Distribution preserving-based deep semi-NMF for data representation
Neurocomputing(2023)
摘要
•To reveal the complex relationships hidden in the data space, kernel density estimation is used to approximate the manifold structures embedded in the feature space. To present these complex relationships accurately, we use the strategy of mutual nearest neighbor to estimate the data distributions of original data space. To alleviate the crowding problem of the deep hidden feature space, we design distribution construction with the Cauchy kernel.•To obtain the distribution preserving deep hidden representations, we seamlessly integrate the benefits of the Deep Semi-NMF and the distribution preserving strategy. The proposed DPNMF method further incorporates the consistency of the two distributions between the original data space and deep hidden feature spaces. By preserving the data distributions, our method can learn the better high-level feature representations that have more discriminating power.•The subproblem of updating the feature matrix in each layer is a typical nonconvex bound-constrained optimization problem, so we devise an adaptive projected Barzilai-Borwein method to estimate the step size with a nonmonotone line search or no line search, to ensure the sufficient decrease of the proposed objective function. In addition, the proposed adaptive projected Barzilai-Borwein can also be used to optimize the Deep Semi-NMF and its variants.
更多查看译文
关键词
Deep semi-NMF,Distribution preserving,Manifold structure,Projected Barzilai-Borwein method,Clustering
AI 理解论文
溯源树
样例
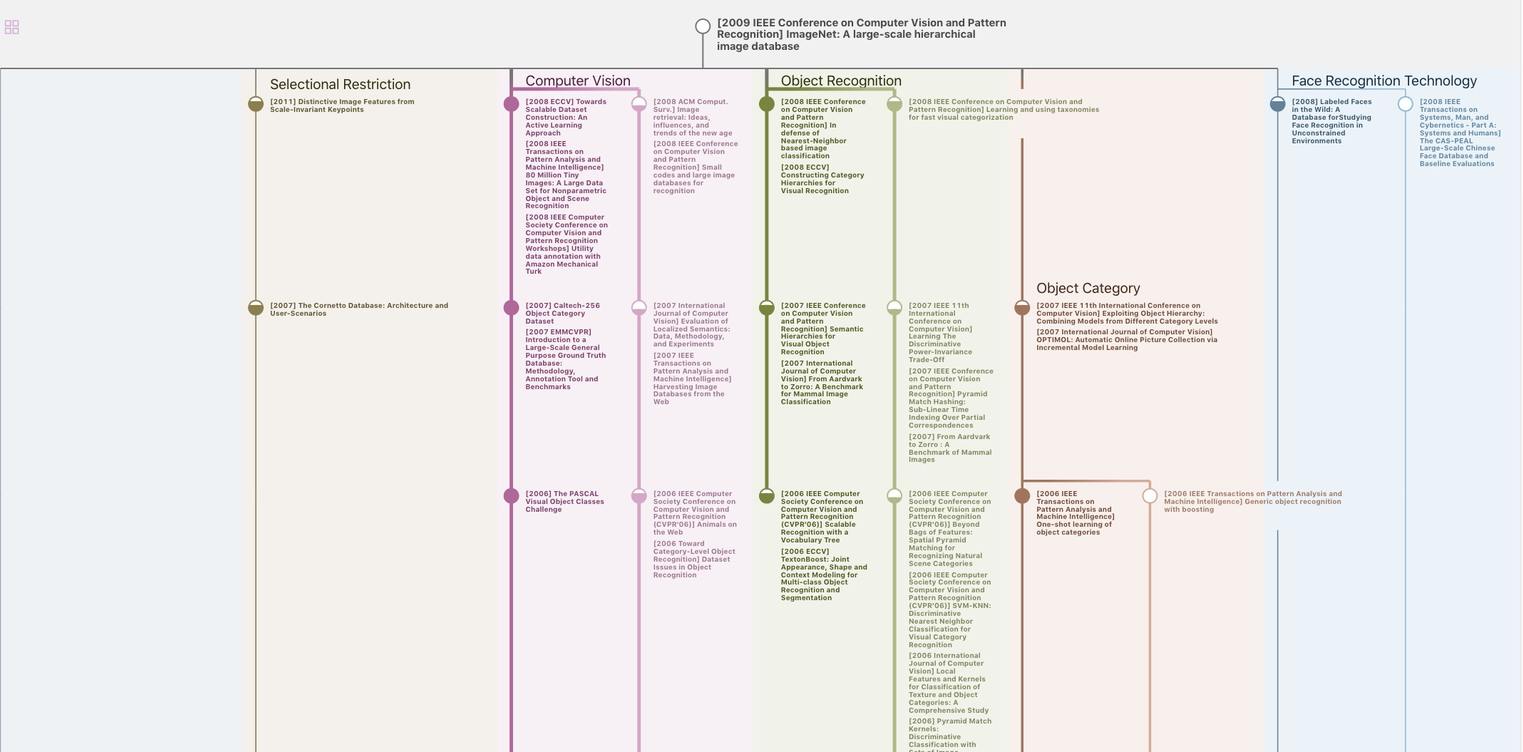
生成溯源树,研究论文发展脉络
Chat Paper
正在生成论文摘要