Transformer-Based Domain-Specific Representation for Unsupervised Domain Adaptive Vehicle Re-Identification
IEEE Transactions on Intelligent Transportation Systems(2023)
摘要
Fully-supervised vehicle re-identification (re-ID) methods are faced with performance degradation when applied to new image domains. Therefore, developing unsupervised domain adaptation (UDA) to transfer the knowledge from learned source domain to new unlabeled target domain becomes an indispensable task. It is challenging because different domains have various image appearances, such as different backgrounds, illuminations and resolutions, especially when cameras have different viewpoints. To tackle this domain gap issue, a novel Transformer-based Domain-Specific Representation learning network (TDSR) is proposed to dynamically focus on corresponding detailed hints for each domain. Specifically, with the source and target domain being trained simultaneously, a domain encoding module is proposed to introduce domain information into the network. The original features of source and target domains are enriched with these domain encodings first, and then sequentially processed by a Transformer encoder to model contextual information and a decoder to summarize the encoded features into the final domain-specific feature representations. Moreover, we propose a Contrastive Clustering Loss (CCL) to directly optimize the distribution of features at cluster level. Instances are overall pulled closer to the prototype of the same identity, and pushed farther from the prototypes of different identities. It helps compact the clusters in the latent space and improve the discriminative capability of the network, leading to more accurate pseudo-label assignment in TDSR. Our method outperforms the state-of-the-art UDA methods on vehicle re-ID benchmark datasets VeRi and VehicleID on both real-world to real-world and synthetic to real-world settings.
更多查看译文
关键词
Unsupervised domain adaption,vehicle re-identification,transformer,domain encodings,clustering
AI 理解论文
溯源树
样例
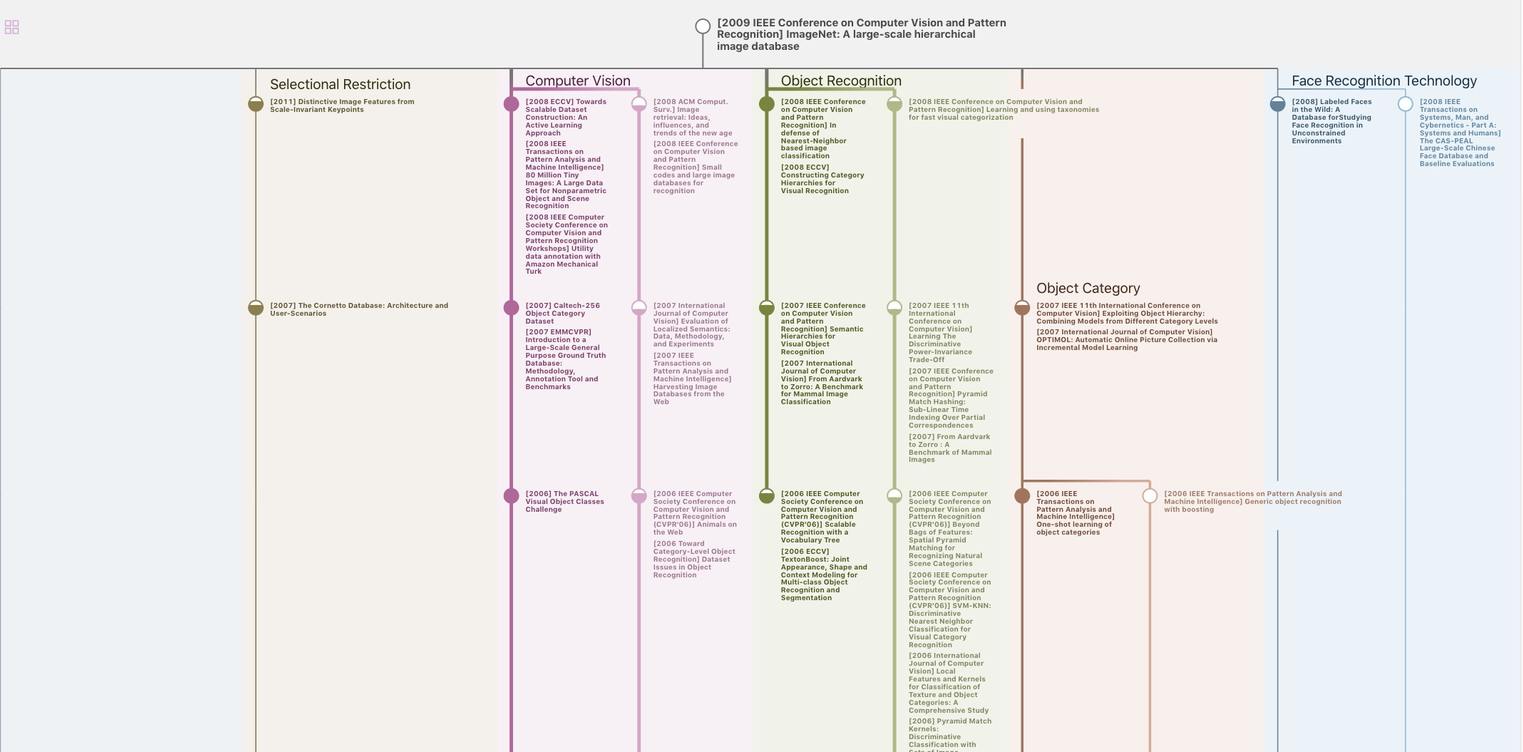
生成溯源树,研究论文发展脉络
Chat Paper
正在生成论文摘要