WSRC: Weakly Supervised Faster RCNN Toward Accurate Traffic Object Detection
IEEE Access(2023)
摘要
Weakly supervised object detection has attracted increasing interest in recent years as driven by data annotation bottleneck in deep network training. On the other hand, the performance of weakly supervised models still lags far behind due to the missing of object location information during training. This paper presents a Weakly Supervised Faster RCNN (WSRC) that aims to train accurate traffic object detectors without using any box annotations, addressing the phenomenon that the traffic object detector trained by traditional method cannot be applied in the real traffic scene due to the lack of data annotation. The WSRC designs an innovative proposal evaluation network (PEN) that is trained by using a large amount of proposals that are scored according to their Intersection-over-Union (IoU) overlap with pseudo ground-truth boxes. Novel loss functions are designed to teach the PEN and WSRC to identify good proposals and regress to accurate object boxes under the guidance of loss dynamics and proposal scores. The proposed WSRC achieves superior detection performance over the datasets Pascal VOC2007, MS COCO and Cityscape, demonstrating its effectiveness while short of annotation boxes.
更多查看译文
关键词
Weakly supervised object detection,proposal evaluation network,traffic object detection
AI 理解论文
溯源树
样例
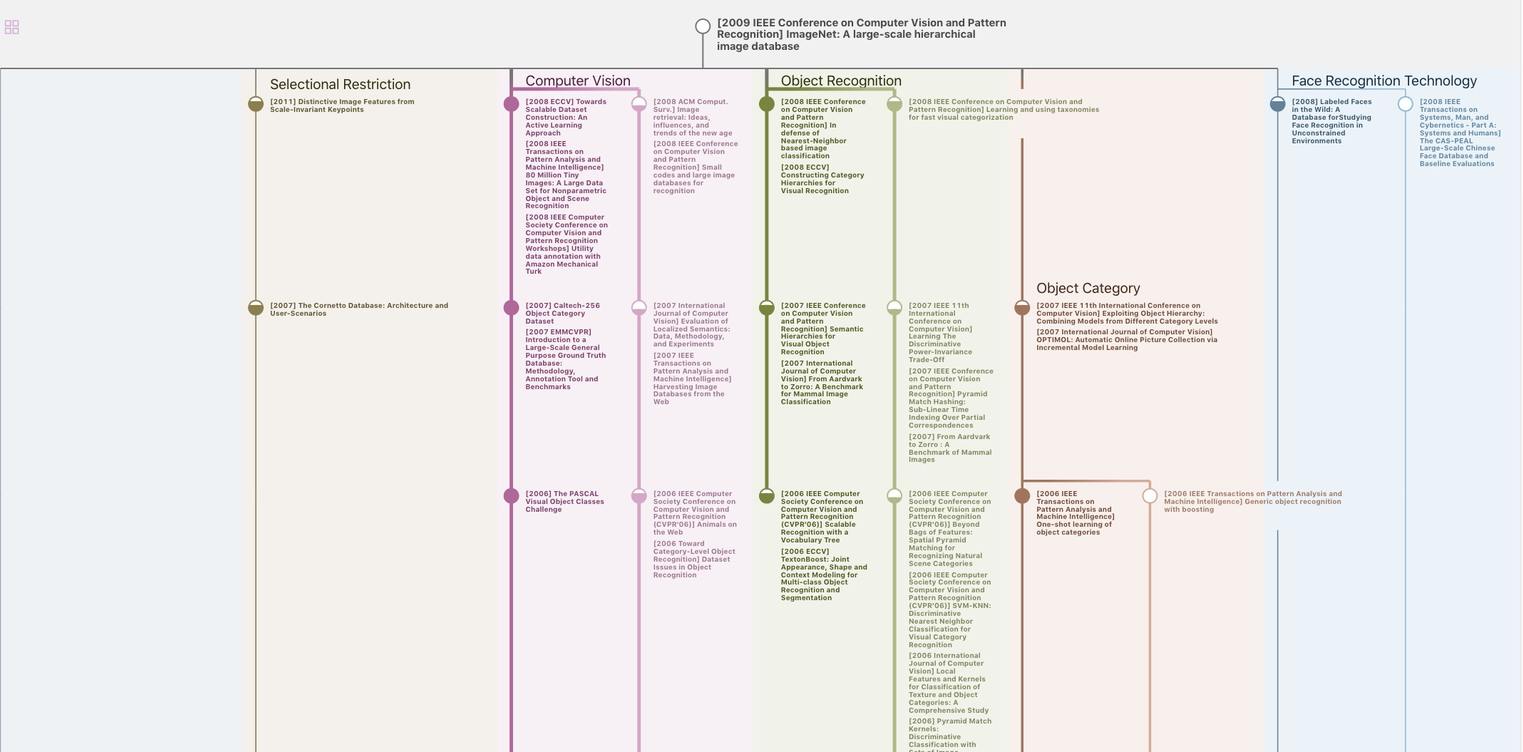
生成溯源树,研究论文发展脉络
Chat Paper
正在生成论文摘要