Towards Interpretable Federated Learning
CoRR(2023)
摘要
Federated learning (FL) enables multiple data owners to build machine learning models collaboratively without exposing their private local data. In order for FL to achieve widespread adoption, it is important to balance the need for performance, privacy-preservation and interpretability, especially in mission critical applications such as finance and healthcare. Thus, interpretable federated learning (IFL) has become an emerging topic of research attracting significant interest from the academia and the industry alike. Its interdisciplinary nature can be challenging for new researchers to pick up. In this paper, we bridge this gap by providing (to the best of our knowledge) the first survey on IFL. We propose a unique IFL taxonomy which covers relevant works enabling FL models to explain the prediction results, support model debugging, and provide insights into the contributions made by individual data owners or data samples, which in turn, is crucial for allocating rewards fairly to motivate active and reliable participation in FL. We conduct comprehensive analysis of the representative IFL approaches, the commonly adopted performance evaluation metrics, and promising directions towards building versatile IFL techniques.
更多查看译文
关键词
learning
AI 理解论文
溯源树
样例
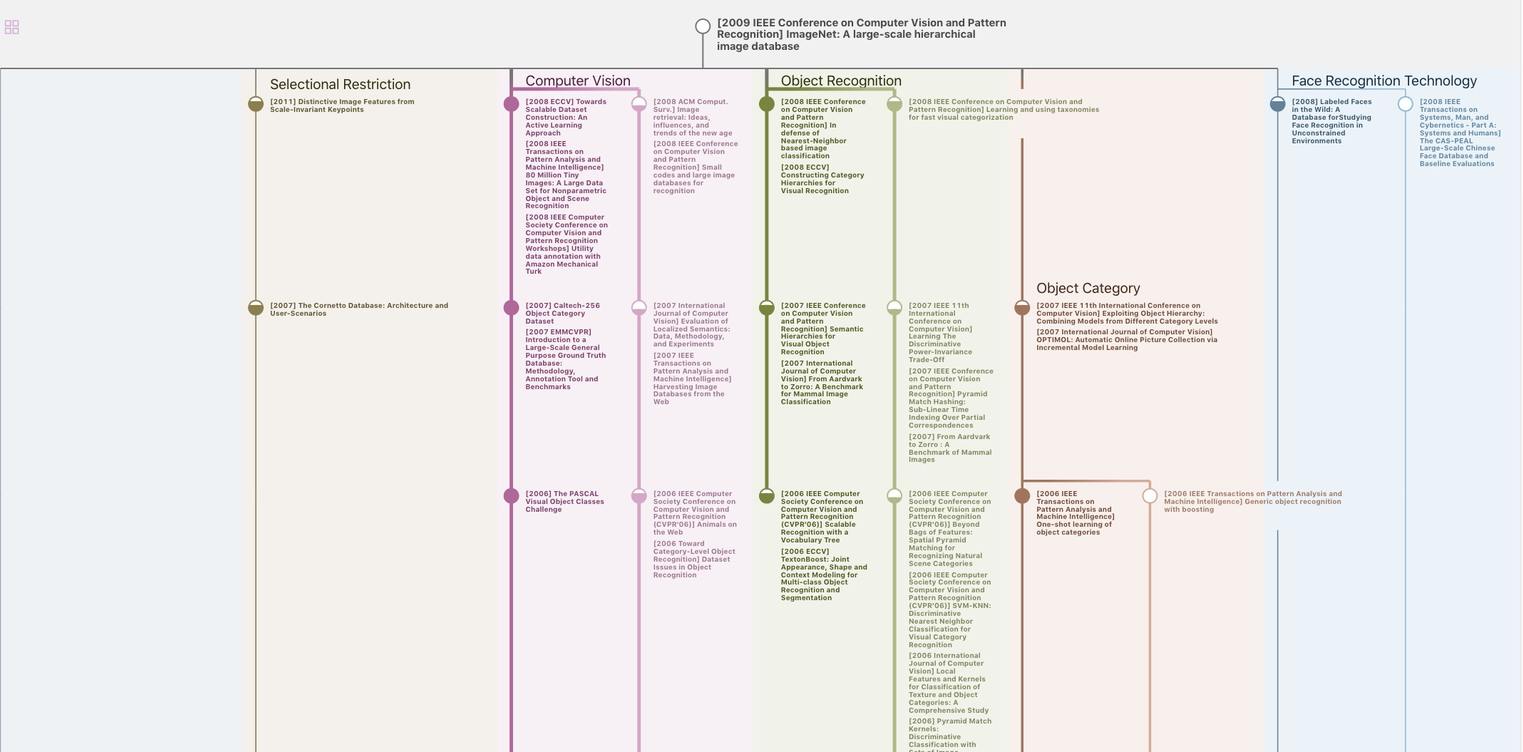
生成溯源树,研究论文发展脉络
Chat Paper
正在生成论文摘要