Neural Image-based Avatars: Generalizable Radiance Fields for Human Avatar Modeling
ICLR 2023(2023)
摘要
We present a method that enables synthesizing novel views and novel poses of arbitrary human performers from sparse multi-view images. A key ingredient of our method is a hybrid appearance blending module that combines the advantages of the implicit body NeRF representation and image-based rendering. Existing generalizable human NeRF methods that are conditioned on the body model have shown robustness against the geometric variation of arbitrary human performers. Yet they often exhibit blurry results when generalized onto unseen identities. Meanwhile, image-based rendering shows high-quality results when sufficient observations are available, whereas it suffers artifacts in sparse-view settings. We propose Neural Image-based Avatars (NIA) that exploits the best of those two methods: to maintain robustness under new articulations and self-occlusions while directly leveraging the available (sparse) source view colors to preserve appearance details of new subject identities. Our hybrid design outperforms recent methods on both in-domain identity generalization as well as challenging cross-dataset generalization settings. Also, in terms of the pose generalization, our method outperforms even the per-subject optimized animatable NeRF methods.
更多查看译文
关键词
Generalizable human radiance fields,Human performance capture,Human NeRF,Neural radiance fields
AI 理解论文
溯源树
样例
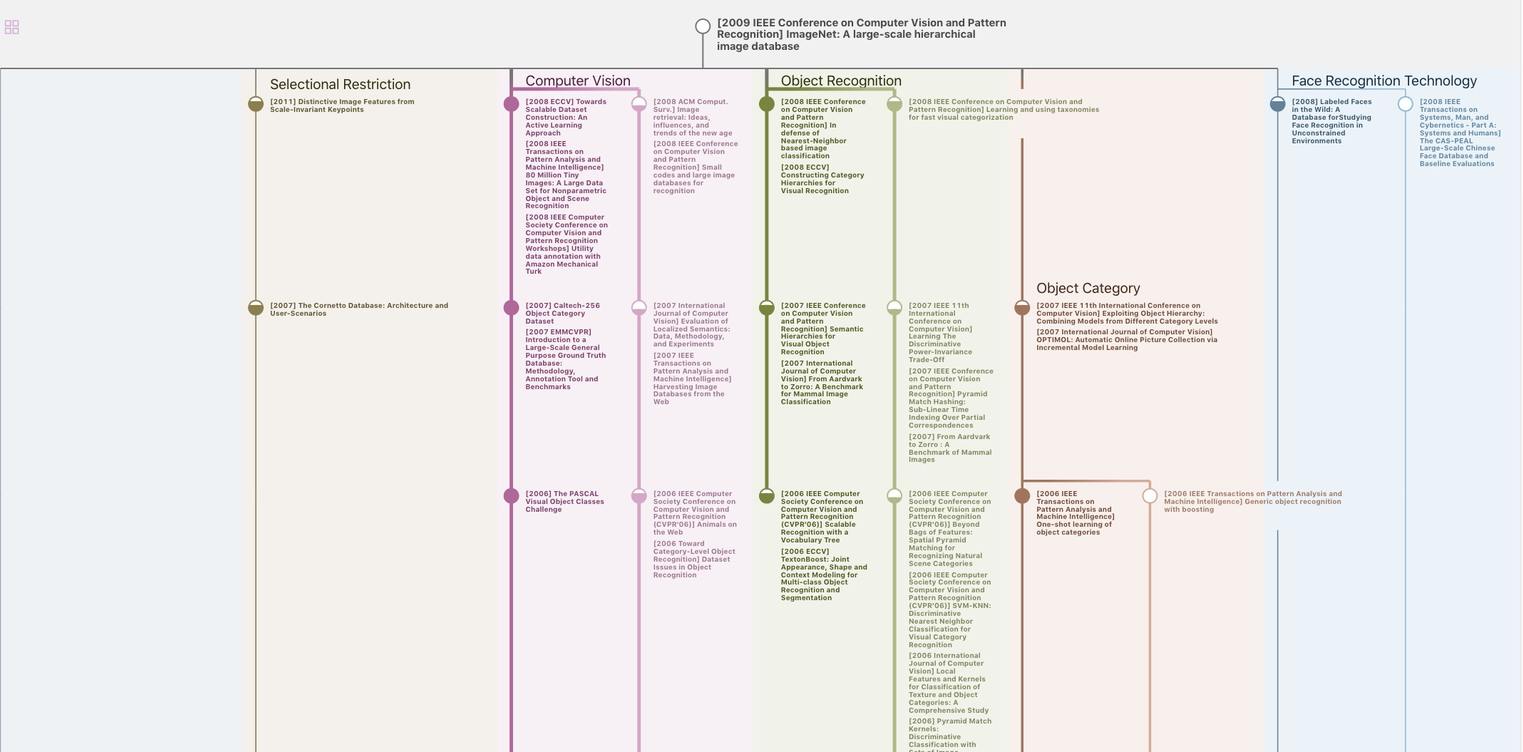
生成溯源树,研究论文发展脉络
Chat Paper
正在生成论文摘要