InPars-Light: Cost-Effective Unsupervised Training of Efficient Rankers
arXiv (Cornell University)(2023)
摘要
We carried out a reproducibility study of InPars recipe for unsupervised training of neural rankers. As a by-product of this study, we developed a simple-yet-effective modification of InPars, which we called InPars-light. Unlike InPars, InPars-light uses only a freely available language model BLOOM and 7x-100x smaller ranking models. On all five English retrieval collections (used in the original InPars study) we obtained substantial (7-30%) and statistically significant improvements over BM25 in nDCG or MRR using only a 30M parameter six-layer MiniLM ranker. In contrast, in the InPars study only a 100x larger MonoT5-3B model consistently outperformed BM25, whereas their smaller MonoT5-220M model (which is still 7x larger than our MiniLM ranker), outperformed BM25 only on MS MARCO and TREC DL 2020. In a purely unsupervised setting, our 435M parameter DeBERTA v3 ranker was roughly at par with the 7x larger MonoT5-3B: In fact, on three out of five datasets, it slightly outperformed MonoT5-3B. Finally, these good results were achieved by re-ranking only 100 candidate documents compared to 1000 used in InPars. We believe that InPars-light is the first truly cost-effective prompt-based unsupervised recipe to train and deploy neural ranking models that outperform BM25.
更多查看译文
关键词
training,efficient,inpars-light,cost-effective
AI 理解论文
溯源树
样例
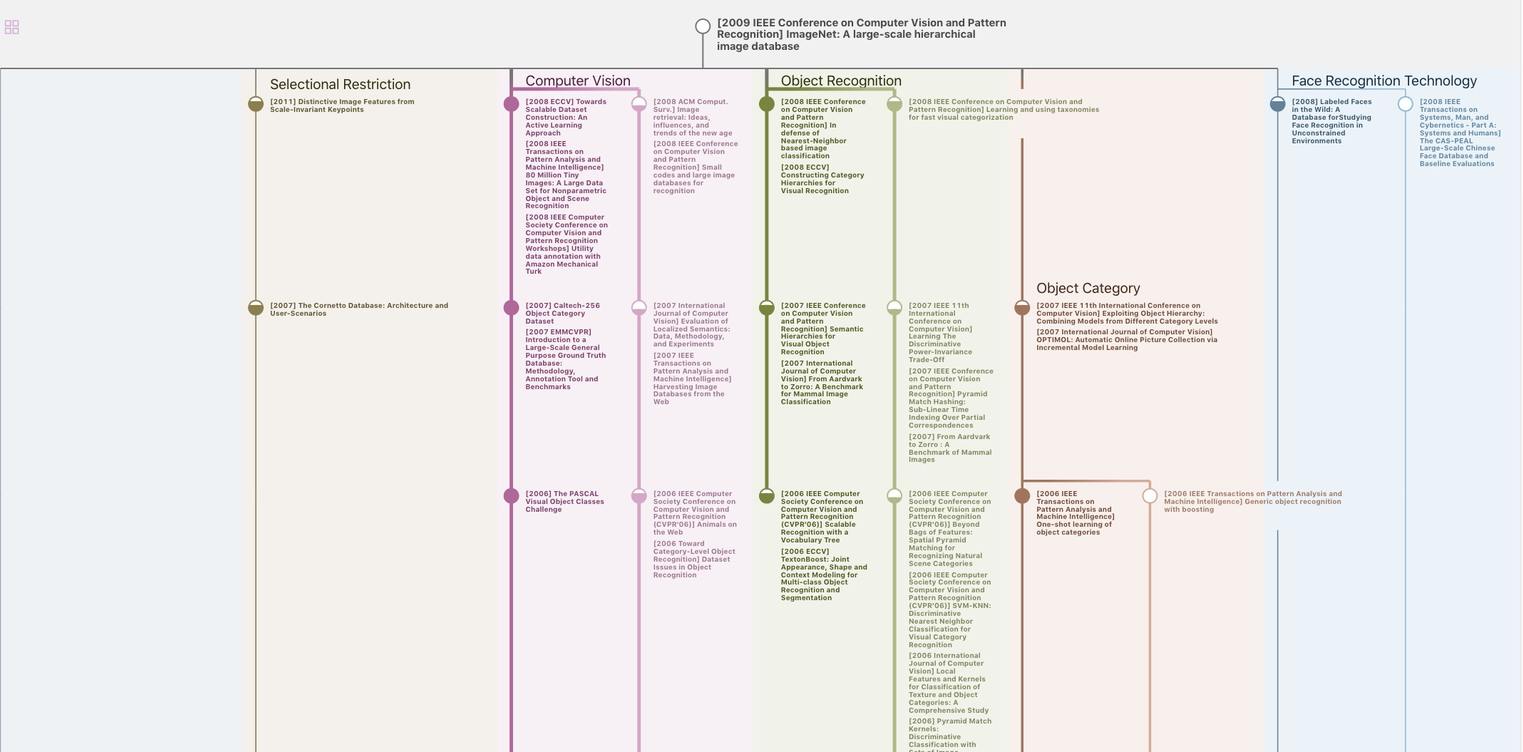
生成溯源树,研究论文发展脉络
Chat Paper
正在生成论文摘要