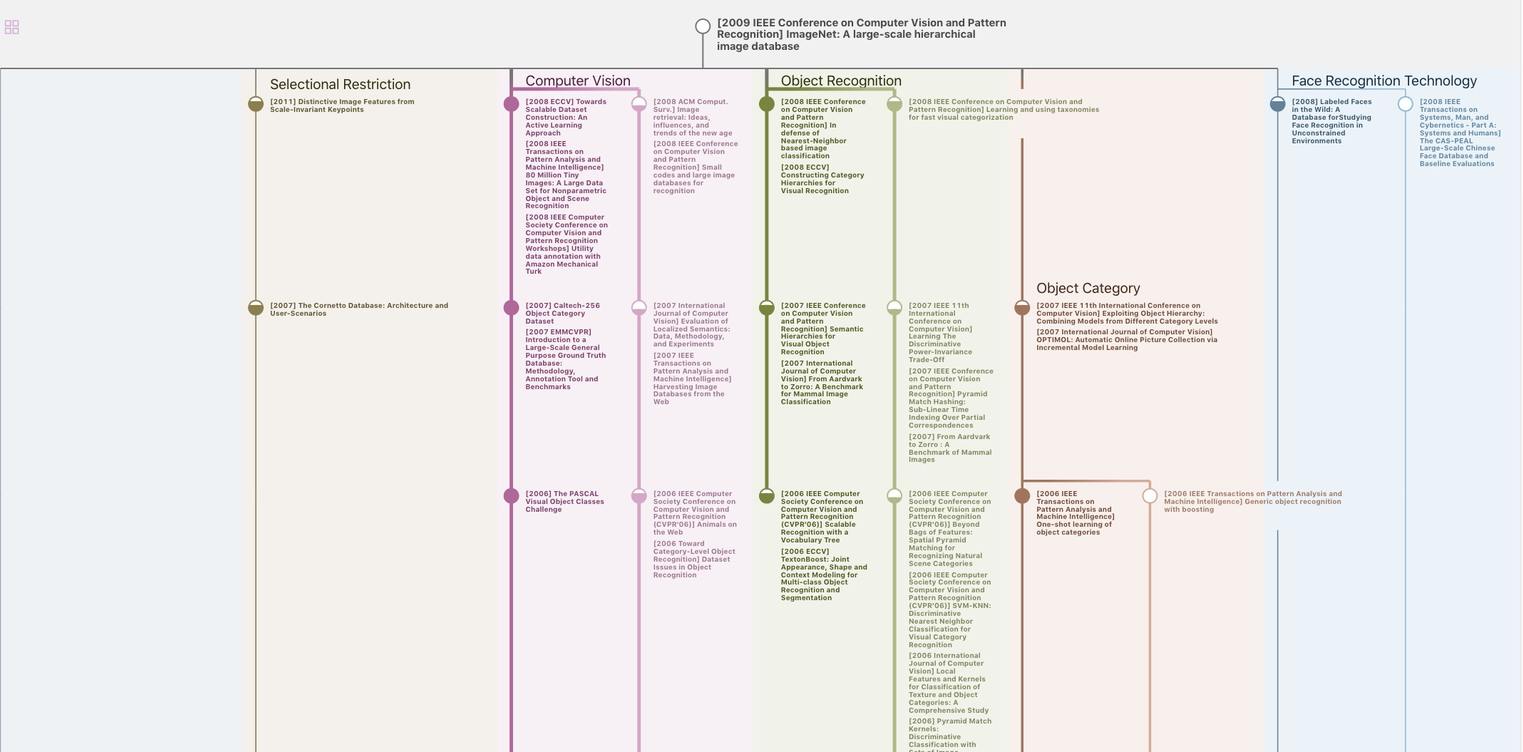
生成溯源树,研究论文发展脉络
Chat Paper
正在生成论文摘要