MOTOR: A Time-To-Event Foundation Model for Structured Medical Records
ICLR 2024(2024)
摘要
We present a self-supervised, time-to-event (TTE) foundation model called MOTOR (Many Outcome Time Oriented Representations) which is pretrained on timestamped sequences of events in electronic health records (EHR) and health insurance claims. TTE models are used for estimating the probability distribution of the time until a specific event occurs, which is an important task in medical settings. TTE models provide many advantages over classification using fixed time horizons, including naturally handling censored observations, but are challenging to train with limited labeled data. MOTOR addresses this challenge by pretraining on up to 55M patient records (9B clinical events). We evaluate MOTOR's transfer learning performance on 19 tasks, across 3 patient databases (a private EHR system, MIMIC-IV, and Merative claims data). Task-specific models adapted from MOTOR improve time-dependent C statistics by 4.6\% over state-of-the-art, improve label efficiency by up to 95\% ,and are more robust to temporal distributional shifts. We further evaluate cross-site portability by adapting our MOTOR foundation model for six prediction tasks on the MIMIC-IV dataset, where it outperforms all baselines. MOTOR is the first foundation model for medical TTE predictions and we release a 143M parameter pretrained model for research use at https://huggingface.co/StanfordShahLab/motor-t-base.
更多查看译文
关键词
foundation models,time-to-event,electronic health records,deep learning,self-supervised learning,transfer learning
AI 理解论文
溯源树
样例
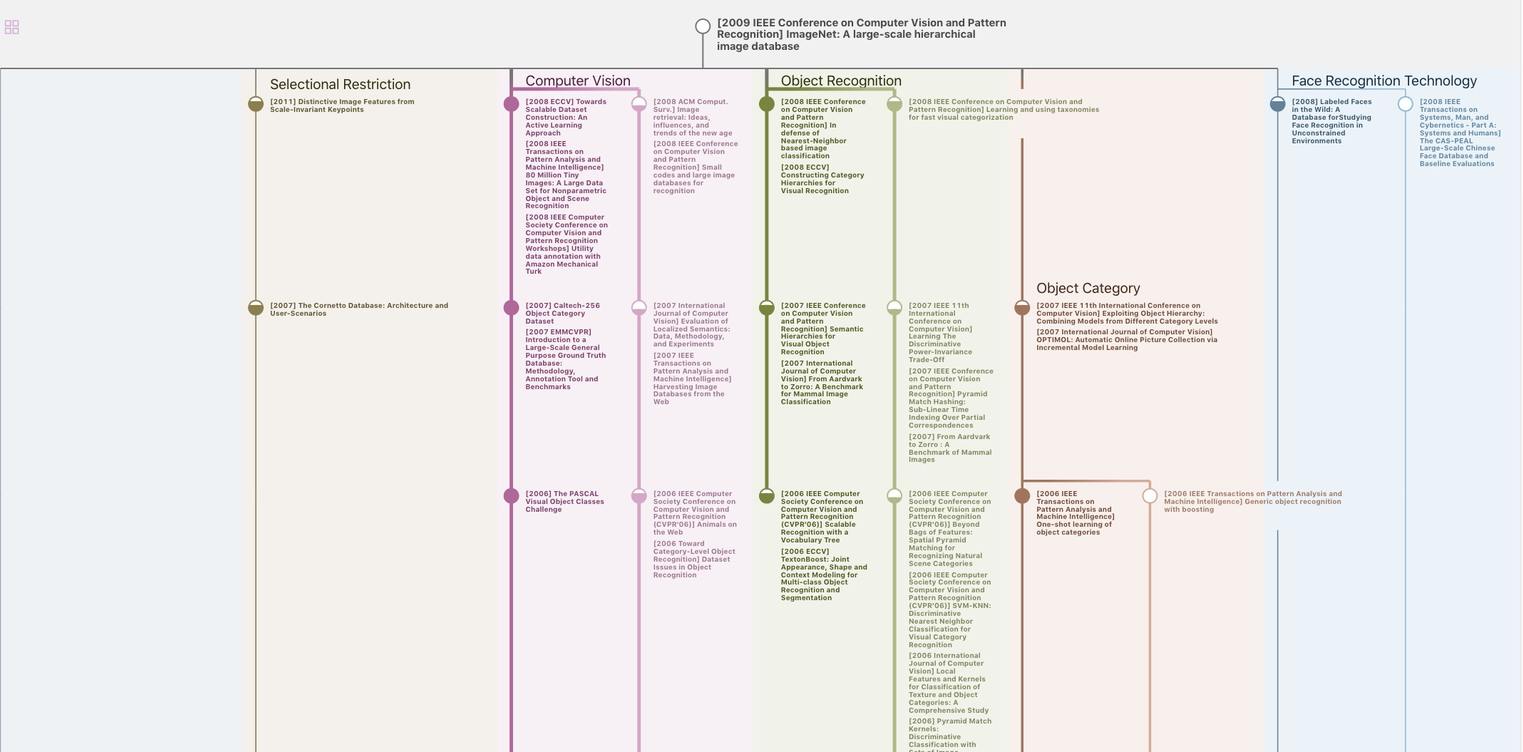
生成溯源树,研究论文发展脉络
Chat Paper
正在生成论文摘要