Autobidders with Budget and ROI Constraints: Efficiency, Regret, and Pacing Dynamics
arXiv (Cornell University)(2023)
摘要
We study a game between autobidding algorithms that compete in an online
advertising platform. Each autobidder is tasked with maximizing its
advertiser's total value over multiple rounds of a repeated auction, subject to
budget and return-on-investment constraints. We propose a gradient-based
learning algorithm that is guaranteed to satisfy all constraints and achieves
vanishing individual regret. Our algorithm uses only bandit feedback and can be
used with the first- or second-price auction, as well as with any
"intermediate" auction format. Our main result is that when these autobidders
play against each other, the resulting expected liquid welfare over all rounds
is at least half of the expected optimal liquid welfare achieved by any
allocation. This holds whether or not the bidding dynamics converges to an
equilibrium.
更多查看译文
关键词
roi constraints,budget,pacing dynamics,efficiency
AI 理解论文
溯源树
样例
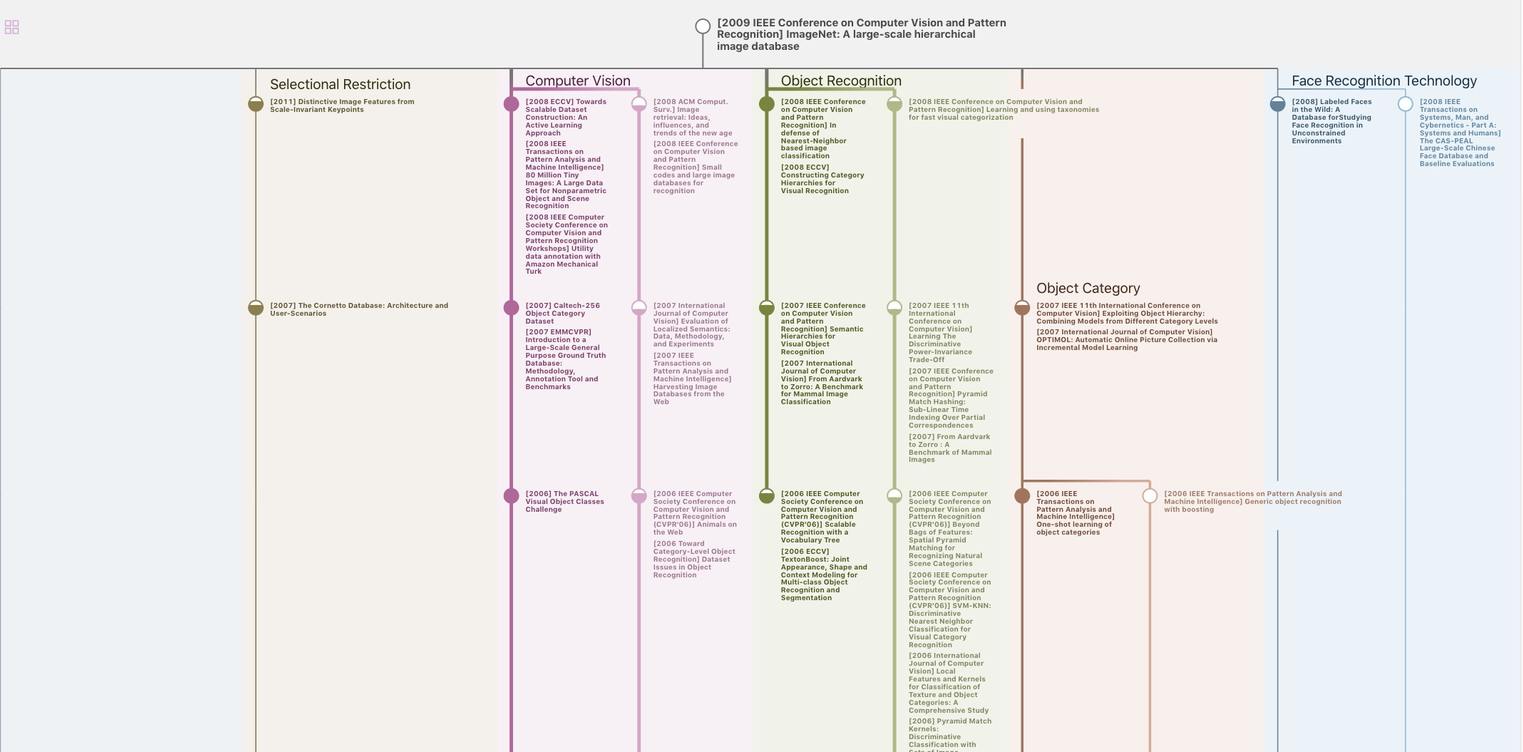
生成溯源树,研究论文发展脉络
Chat Paper
正在生成论文摘要