Q-Match: Self-Supervised Learning by Matching Distributions Induced by a Queue
CoRR(2023)
摘要
In semi-supervised learning, student-teacher distribution matching has been successful in improving performance of models using unlabeled data in conjunction with few labeled samples. In this paper, we aim to replicate that success in the self-supervised setup where we do not have access to any labeled data during pre-training. We introduce our algorithm, Q-Match, and show it is possible to induce the student-teacher distributions without any knowledge of downstream classes by using a queue of embeddings of samples from the unlabeled dataset. We focus our study on tabular datasets and show that Q-Match outperforms previous self-supervised learning techniques when measuring downstream classification performance. Furthermore, we show that our method is sample efficient--in terms of both the labels required for downstream training and the amount of unlabeled data required for pre-training--and scales well to the sizes of both the labeled and unlabeled data.
更多查看译文
关键词
matching distributions,learning,q-match,self-supervised
AI 理解论文
溯源树
样例
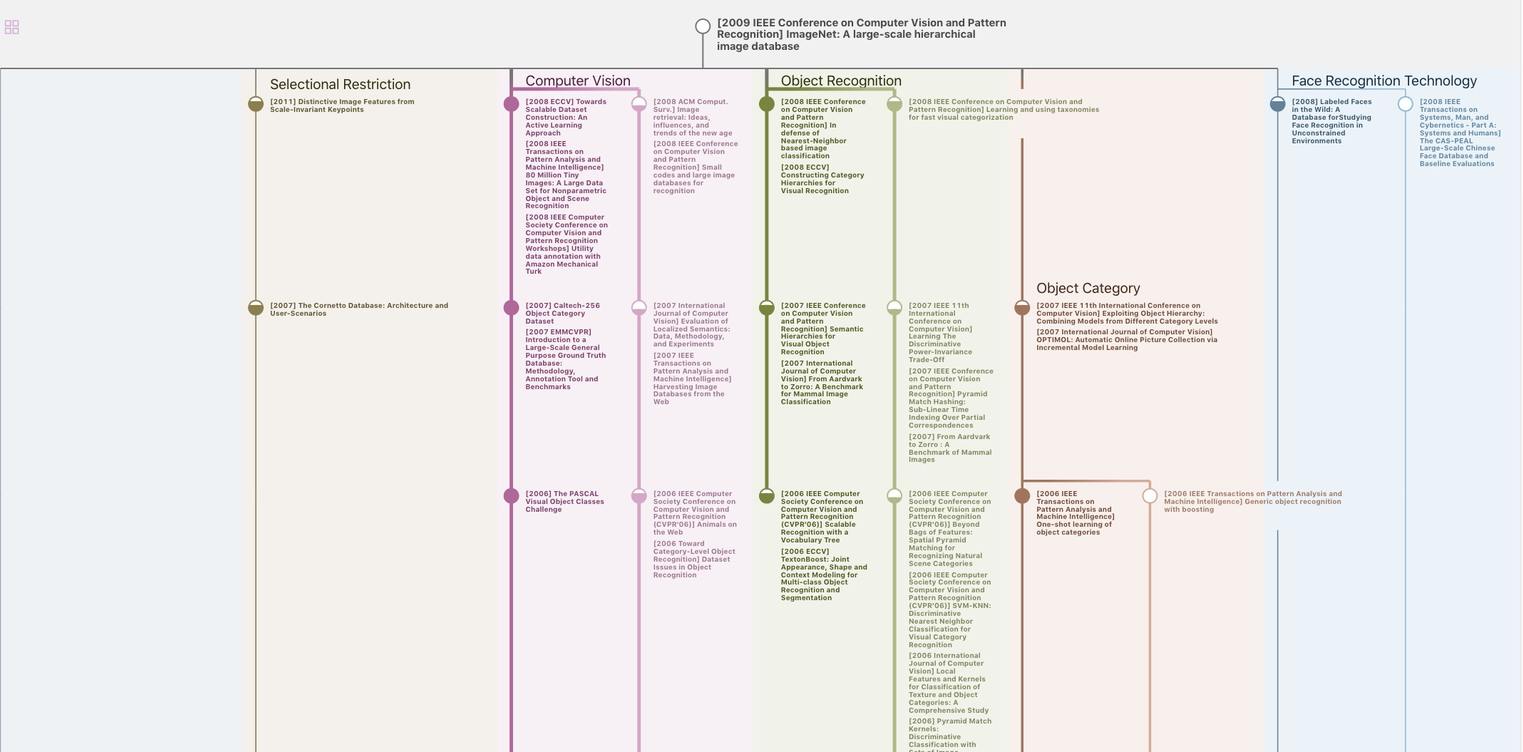
生成溯源树,研究论文发展脉络
Chat Paper
正在生成论文摘要