Social Determinants of COVID-19 Vaccination Rates: A Time-Constrained Multiple Mediation Analysis.
Cureus(2023)
摘要
Objective To estimate the multiple direct/indirect effects of social, environmental, and economic factors on COVID-19 vaccination rates (series complete) in the 3109 continental counties in the United States (U.S.). Study design The dependent variable was the COVID-19 vaccination rates in the U.S. (April 15, 2022). Independent variables were collected from reliable secondary data sources, including the Census and CDC. Independent variables measured at two different time frames were utilized to predict vaccination rates. The number of vaccination sites in a given county was calculated using the geographic information system (GIS) packages as of April 9, 2022. The Internet Archive (Way Back Machine) was used to look up data for historical dates. Methods A chain of temporally-constrained least absolute shrinkage and selection operator (LASSO) regressions was used to identify direct and indirect effects on vaccination rates. The first regression identified direct predictors of vaccination rates. Next, the direct predictors were set as response variables in subsequent regressions and regressed on variables that occurred before them. These regressions identified additional indirect predictors of vaccination. Finally, both direct and indirect variables were included in a network model. Results Fifteen variables directly predicted vaccination rates and explained 43% of the variation in vaccination rates in April 2022. In addition, 11 variables indirectly affected vaccination rates, and their influence on vaccination was mediated by direct factors. For example, children in poverty rate mediated the effect of (a) median household income, (b) children in single-parent homes, and (c) income inequality. For another example, median household income mediated the effect of (a) the percentage of residents under the age of 18, (b) the percentage of residents who are Asian, (c) home ownership, and (d) traffic volume in the prior year. Our findings describe not only the direct but also the indirect effect of variables. Conclusions A diverse set of demographics, social determinants, public health status, and provider characteristics predicted vaccination rates. Vaccination rates change systematically and are affected by the demographic composition and social determinants of illness within the county. One of the merits of our study is that it shows how the direct predictors of vaccination rates could be mediators of the effects of other variables.
更多查看译文
关键词
a chain of lasso,covid-19 vaccination rates,data-driven approach,multiple mediation analysis,social/environmental determinants
AI 理解论文
溯源树
样例
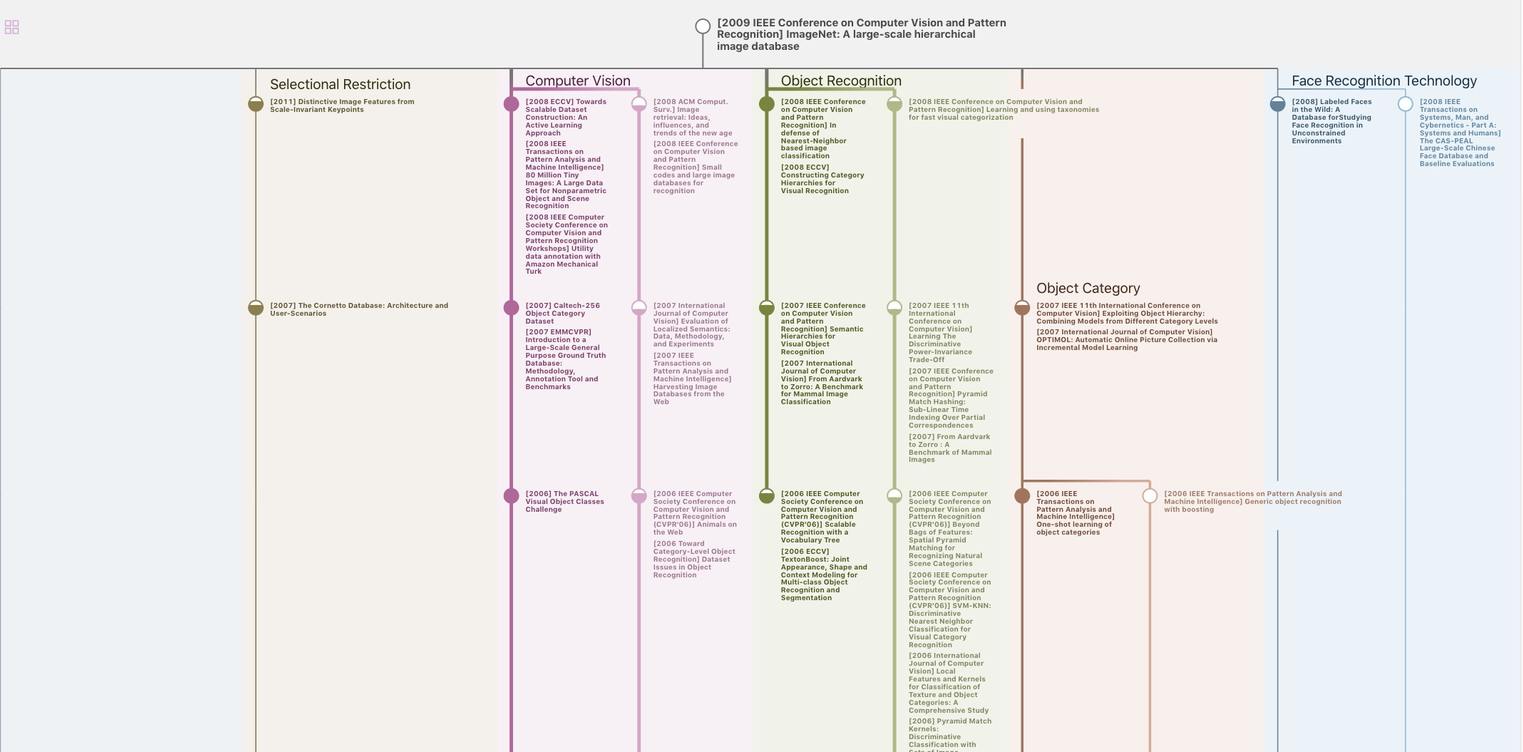
生成溯源树,研究论文发展脉络
Chat Paper
正在生成论文摘要