Efficient Non-sampling Expert Finding.
International Conference on Information and Knowledge Management (CIKM)(2022)
摘要
Expert finding aims at seeking potential users to answer new questions in Community Question Answering (CQA) websites. Most existing methods focus on designing matching frameworks between questions and experts, and rely on negative sampling technology for model training. However, sampling would lose lots of useful information about experts and questions, and make these sampling-based methods suffer the bias and non-robust issues, which may lead to an insufficient matching performance for expert findings. In this paper, we propose a novel Efficient Non-sampling Expert Finding model, named ENEF, which could learn accurate representations of questions and experts from whole training data. In our approach, we adopt a rather basic question encoder and a simple matching framework, then an efficient whole-data optimization method is elaborately designed to learn the model parameters without negative sampling with rather a low space and time complexity. Extensive experimental results on four real-world CQA datasets demonstrate that our model ENEF could achieve better performance and faster training efficiency than existing state-of-the-art expert finding methods.
更多查看译文
关键词
non-sampling
AI 理解论文
溯源树
样例
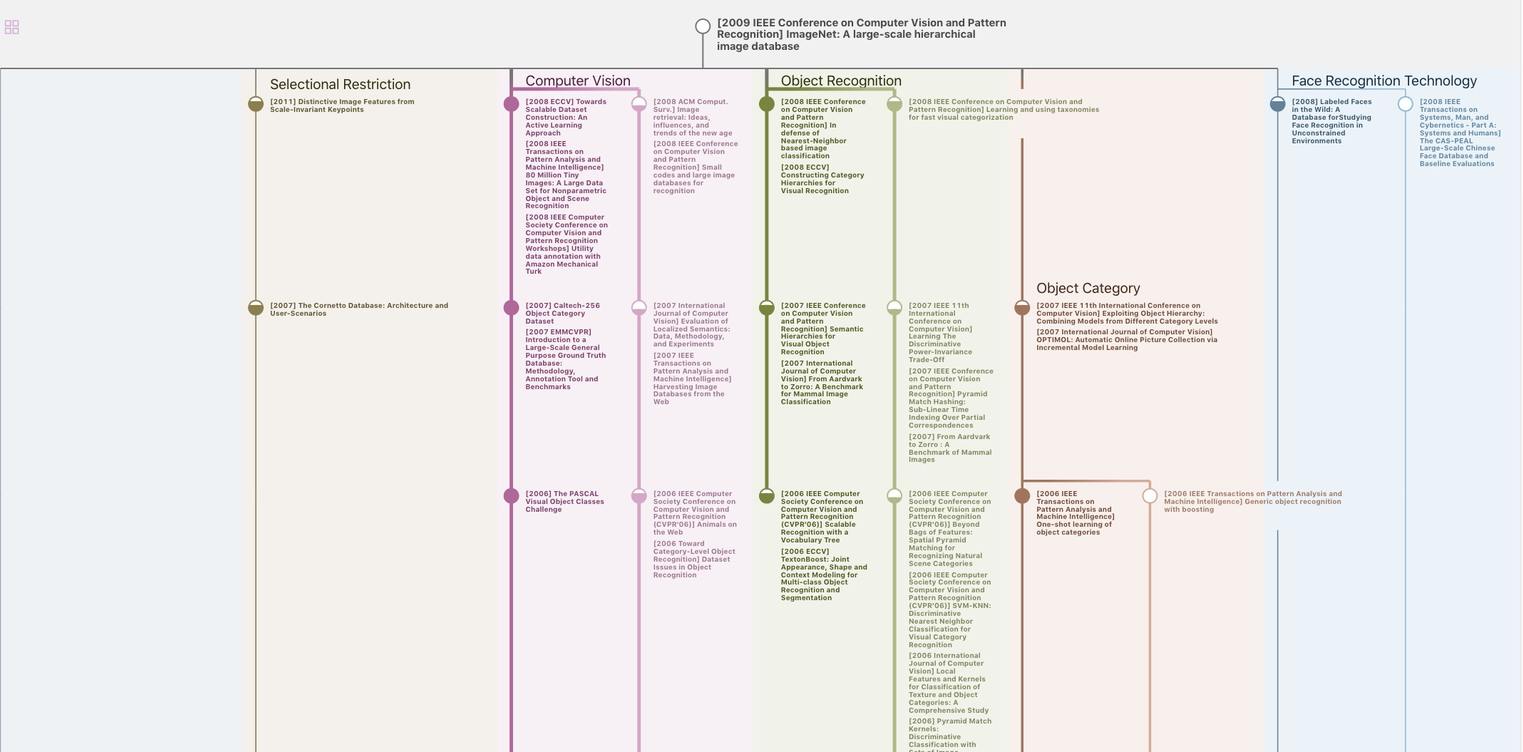
生成溯源树,研究论文发展脉络
Chat Paper
正在生成论文摘要