Online Learning for Equilibrium Pricing in Markets under Incomplete Information
2023 62ND IEEE CONFERENCE ON DECISION AND CONTROL, CDC(2023)
摘要
The study of market equilibria is central to economic theory, particularly in efficiently allocating scarce resources. However, the computation of equilibrium prices at which the supply of goods matches their demand typically relies on having access to complete information on private attributes of agents, e.g., suppliers' cost functions, which are often unavailable in practice. Motivated by this practical consideration, we consider the problem of setting equilibrium prices in the incomplete information setting wherein a market operator seeks to satisfy the customer demand for a commodity by purchasing the required amount from competing suppliers with privately known cost functions unknown to the market operator. In this incomplete information setting, we consider the online learning problem of learning equilibrium prices over time while jointly optimizing three performance metrics-unmet demand, cost regret, and payment regret-pertinent in the context of equilibrium pricing over a horizon of T periods. In the general setting when suppliers' cost functions are time-varying, we show that no online algorithm can achieve sublinear regret on all three metrics. Thus, we consider the setting when suppliers' cost functions are fixed and develop algorithms that achieve a regret of (i) O(log log T) when the customer demand is constant over time and (ii) O(root T log log T) when the demand is variable over time.
更多查看译文
AI 理解论文
溯源树
样例
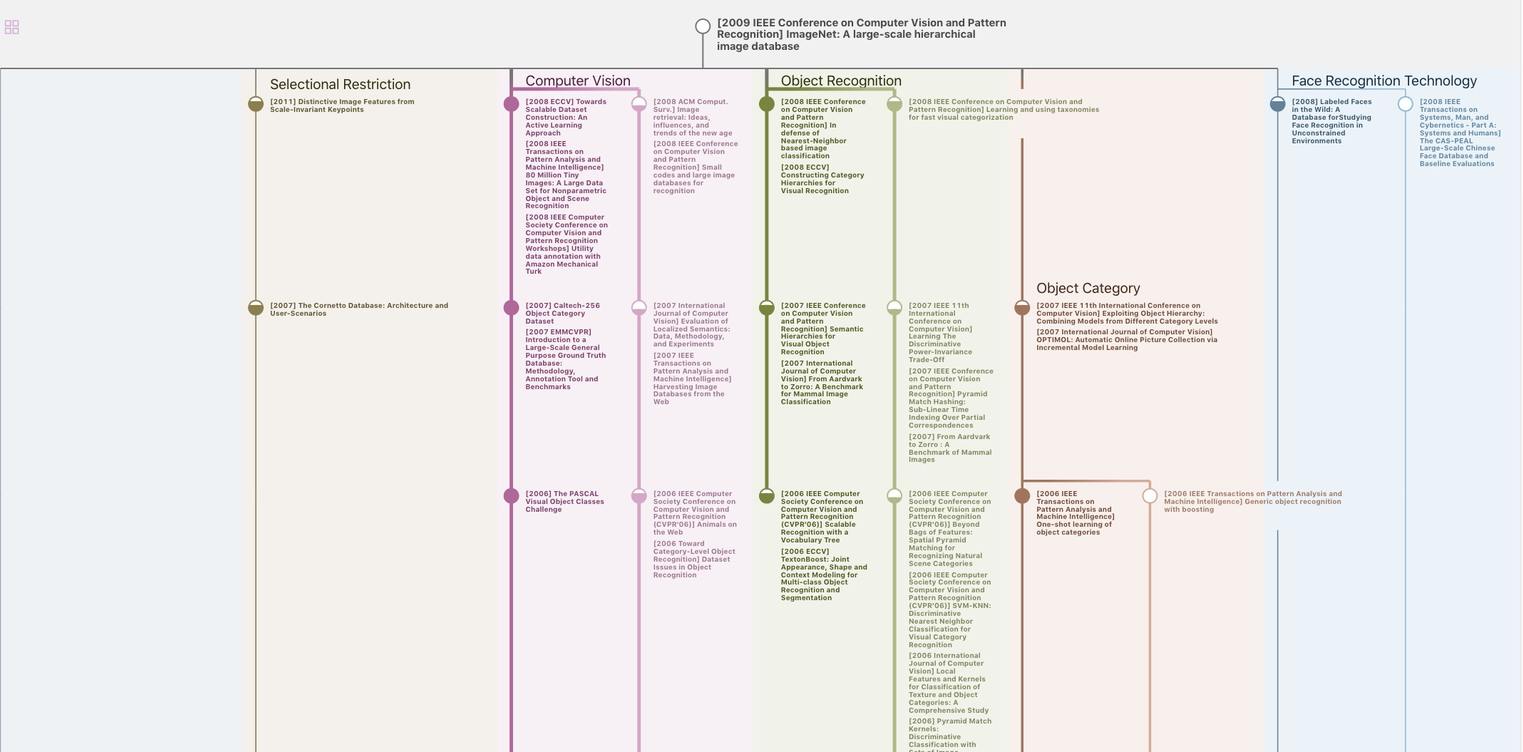
生成溯源树,研究论文发展脉络
Chat Paper
正在生成论文摘要