A widely-used eddy covariance gap-filling method creates systematic bias in carbon balance estimates
Scientific reports(2023)
摘要
Climate change mitigation requires, besides reductions in greenhouse gas emissions, actions to increase carbon sinks in terrestrial ecosystems. A key measurement method for quantifying such sinks and calibrating models is the eddy covariance technique, but it requires imputation, or gap-filling, of missing data for determination of annual carbon balances of ecosystems. Previous comparisons of gap-filling methods have concluded that commonly used methods, such as marginal distribution sampling (MDS), do not have a significant impact on the carbon balance estimate. By analyzing an extensive, global data set, we show that MDS causes significant carbon balance errors for northern (latitude >60^∘ ) sites. MDS systematically overestimates the carbon dioxide (CO _2 ) emissions of carbon sources and underestimates the CO _2 sequestration of carbon sinks. We also reveal reasons for these biases and show how a machine learning method called extreme gradient boosting or a modified implementation of MDS can be used to substantially reduce the northern site bias.
更多查看译文
关键词
Atmospheric science,Carbon cycle,Science,Humanities and Social Sciences,multidisciplinary
AI 理解论文
溯源树
样例
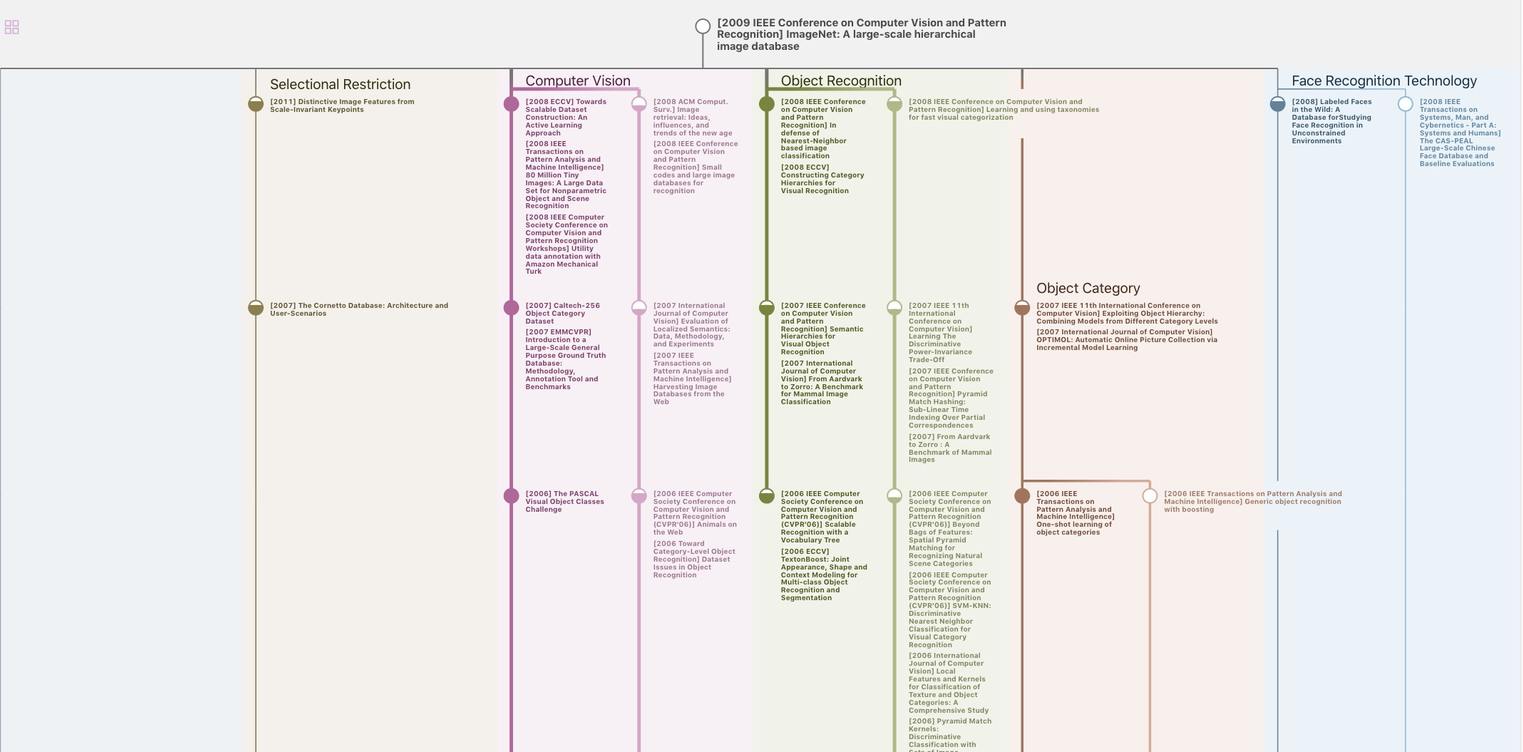
生成溯源树,研究论文发展脉络
Chat Paper
正在生成论文摘要