Point Processes for Competing Observations with Recurrent Networks (POPCORN): A Generative Model of EHR Data.
Machine Learning in Health Care (MLHC)(2021)
摘要
Modeling EHR data is of significant interest in a broad range of applications including prediction of future conditions or building latent representations of patient history. This can be challenging because EHR data is multivariate and irregularly sampled. Traditional treatments of EHR data involve handling irregular sampling by imputation or discretization. In this work, we model the full longitudinal history of a patient using a generative multivariate point process that simultaneously: (1) Models irregularly sampled events probabilistically without discretization or interpolation (2) Has a closed-form likelihood, making training straightforward (3) Encodes dependence between times and events with an approach inspired by competing risk models (4) Allows for direct sampling. We show improved performance on next-event prediction compared to existing approaches. Our proposed framework could potentially be used in many different contexts including prediction, generation of synthetic data and building latent representations of patient history.
更多查看译文
关键词
recurrent networks,generative model,competing observations,data
AI 理解论文
溯源树
样例
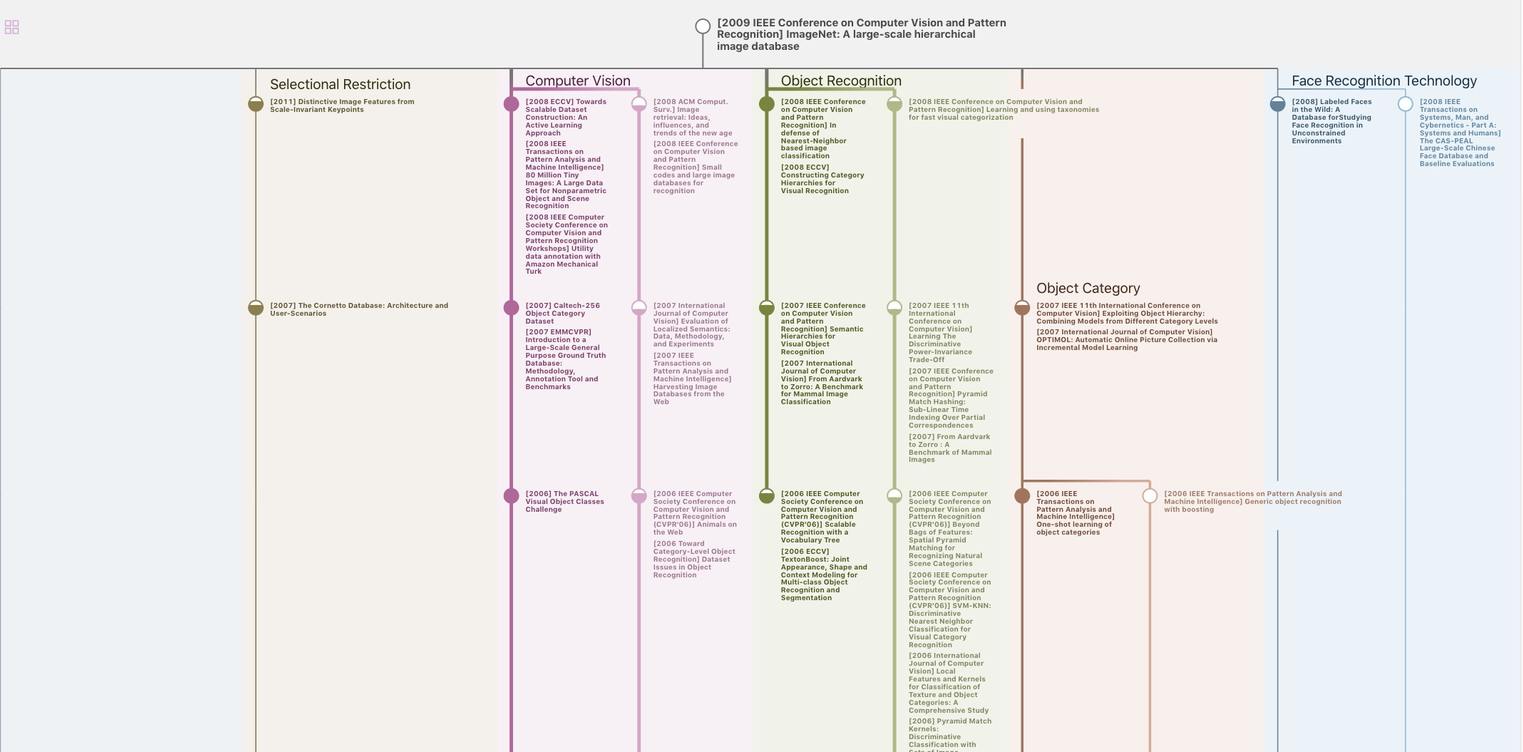
生成溯源树,研究论文发展脉络
Chat Paper
正在生成论文摘要