Understanding plasticity in neural networks
arxiv(2023)
摘要
Plasticity, the ability of a neural network to quickly change its predictions in response to new information, is essential for the adaptability and robustness of deep reinforcement learning systems. Deep neural networks are known to lose plasticity over the course of training even in relatively simple learning problems, but the mechanisms driving this phenomenon are still poorly understood. This paper conducts a systematic empirical analysis into plasticity loss, with the goal of understanding the phenomenon mechanistically in order to guide the future development of targeted solutions. We find that loss of plasticity is deeply connected to changes in the curvature of the loss landscape, but that it typically occurs in the absence of saturated units or divergent gradient norms. Based on this insight, we identify a number of parameterization and optimization design choices which enable networks to better preserve plasticity over the course of training. We validate the utility of these findings in larger-scale learning problems by applying the best-performing intervention, layer normalization, to a deep RL agent trained on the Arcade Learning Environment.
更多查看译文
关键词
plasticity,neural networks,understanding
AI 理解论文
溯源树
样例
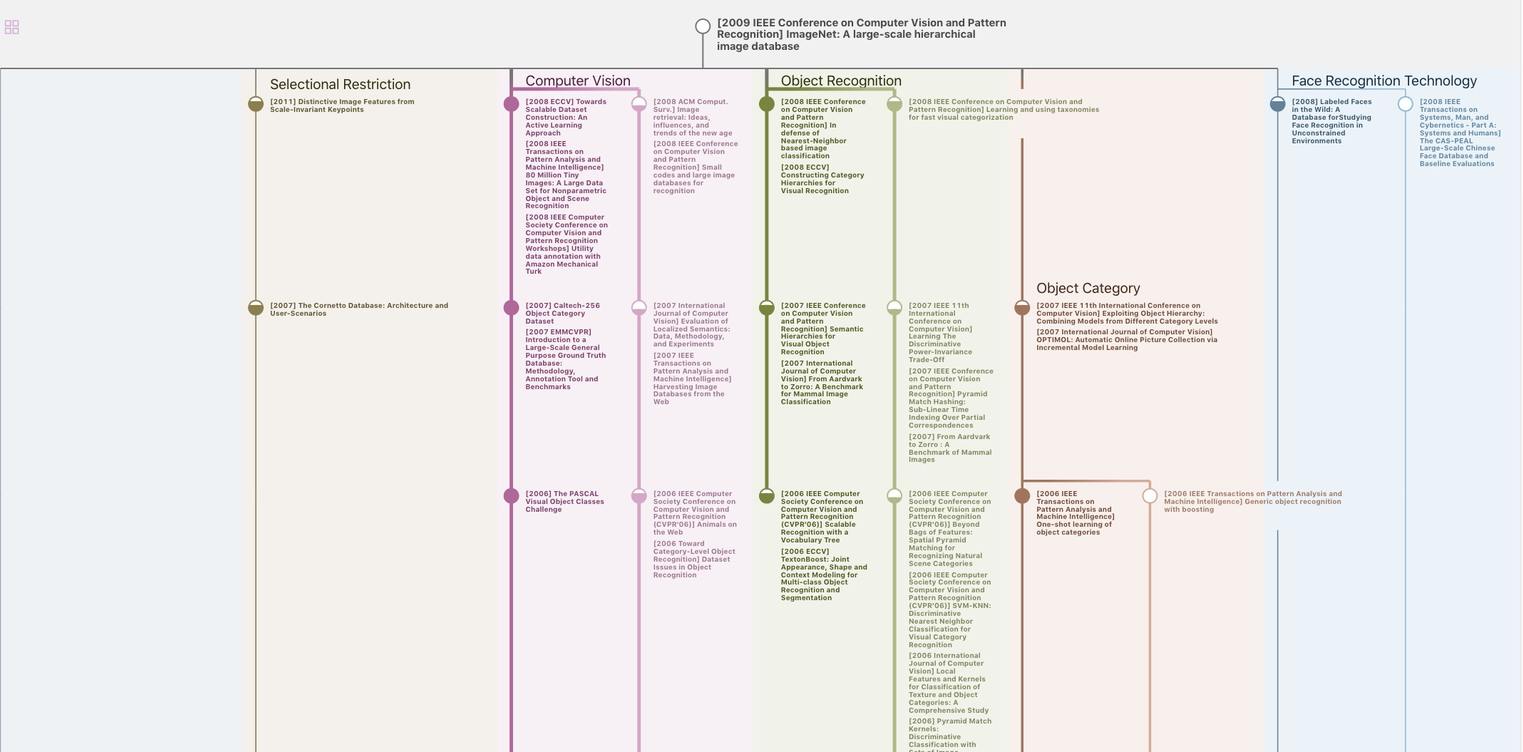
生成溯源树,研究论文发展脉络
Chat Paper
正在生成论文摘要