GReTo: Remedying dynamic graph topology-task discordance via target homophily
ICLR 2023(2023)
Abstract
Dynamic graphs are ubiquitous across disciplines where observations usually change over time. Regressions on dynamic graphs often contribute to diverse critical tasks, such as climate early-warning and traffic controlling. Existing homophily Graph Neural Networks (GNNs) adopt physical connections or feature similarity as adjacent matrix to perform node-level aggregations. However, on dynamic graphs with diverse node-wise relations, exploiting a pre-defined fixed topology for message passing inevitably leads to the aggregations of target-deviated neighbors. We designate such phenomenon as the topology-task discordance, which naturally challenges the homophily assumption. In this work, we revisit node-wise relationships and explore novel homophily measurements on dynamic graphs with both signs and distances, capturing multiple node-level spatial relations and temporal evolutions. We discover that advancing homophily aggregations to signed target-oriented message passing can effectively resolve abovementioned discordance and promote the aggregation capacity. Therefore, a novel GReTo is proposed, which performs signed message passing in immediate neighborhood, and exploits both local environments and target awareness to realize high-order message propagation. Empirically, our solution achieves significant improvements against best baselines, notably improving 24.79% on KnowAir and 3.60% on Metr-LA.
MoreTranslated text
Key words
Dynamic graph,graph homophily theory,Graph Neural Network,topology-task discordance
AI Read Science
Must-Reading Tree
Example
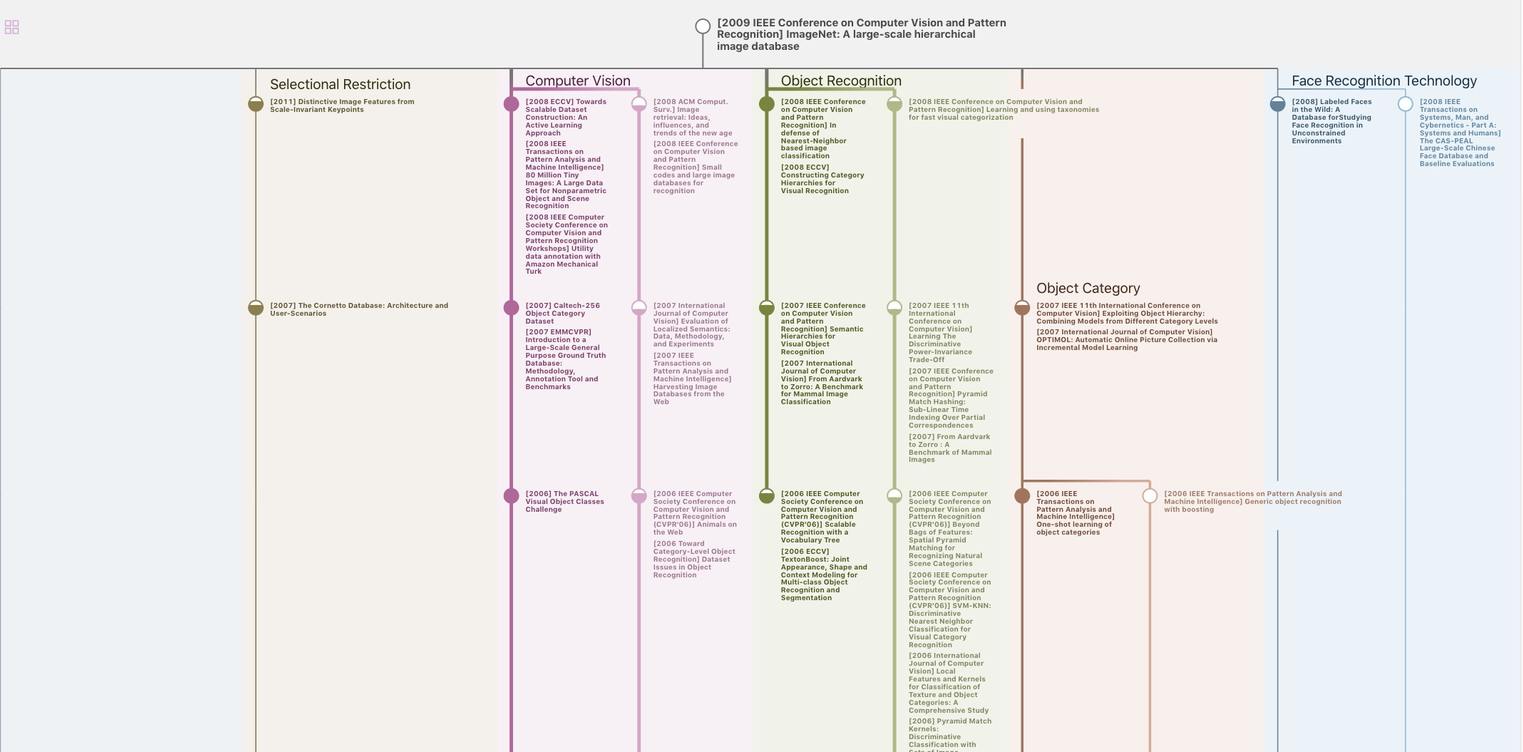
Generate MRT to find the research sequence of this paper
Chat Paper
Summary is being generated by the instructions you defined