REASONER: An Explainable Recommendation Dataset with Multi-aspect Real User Labeled Ground Truths Towards more Measurable Explainable Recommendation
arxiv(2023)
摘要
Explainable recommendation has attracted much attention from the industry and academic communities. It has shown great potential for improving the recommendation persuasiveness, informativeness and user satisfaction. Despite a lot of promising explainable recommender models have been proposed in the past few years, the evaluation strategies of these models suffer from several limitations. For example, the explanation ground truths are not labeled by real users, the explanations are mostly evaluated based on only one aspect and the evaluation strategies can be hard to unify. To alleviate the above problems, we propose to build an explainable recommendation dataset with multi-aspect real user labeled ground truths. In specific, we firstly develop a video recommendation platform, where a series of questions around the recommendation explainability are carefully designed. Then, we recruit about 3000 users with different backgrounds to use the system, and collect their behaviors and feedback to our questions. In this paper, we detail the construction process of our dataset and also provide extensive analysis on its characteristics. In addition, we develop a library, where ten well-known explainable recommender models are implemented in a unified framework. Based on this library, we build several benchmarks for different explainable recommendation tasks. At last, we present many new opportunities brought by our dataset, which are expected to shed some new lights to the explainable recommendation field. Our dataset, library and the related documents have been released at https://reasoner2023.github.io/.
更多查看译文
关键词
explainable recommendation dataset,measurable explainable recommendation,user labeled ground truths,multi-aspect
AI 理解论文
溯源树
样例
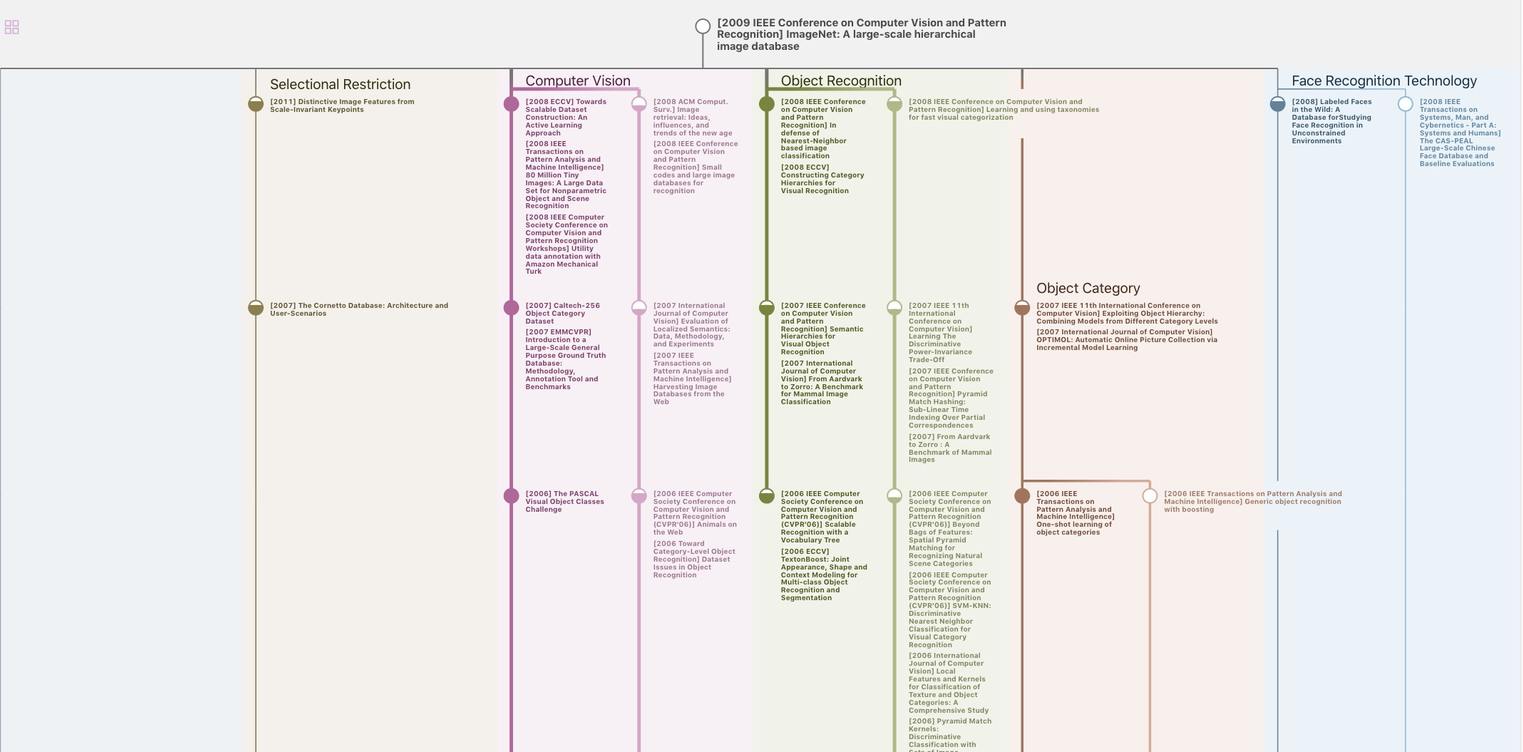
生成溯源树,研究论文发展脉络
Chat Paper
正在生成论文摘要