Weighted Clock Logic Point Process
ICLR 2023(2023)
摘要
Datasets involving multivariate event streams are prevalent in numerous applications. We present a novel framework for modeling temporal point processes called clock logic neural networks (CLNN) which learn weighted clock logic (wCL) formulas as interpretable temporal rules by which some events promote or inhibit other events. Specifically, CLNN models temporal relations between events using conditional intensity rates informed by a set of wCL formulas, which are more expressive than related prior work. Unlike conventional approaches of searching for generative rules through expensive combinatorial optimization, we design smooth activation functions for components of wCL formulas that enable a continuous relaxation of the discrete search space and efficient learning of wCL formulas using gradient-based methods. Experiments on synthetic datasets manifest our model's ability to recover the ground-truth rules and improve computational efficiency. In addition, experiments on real-world datasets show that our models perform competitively when compared with state-of-the-art models.
更多查看译文
关键词
Multivariate event data,Neuro-symbolic models,Temporal point process,Propositional logic
AI 理解论文
溯源树
样例
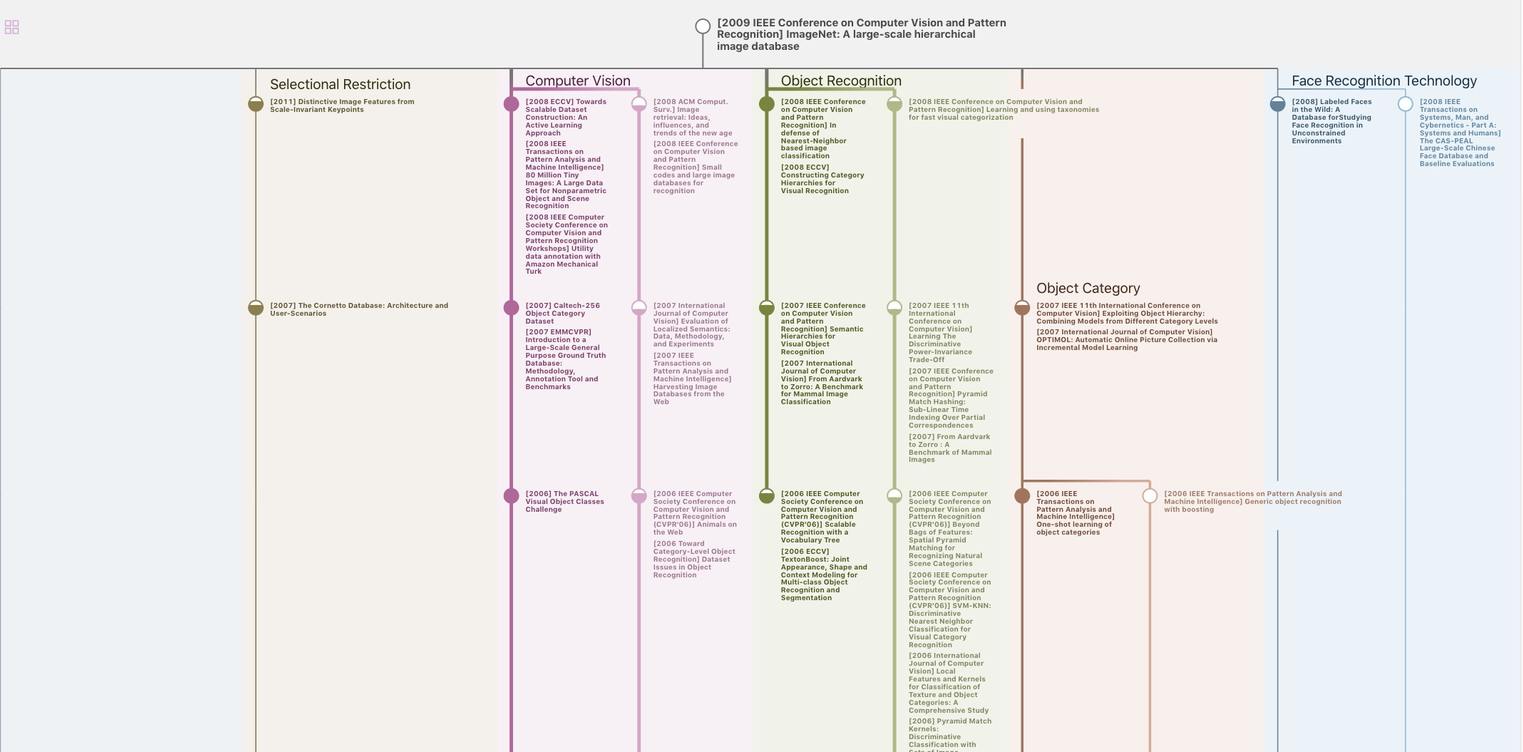
生成溯源树,研究论文发展脉络
Chat Paper
正在生成论文摘要