Dealing with Textual Noise for Robust and Effective BERT Re-Ranking.
INFORMATION PROCESSING & MANAGEMENT(2023)
摘要
The pre-trained language models (PLMs), such as BERT, have been successfully employed in two-phases ranking pipeline for information retrieval (IR). Meanwhile, recent studies have reported that BERT model is vulnerable to imperceptible textual perturbations on quite a few natural language processing (NLP) tasks. As for IR tasks, current established BERT re-ranker is mainly trained on large-scale and relatively clean dataset, such as MS MARCO, but actually noisy text is more common in real-world scenarios, such as web search. In addition, the impact of within-document textual noises (perturbations) on retrieval effectiveness remains to be investigated, especially on the ranking quality of BERT re-ranker, considering its contextualized nature. To mitigate this gap, we carry out exploratory experiments on the MS MARCO dataset in this work to examine whether BERT re-ranker can still perform well when ranking text with noise. Unfortunately, we observe non-negligible effectiveness degradation of BERT re-ranker over a total of ten different types of synthetic within-document textual noise. Furthermore, to address the effectiveness losses over textual noise, we propose a novel noise-tolerant model, De-Ranker, which is learned by minimizing the distance between the noisy text and its original clean version. Our evaluation on the MS MARCO and TREC 2019–2020 DL datasets demonstrates that De-Ranker can deal with synthetic textual noise more effectively, with 3%–4% performance improvement over vanilla BERT re-ranker. Meanwhile, extensive zero-shot transfer experiments on a total of 18 widely-used IR datasets show that De-Ranker can not only tackle natural noise in real-world text, but also achieve 1.32% improvement on average in terms of cross-domain generalization ability on the BEIR benchmark. • The first investigation into the effects of within-document textual noise on BERT re-ranker. • Effectiveness of BERT re-ranker declines when coming across textual noises. • Synthetic noise injected into MS MARCO can be useful to enhance BERT re-ranker. • De-Ranker can effectively deal with textual noise by learning a noise-invariant relevance estimation.
更多查看译文
关键词
Textual noise,BERT re-ranking,Ranking model robustness,Text perturbation,Text information retrieval
AI 理解论文
溯源树
样例
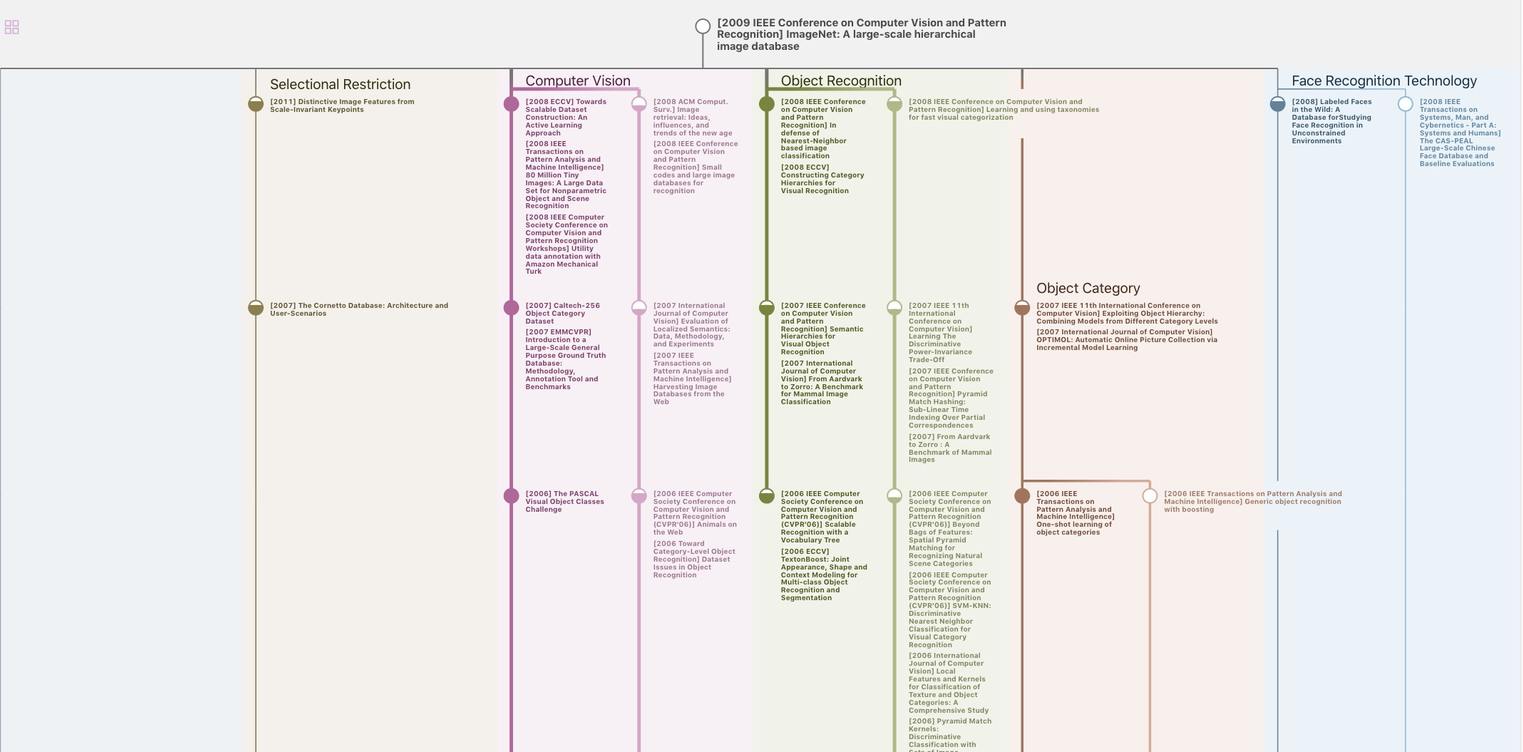
生成溯源树,研究论文发展脉络
Chat Paper
正在生成论文摘要