Information Plane Analysis for Dropout Neural Networks
ICLR 2023(2023)
摘要
The information theoretic framework promises to explain the predictive power of neural networks. In particular, the information plane analysis, which measures mutual information (MI) between input and representation as well as representation and output, should give rich insights into the training process. This approach, however, was shown to strongly depend on the choice of estimator of the MI: measuring discrete MI does not capture the nature of deterministic neural networks and continuous data distributions, and different approaches for discretization arbitrarily change results. On the other hand, measuring continuous MI for a deterministic network is not mathematically meaningful. In this work we show how the stochasticity induced by dropout layers can be utilized to estimate MI in a theoretically sound manner. We demonstrate in a range of experiments that this approach enables a meaningful information plane analysis for the large class of dropout neural networks that is widely used in practice.
更多查看译文
关键词
information plane,deep learning,mutual information,dropout,continuous distributions
AI 理解论文
溯源树
样例
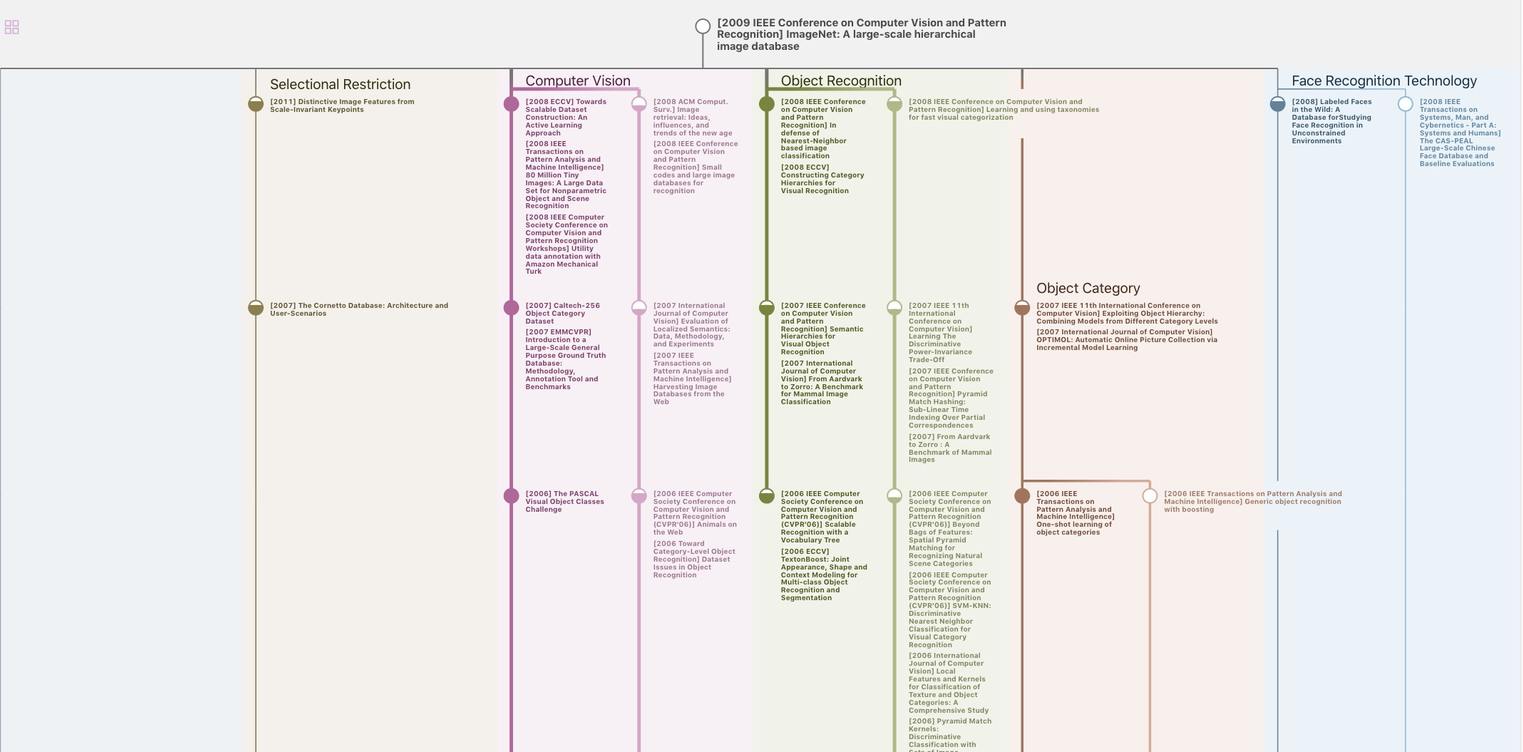
生成溯源树,研究论文发展脉络
Chat Paper
正在生成论文摘要