Dynamic Named Entity Recognition.
ACM Symposium on Applied Computing(2023)
摘要
Named Entity Recognition (NER) is a challenging and widely studied task that involves detecting and typing entities in text. So far, NER still approaches entity typing as a task of classification into universal classes (e.g. date, person, or location). Recent advances in natural language processing focus on architectures of increasing complexity that may lead to overfitting and memorization, and thus, underuse of context. Our work targets situations where the type of entities depends on the context and cannot be solved solely by memorization. We hence introduce a new task: Dynamic Named Entity Recognition (DNER), providing a framework to better evaluate the ability of algorithms to extract entities by exploiting the context. The DNER benchmark is based on two datasets, DNER-RotoWire and DNER-IMDb, respectively aiming at detecting winning/losing players in different basketball match summaries and predicting actors' credit orders within different movie synopsis. We evaluate baseline models and present experiments reflecting issues and research axes related to this novel task.
更多查看译文
关键词
Information extraction,NER,contextualization,datasets
AI 理解论文
溯源树
样例
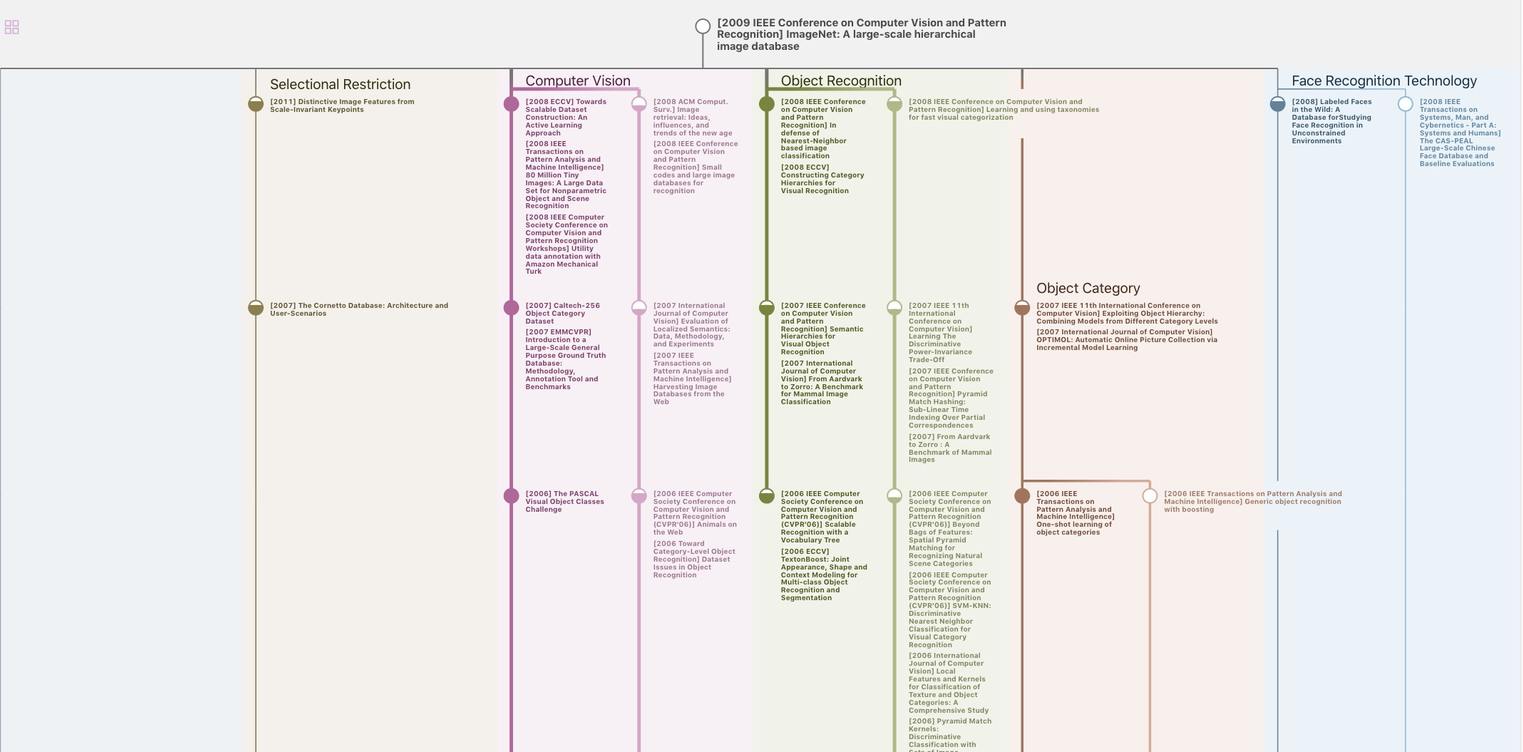
生成溯源树,研究论文发展脉络
Chat Paper
正在生成论文摘要