Masked Image Training for Generalizable Deep Image Denoising
CVPR 2023(2023)
摘要
When capturing and storing images, devices inevitably introduce noise. Reducing this noise is a critical task called image denoising. Deep learning has become the de facto method for image denoising, especially with the emergence of Transformer-based models that have achieved notable state-of-the-art results on various image tasks. However, deep learning-based methods often suffer from a lack of generalization ability. For example, deep models trained on Gaussian noise may perform poorly when tested on other noise distributions. To address this issue, we present a novel approach to enhance the generalization performance of denoising networks, known as masked training. Our method involves masking random pixels of the input image and reconstructing the missing information during training. We also mask out the features in the self-attention layers to avoid the impact of training-testing inconsistency. Our approach exhibits better generalization ability than other deep learning models and is directly applicable to real-world scenarios. Additionally, our interpretability analysis demonstrates the superiority of our method.
更多查看译文
关键词
Low-level vision
AI 理解论文
溯源树
样例
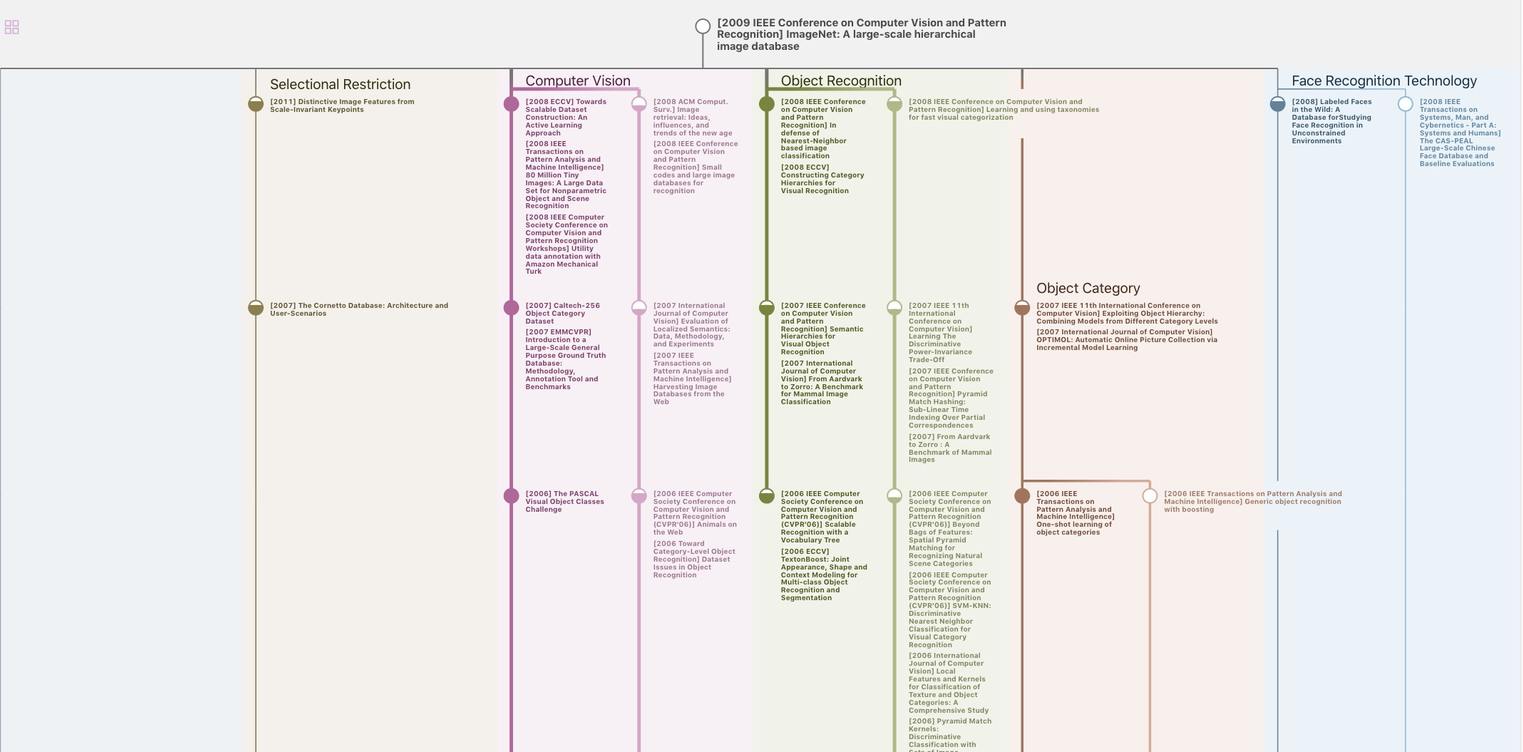
生成溯源树,研究论文发展脉络
Chat Paper
正在生成论文摘要