Structured State Space Models for In-Context Reinforcement Learning
NeurIPS(2023)
摘要
Structured state space sequence (S4) models have recently achieved state-of-the-art performance on long-range sequence modeling tasks. These models also have fast inference speeds and parallelisable training, making them potentially useful in many reinforcement learning settings. We propose a modification to a variant of S4 that enables us to initialise and reset the hidden state in parallel, allowing us to tackle reinforcement learning tasks. We show that our modified architecture runs asymptotically faster than Transformers and performs better than LSTM models on a simple memory-based task. Then, by leveraging the model's ability to handle long-range sequences, we achieve strong performance on a challenging meta-learning task in which the agent is given a randomly-sampled continuous control environment, combined with a randomly-sampled linear projection of the environment's observations and actions. Furthermore, we show the resulting model can adapt to out-of-distribution held-out tasks. Overall, the results presented in this paper suggest that the S4 models are a strong contender for the default architecture used for in-context reinforcement learning
更多查看译文
关键词
structured state space models,reinforcement,learning,in-context
AI 理解论文
溯源树
样例
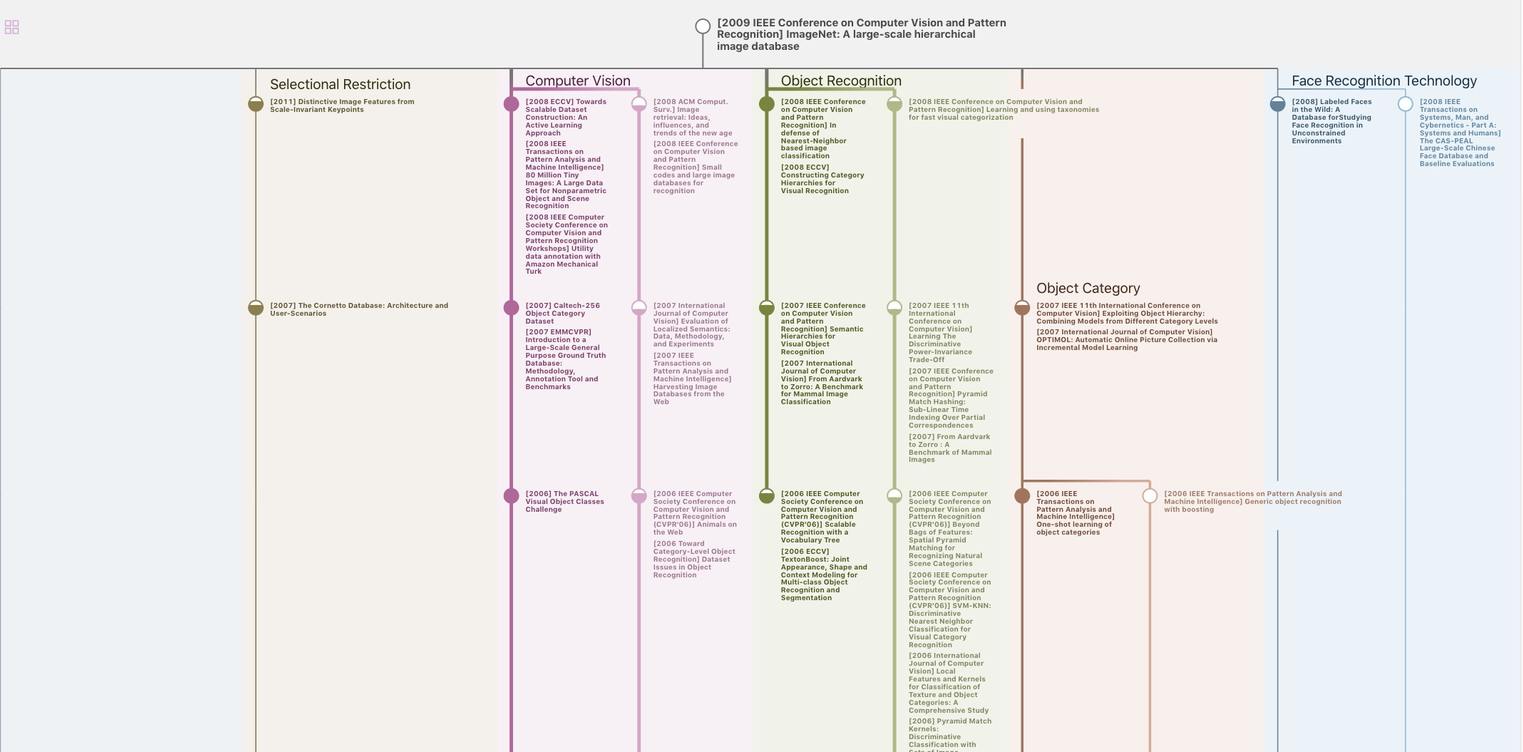
生成溯源树,研究论文发展脉络
Chat Paper
正在生成论文摘要