PaGE-Link: Path-based Graph Neural Network Explanation for Heterogeneous Link Prediction
WWW 2023(2023)
摘要
Transparency and accountability have become major concerns for black-box machine learning (ML) models. Proper explanations for the model behavior increase model transparency and help researchers develop more accountable models. Graph neural networks (GNN) have recently shown superior performance in many graph ML problems than traditional methods, and explaining them has attracted increased interest. However, GNN explanation for link prediction (LP) is lacking in the literature. LP is an essential GNN task and corresponds to web applications like recommendation and sponsored search on web. Given existing GNN explanation methods only address node/graph-level tasks, we propose Path-based GNN Explanation for heterogeneous Link prediction (PaGE-Link) that generates explanations with connection interpretability, enjoys model scalability, and handles graph heterogeneity. Qualitatively, PaGE-Link can generate explanations as paths connecting a node pair, which naturally captures connections between the two nodes and easily transfer to human-interpretable explanations. Quantitatively, explanations generated by PaGE-Link improve AUC for recommendation on citation and user-item graphs by 9 - 35% and are chosen as better by 78.79% of responses in human evaluation.
更多查看译文
关键词
graph neural network explanation,heterogeneous
AI 理解论文
溯源树
样例
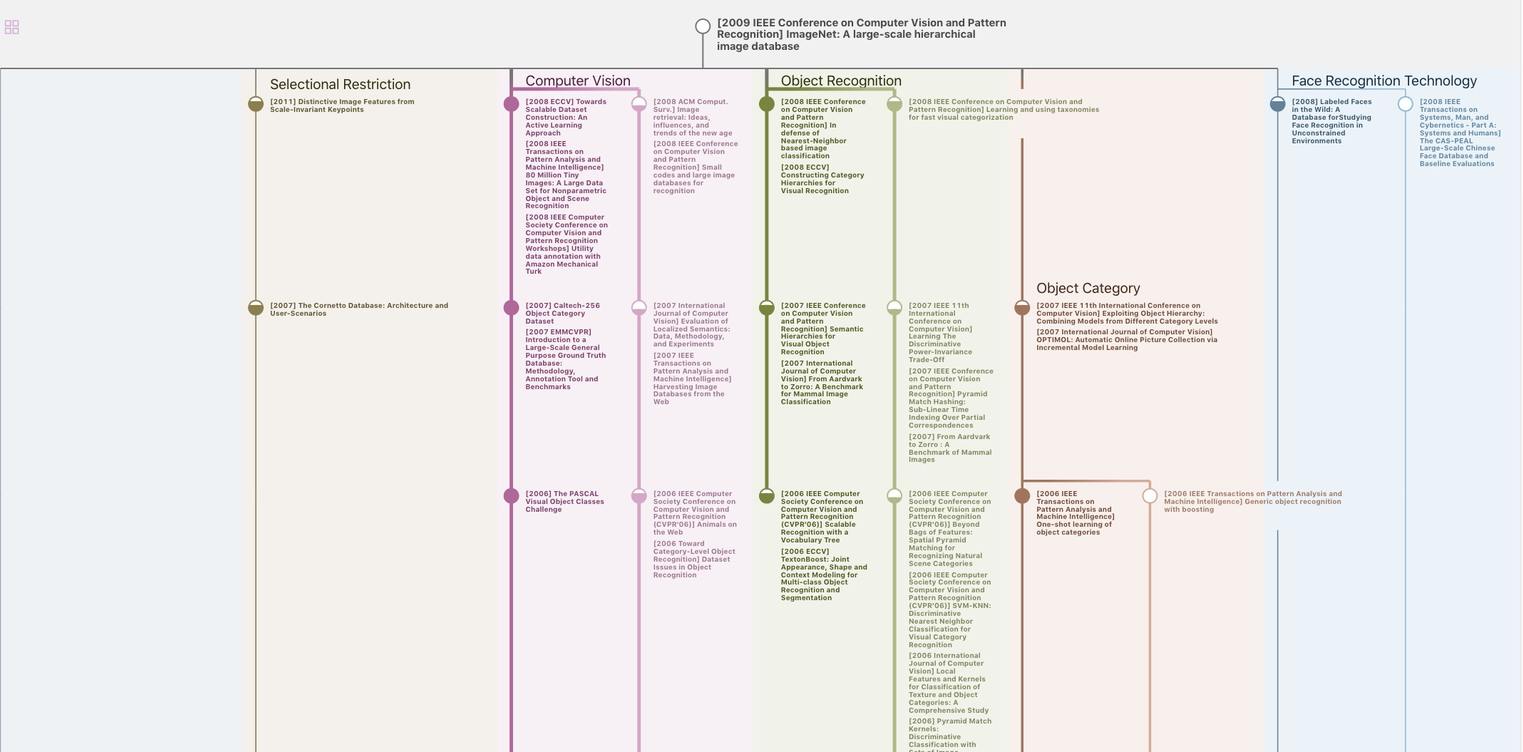
生成溯源树,研究论文发展脉络
Chat Paper
正在生成论文摘要