From Local to Holistic: Self-supervised Single Image 3D Face Reconstruction Via Multi-level Constraints.
IEEE/RJS International Conference on Intelligent RObots and Systems (IROS)(2022)
摘要
Single image 3D face reconstruction with accurate geometric details is a critical and challenging task due to the similar appearance on the face surface and fine details in organs. In this work, we introduce a self-supervised 3D face reconstruction approach from a single image that can recover detailed textures under different camera settings. The proposed network learns high-quality disparity maps from stereo face images during the training stage, while just a single face image is required to generate the 3D model in real applications. To recover fine details of each organ and facial surface, the framework introduces facial landmark spatial consistency to constrain the face recovering learning process in local point level and segmentation scheme on facial organs to constrain the correspondences at the organ level. The face shape and textures will further be refined by establishing holistic constraints based on the varying light illumination and shading information. The proposed learning framework can recover more accurate 3D facial details both quantitatively and qualitatively compared with state-of-the-art 3DMM and geometry-based reconstruction algorithms based on a single image.
更多查看译文
关键词
3DMM,accurate 3D facial details,accurate geometric details,camera settings,detailed textures,face shape,face surface,facial landmark spatial consistency,facial organs,facial surface,geometry-based reconstruction algorithms,high-quality disparity maps,holistic constraints,learning process,local point level,multilevel constraints,segmentation scheme,self-supervised single image 3D face reconstruction,shading information,single face image,stereo face images,varying light illumination
AI 理解论文
溯源树
样例
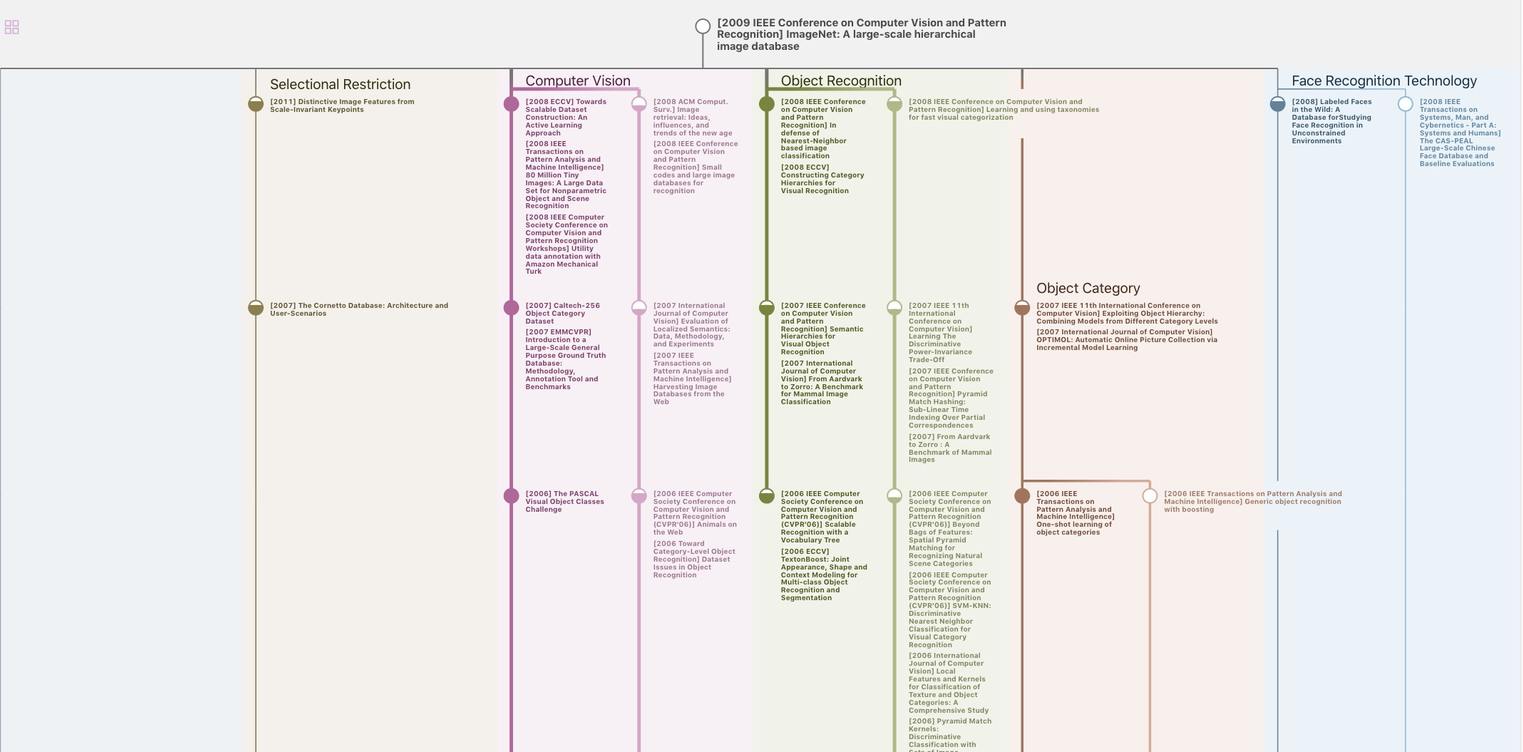
生成溯源树,研究论文发展脉络
Chat Paper
正在生成论文摘要