Hierarchical Spatio-Temporal Graph Neural Networks for Pandemic Forecasting
Conference on Information and Knowledge Management(2022)
摘要
ABSTRACTThe spread of COVID-19 throughout the world has led to cataclysmic consequences on the global community, which poses an urgent need to accurately understand and predict the trajectories of the pandemic. Existing research has relied on graph-structured human mobility data for the task of pandemic forecasting. To perform pandemic forecasting of COVID-19 in the United States, we curate Large-MG, a large-scale mobility dataset that contains 66 dynamic mobility graphs, with each graph having over 3k nodes and an average of 540k edges. One drawback with existing Graph Neural Networks (GNNs) for pandemic forecasting is that they generally perform information propagation in a flat way and thus ignore the inherent community structure in a mobility graph. To bridge this gap, we propose a Hierarchical Spatio-Temporal Graph Neural Network (HiSTGNN) to perform pandemic forecasting, which learns both spatial and temporal information from a sequence of dynamic mobility graphs. HiSTGNN consists of two network architectures. One is a hierarchical graph neural network (HiGNN) that constructs a two-level neural architecture: county-level and region-level, and performs information propagation in a hierarchical way. The other network architecture is a Transformer-based model that captures the temporal dynamics among the sequence of learned node representations from HiGNN. Additionally, we introduce a joint learning objective to further optimize HiSTGNN. Extensive experiments have demonstrated HiSTGNN's superior predictive power of COVID-19 new case/death counts compared with state-of-the-art baselines.
更多查看译文
关键词
pandemic,forecasting,networks,spatio-temporal
AI 理解论文
溯源树
样例
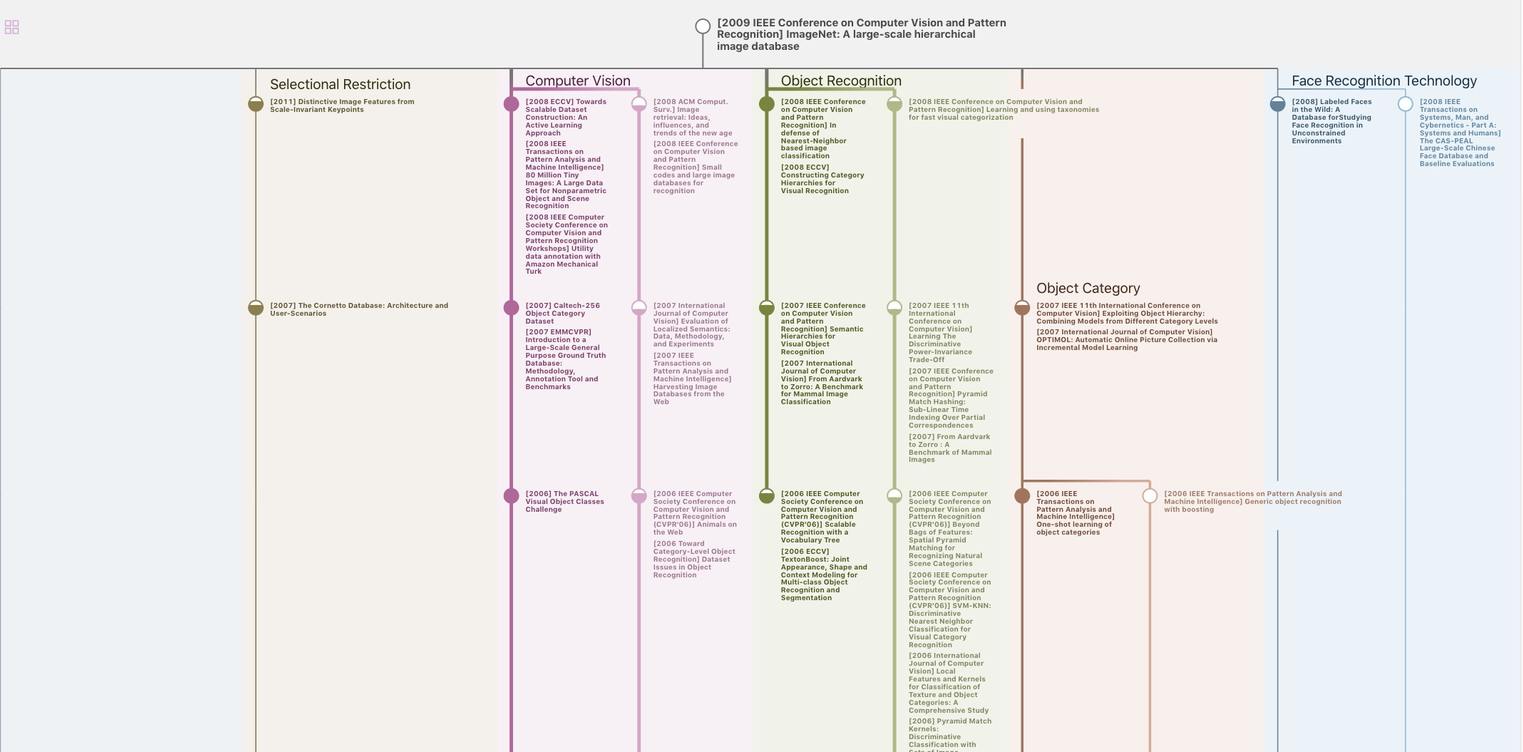
生成溯源树,研究论文发展脉络
Chat Paper
正在生成论文摘要